集成学习介绍:
强力的集成学习算法主要有2种:基于Bagging的算法和基于Boosting的算法,基于Bagging的代表算法有随机森林,而基于Boosting的代表算法则有Adaboost、GBDT、XGBOOST。
集成学习的思想同样适用于深度学习,集成应用于深度学习时,组合若干网络的预测以得到一个最终的预测。通常,使用多个不同架构的神经网络得到的性能会更好,因为不同架构的网络一般会在不同的训练样本上犯错,因而集成学习带来的收益会更大。即使是使用同一个模型训练的结果也会有意想不到的惊喜。
1. 快照集成策略
在训练同一个网络的过程中保存了不同的权值快照,然后在训练之后创建了同一架构、不同权值的集成网络。这么做可以提升测试的表现,同时也超省事,因为你只需要训练一个模型、训练一次就好,只要记得随时保存权值就行。
快照集成推荐使用热重启+余弦退火学习率方式(又被叫做热重启随机梯度下降),这种循环学习率几乎为快照集成量身打造,利用热重启随机梯度下降法的特点,每次收敛到局部极值点的时候就可以缓存一个权重快照,缓存那么几个就可以做集成学习了。
2. 随机权重平均
和上面的快照集成策略需要让同一个样本通过几个结构相同但是不同参数网络,然后集成不同。随机权重平均通过组合同一网络在训练的不同阶段的权值得到一个集成,接着使用组合后的权值做出预测。这样只需要进行一次预测,并且预测的结果要优于目前最先进的快照集成。
随机权重平均(Stochastic Weight Averaging):这是常用的一种平均算法,实现还是比较简单的,每次迭代更新权重,保证每个权重对于最终结果的影响因子是一致的。
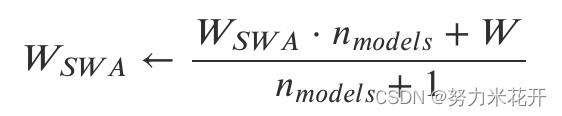
SWA是一种不需要额外训练,不需要额外增加推理时间,但是非常有效的一种深度学习集成策略。很推荐在模型训练的时候使用。
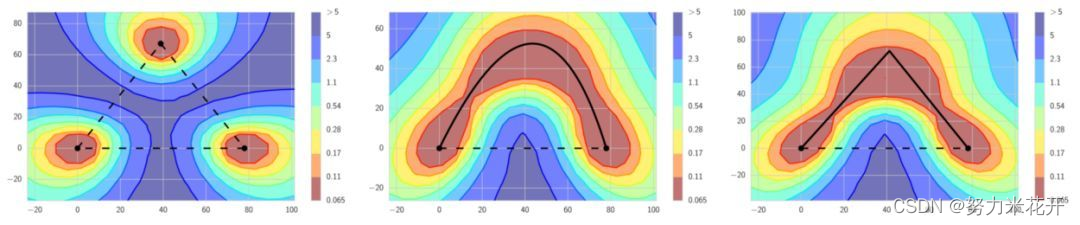
torch中集成了SWA算法,具体介绍一下初始化的参数
#代码位置torchcontrib.optim.swa
class SWA(Optimizer):
def __init__(self, optimizer, swa_start=None, swa_freq=None, swa_lr=None)
#参数含义,swa有两种格式,一种是自动模式是一种是手动模式。在自动模式中,SWA按照频率swa_fre计算计算模型平均值
# swa_start表示在多少iteration之后开始计算模型的平均值,这是SWA第一次开始计算的iteration
# 如果用户配置了swa_lr参数,那么从swa_start之后的iter中,优化器的学习率将会变成swa_lr
# 如果需要设置成自动模型,那么swa_start和swa_freq是必须提供的两个参数。
# 如果需要设置成手动模型,俺么需要使用该类的update_swa()或者update_swa_group()方法来主动更新swa参数
# 在模型训练的最后阶段,需要使用方法swap_swa_sgd方法,这个方法将swa保存的平均模型参数赋值到模型的params.data中。
# 如果模型中涉及到BN层,那么需要在训练的最后阶段更新BN的running mean和running var.也可以使用方法torchcontrib.optim.swa.bn_update实现
# bn_update方法会全量的遍历训练数据,从头开始统计网络每个BN层的running mean和running var。因此当训练数据较多的时候,训练速度比较慢。
SWA可以结合不同的优化器算法一起实用,这里给出一个和SGD一起使用的一个例子
from torchcontrib.optim.swa import SWA
# training loop
base_opt = torch.optim.SGD(model.parameters(), lr=0.1)
opt = torchcontrib.optim.SWA(base_opt, swa_start=10, swa_freq=5, swa_lr=0.05)
for _ in range(100):
opt.zero_grad()
loss_fn(model(input), target).backward()
opt.step()
opt.swap_swa_sgd()
opt.bn_update(train_loader, model)
#执行到这里之后,直接保存model即可,这时候保存的已经是通过swa权值平均的模型参数了。
torch.save({'state_dict':model.state_dict()},'final.pth.tar')
如果想要和周期性学习率一起使用SWA,那么可以这么写。需要注意的细节有:
- 在训练的时候,必须要先调用基础优化器的step方法,然后再调用周期性学习率的step方法,顺序不能反
- 不要调用swa算法的step方法,不然会重复反向梯度传播,浪费时间。
from torchcontrib.optim.swa import SWA
# training loop
model = Unet(classes=2)
dataloader = pass#定义自己的dataloader
num_epoch = 10
base_opt = torch.optim.SGD(model.parameters(), lr=0.1)
scheduler = torch.optim.lr_scheduler.CyclicLR(base_opt, base_lr=1e-4, max_lr=args.lr, step_size_up=2000,
mode="triangular")
swa = torchcontrib.optim.SWA(base_opt)#采用手动swa
step = 0
for epoch in range(num_epochs):
model.train())
for i, (data, label) in enumerate(dataloader):
base_opt.zero_grad()
loss_fn(model(input), target).backward()
base_opt.step()
scheduler.step()
step +=1
if step%4000==0:
#一般swa的更新设置为周期学习率的一个周期
swa.update_swa()
opt.swap_swa_sgd()
opt.bn_update(train_loader, model)
