蚁群算法又称蚂蚁算法,容易与其他算法相结合,但也存在收敛速度慢,容易陷入局部最优等缺点。
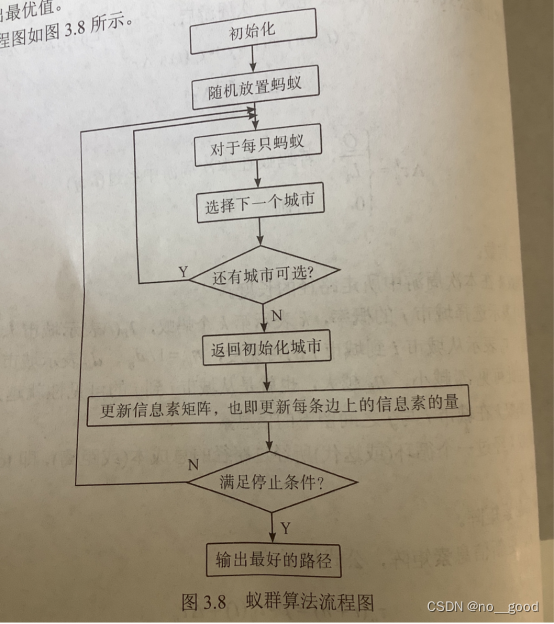
# -*- coding: utf-8 -*-
import random
import copy
import time
import sys
import math
import tkinter # //GUI模块
import threading
from functools import reduce
# 参数
'''
ALPHA:信息启发因子,值越大,则蚂蚁选择之前走过的路径可能性就越大
,值越小,则蚁群搜索范围就会减少,容易陷入局部最优
BETA:Beta值越大,蚁群越就容易选择局部较短路径,这时算法收敛速度会
加快,但是随机性不高,容易得到局部的相对最优
RHO:控制参数,更新信息素矩阵时使用
Q:正常数,信息素计算时使用
'''
(ALPHA, BETA, RHO, Q) = (1.0, 2.0, 0.5, 100.0)
# 城市数,蚁群
(city_num, ant_num) = (50, 50)
distance_x = [
178, 272, 176, 171, 650, 499, 267, 703, 408, 437, 491, 74, 532,
416, 626, 42, 271, 359, 163, 508, 229, 576, 147, 560, 35, 714,
757, 517, 64, 314, 675, 690, 391, 628, 87, 240, 705, 699, 258,
428, 614, 36, 360, 482, 666, 597, 209, 201, 492, 294]
distance_y = [
170, 395, 198, 151, 242, 556, 57, 401, 305, 421, 267, 105, 525,
381, 244, 330, 395, 169, 141, 380, 153, 442, 528, 329, 232, 48,
498, 265, 343, 120, 165, 50, 433, 63, 491, 275, 348, 222, 288,
490, 213, 524, 244, 114, 104, 552, 70, 425, 227, 331]
# 城市距离和信息素
distance_graph = [[0.0 for col in range(city_num)] for raw in range(city_num)] # list里有50个list,每个子list里有50个0.0
pheromone_graph = [[1.0 for col in range(city_num)] for raw in range(city_num)]# 大list里套50个小list,小list里初始为50个1.0
print(f"初始化城市距离矩阵:\n{distance_graph}")
print(f"初始化信息素矩阵:\n{pheromone_graph}")
# ----------- 蚂蚁 -----------
class Ant(object):
# 初始化
def __init__(self, ID):
self.ID = ID # ID
self.__clean_data() # 随机初始化出生点
# 初始数据
def __clean_data(self):
self.path = [] # 当前蚂蚁的路径
self.total_distance = 0.0 # 当前路径的总距离
self.move_count = 0 # 移动次数
self.current_city = -1 # 当前停留的城市
self.open_table_city = [True for i in range(city_num)] # 探索城市的状态
# print(self.open_table_city)
city_index = random.randint(0, city_num - 1) # 随机初始出生点
self.current_city = city_index
self.path.append(city_index)
self.open_table_city[city_index] = False
self.move_count = 1
# 选择下一个城市
def __choice_next_city(self):
next_city = -1
select_citys_prob = [0.0 for i in range(city_num)] # 存储去下个城市的概率
total_prob = 0.0 # 所有概率之和,用于轮盘赌选择城市
# 获取去下一个城市的概率
for i in range(city_num):
if self.open_table_city[i]: # 如果这里是true 那就是这个城市还没去过
try:
# 计算概率:与信息素浓度成正比,与距离成反比 pow(x,y)计算x的y次幂
select_citys_prob[i] = pow(pheromone_graph[self.current_city][i], ALPHA) * pow(
(1.0 / distance_graph[self.current_city][i]), BETA)
total_prob += select_citys_prob[i]
except ZeroDivisionError as e:
print('Ant ID: {ID}, current city: {current}, target city: {target}'.format(ID=self.ID,
current=self.current_city,
target=i))
sys.exit(1)
# 轮盘选择城市
if total_prob > 0.0:
# 产生一个随机概率,0.0--total_prob
temp_prob = random.uniform(0.0, total_prob) # 产生的浮点数范围为【a,b】,所以可能为total_prob
for i in range(city_num):
if self.open_table_city[i]: # 如果这里是true 那就是这个城市还没去过
# 轮次相减
temp_prob -= select_citys_prob[i]
if temp_prob < 0.0: # 最终结果可能为0
next_city = i
break
# 不从概率产生,顺序选择一个未访问城市
# if next_city == -1:
# for i in range(city_num):
# if self.open_table_city[i]:
# next_city = i
# break
if (next_city == -1):
next_city = random.randint(0, city_num - 1)
while ((self.open_table_city[next_city]) == False): # if==False,说明已经遍历过了
next_city = random.randint(0, city_num - 1)
# 返回下一个城市序号
return next_city
# 计算路径总距离
def __cal_total_