#执行到这里之后,直接保存model即可,这时候保存的已经是通过swa权值平均的模型参数了。
torch.save({'state_dict':model.state_dict()},'final.pth.tar')
具体的SWA算法如下,看具体的方法实现可以帮助更加熟悉SWA的实现原理:
from collections import defaultdict
from itertools import chain
from torch.optim import Optimizer
import torch
import warnings
class SWA(Optimizer):
def __init__(self, optimizer, swa_start=None, swa_freq=None, swa_lr=None):
r"""Implements Stochastic Weight Averaging (SWA).
Stochastic Weight Averaging was proposed in `Averaging Weights Leads to
Wider Optima and Better Generalization`_ by Pavel Izmailov, Dmitrii
Podoprikhin, Timur Garipov, Dmitry Vetrov and Andrew Gordon Wilson
(UAI 2018).
SWA is implemented as a wrapper class taking optimizer instance as input
and applying SWA on top of that optimizer.
SWA can be used in two modes: automatic and manual. In the automatic
mode SWA running averages are automatically updated every
:attr:`swa_freq` steps after :attr:`swa_start` steps of optimization. If
:attr:`swa_lr` is provided, the learning rate of the optimizer is reset
to :attr:`swa_lr` at every step starting from :attr:`swa_start`. To use
SWA in automatic mode provide values for both :attr:`swa_start` and
:attr:`swa_freq` arguments.
Alternatively, in the manual mode, use :meth:`update_swa` or
:meth:`update_swa_group` methods to update the SWA running averages.
In the end of training use `swap_swa_sgd` method to set the optimized
variables to the computed averages.
Args:
optimizer (torch.optim.Optimizer): optimizer to use with SWA
swa_start (int): number of steps before starting to apply SWA in
automatic mode; if None, manual mode is selected (default: None)
swa_freq (int): number of steps between subsequent updates of
SWA running averages in automatic mode; if None, manual mode is
selected (default: None)
swa_lr (float): learning rate to use starting from step swa_start
in automatic mode; if None, learning rate is not changed
(default: None)
Examples:
>>> # automatic mode
>>> base_opt = torch.optim.SGD(model.parameters(), lr=0.1)
>>> opt = torchcontrib.optim.SWA(
>>> base_opt, swa_start=10, swa_freq=5, swa_lr=0.05)
>>> for _ in range(100):
>>> opt.zero_grad()
>>> loss_fn(model(input), target).backward()
>>> opt.step()
>>> opt.swap_swa_sgd()
>>> # manual mode
>>> opt = torchcontrib.optim.SWA(base_opt)
>>> for i in range(100):
>>> opt.zero_grad()
>>> loss_fn(model(input), target).backward()
>>> opt.step()
>>> if i > 10 and i % 5 == 0:
>>> opt.update_swa()
>>> opt.swap_swa_sgd()
.. note::
SWA does not support parameter-specific values of :attr:`swa_start`,
:attr:`swa_freq` or :attr:`swa_lr`. In automatic mode SWA uses the
same :attr:`swa_start`, :attr:`swa_freq` and :attr:`swa_lr` for all
parameter groups. If needed, use manual mode with
:meth:`update_swa_group` to use different update schedules for
different parameter groups.
.. note::
Call :meth:`swap_swa_sgd` in the end of training to use the computed
running averages.
.. note::
If you are using SWA to optimize the parameters of a Neural Network
containing Batch Normalization layers, you need to update the
:attr:`running_mean` and :attr:`running_var` statistics of the
Batch Normalization module. You can do so by using
`torchcontrib.optim.swa.bn_update` utility.
.. _Averaging Weights Leads to Wider Optima and Better Generalization:
https://arxiv.org/abs/1803.05407
.. _Improving Consistency-Based Semi-Supervised Learning with Weight
Averaging:
https://arxiv.org/abs/1806.05594
"""
self._auto_mode, (self.swa_start, self.swa_freq) = \
self._check_params(self, swa_start, swa_freq)
self.swa_lr = swa_lr
if self._auto_mode:
if swa_start < 0:
raise ValueError("Invalid swa_start: {}".format(swa_start))
if swa_freq < 1:
raise ValueError("Invalid swa_freq: {}".format(swa_freq))
else:
if self.swa_lr is not None:
warnings.warn(
"Some of swa_start, swa_freq is None, ignoring swa_lr")
# If not in auto mode make all swa parameters None
self.swa_lr = None
self.swa_start = None
self.swa_freq = None
if self.swa_lr is not None and self.swa_lr < 0:
raise ValueError("Invalid SWA learning rate: {}".format(swa_lr))
self.optimizer = optimizer
self.param_groups = self.optimizer.param_groups
self.state = defaultdict(dict)
self.opt_state = self.optimizer.state
for group in self.param_groups:
group['n_avg'] = 0
group['step_counter'] = 0
@staticmethod
def _check_params(self, swa_start, swa_freq):
params = [swa_start, swa_freq]
params_none = [param is None for param in params]
if not all(params_none) and any(params_none):
warnings.warn(
"Some of swa_start, swa_freq is None, ignoring other")
for i, param in enumerate(params):
if param is not None and not isinstance(param, int):
params[i] = int(param)
warnings.warn("Casting swa_start, swa_freq to int")
return not any(params_none), params
def _reset_lr_to_swa(self):
if self.swa_lr is None:
return
for param_group in self.param_groups:
if param_group['step_counter'] >= self.swa_start:
param_group['lr'] = self.swa_lr
def update_swa_group(self, group):
r"""Updates the SWA running averages for the given parameter group.
Arguments:
param_group (dict): Specifies for what parameter group SWA running
averages should be updated
Examples:
>>> # automatic mode
>>> base_opt = torch.optim.SGD([{'params': [x]},
>>> {'params': [y], 'lr': 1e-3}], lr=1e-2, momentum=0.9)
>>> opt = torchcontrib.optim.SWA(base_opt)
>>> for i in range(100):
>>> opt.zero_grad()
>>> loss_fn(model(input), target).backward()
>>> opt.step()
>>> if i > 10 and i % 5 == 0:
>>> # Update SWA for the second parameter group
>>> opt.update_swa_group(opt.param_groups[1])
>>> opt.swap_swa_sgd()
"""
for p in group['params']:
param_state = self.state[p]
if 'swa_buffer' not in param_state:
param_state['swa_buffer'] = torch.zeros_like(p.data)
buf = param_state['swa_buffer']
virtual_decay = 1 / float(group["n_avg"] + 1)
diff = (p.data - buf) * virtual_decay
buf.add_(diff)
group["n_avg"] += 1
def update_swa(self):
r"""Updates the SWA running averages of all optimized parameters.
"""
for group in self.param_groups:
self.update_swa_group(group)
def swap_swa_sgd(self):
r"""Swaps the values of the optimized variables and swa buffers.
It's meant to be called in the end of training to use the collected
swa running averages. It can also be used to evaluate the running
averages during training; to continue training `swap_swa_sgd`
should be called again.
"""
for group in self.param_groups:
for p in group['params']:
param_state = self.state[p]
if 'swa_buffer' not in param_state:
# If swa wasn't applied we don't swap params
warnings.warn(
"SWA wasn't applied to param {}; skipping it".format(p))
continue
buf = param_state['swa_buffer']
tmp = torch.empty_like(p.data)
tmp.copy_(p.data)
p.data.copy_(buf)
buf.copy_(tmp)
def step(self, closure=None):
r"""Performs a single optimization step.
In automatic mode also updates SWA running averages.
"""
self._reset_lr_to_swa()
loss = self.optimizer.step(closure)
for group in self.param_groups:
group["step_counter"] += 1
steps = group["step_counter"]
if self._auto_mode:
if steps > self.swa_start and steps % self.swa_freq == 0:
self.update_swa_group(group)
return loss
def state_dict(self):
r"""Returns the state of SWA as a :class:`dict`.
It contains three entries:
* opt_state - a dict holding current optimization state of the base
optimizer. Its content differs between optimizer classes.
* swa_state - a dict containing current state of SWA. For each
optimized variable it contains swa_buffer keeping the running
average of the variable
* param_groups - a dict containing all parameter groups
"""
opt_state_dict = self.optimizer.state_dict()
swa_state = {(id(k) if isinstance(k, torch.Tensor) else k): v
for k, v in self.state.items()}
opt_state = opt_state_dict["state"]
param_groups = opt_state_dict["param_groups"]
return {"opt_state": opt_state, "swa_state": swa_state,
"param_groups": param_groups}
def load_state_dict(self, state_dict):
r"""Loads the optimizer state.
Args:
state_dict (dict): SWA optimizer state. Should be an object returned
from a call to `state_dict`.
"""
swa_state_dict = {"state": state_dict["swa_state"],
"param_groups": state_dict["param_groups"]}
opt_state_dict = {"state": state_dict["opt_state"],
"param_groups": state_dict["param_groups"]}
super(SWA, self).load_state_dict(swa_state_dict)
self.optimizer.load_state_dict(opt_state_dict)
self.opt_state = self.optimizer.state
def add_param_group(self, param_group):
r"""Add a param group to the :class:`Optimizer` s `param_groups`.
This can be useful when fine tuning a pre-trained network as frozen
layers can be made trainable and added to the :class:`Optimizer` as
training progresses.
Args:
param_group (dict): Specifies what Tensors should be optimized along
with group specific optimization options.
"""
param_group['n_avg'] = 0
param_group['step_counter'] = 0
self.optimizer.add_param_group(param_group)
@staticmethod
def bn_update(loader, model, device=None):
r"""Updates BatchNorm running_mean, running_var buffers in the model.
It performs one pass over data in `loader` to estimate the activation
statistics for BatchNorm layers in the model.
Args:
loader (torch.utils.data.DataLoader): dataset loader to compute the
activation statistics on. Each data batch should be either a
tensor, or a list/tuple whose first element is a tensor
containing data.
model (torch.nn.Module): model for which we seek to update BatchNorm
statistics.
device (torch.device, optional): If set, data will be trasferred to
:attr:`device` before being passed into :attr:`model`.
"""
if not _check_bn(model):
return
was_training = model.training
model.train()
momenta = {}
model.apply(_reset_bn)
model.apply(lambda module: _get_momenta(module, momenta))
n = 0
for input in loader:
if isinstance(input, (list, tuple)):
input = input[0]
b = input.size(0)
momentum = b / float(n + b)
for module in momenta.keys():
module.momentum = momentum
if device is not None:
input = input.to(device)
model(input)
n += b
model.apply(lambda module: _set_momenta(module, momenta))
model.train(was_training)
# BatchNorm utils
def _check_bn_apply(module, flag):
if issubclass(module.__class__, torch.nn.modules.batchnorm._BatchNorm):
flag[0] = True
def _check_bn(model):
flag = [False]
model.apply(lambda module: _check_bn_apply(module, flag))
return flag[0]
def _reset_bn(module):
if issubclass(module.__class__, torch.nn.modules.batchnorm._BatchNorm):
module.running_mean = torch.zeros_like(module.running_mean)
module.running_var = torch.ones_like(module.running_var)
def _get_momenta(module, momenta):
if issubclass(module.__class__, torch.nn.modules.batchnorm._BatchNorm):
momenta[module] = module.momentum
def _set_momenta(module, momenta):
if issubclass(module.__class__, torch.nn.modules.batchnorm._BatchNorm):
module.momentum = momenta[module]
参考文献:
-
随机加权平均 – 在深度学习中获得最优结果的新方法https://cloud.tencent.com/developer/article/1166603
- 优化器的使用 / 学习率的调整 / SWA策略(https://blog.csdn.net/zyw2002/article/details/128258579)
- lr_scheduler用法总结(https://zhuanlan.zhihu.com/p/380795956)
- git上的官方实例(https://github.com/izmailovpavel/contrib_swa_examples)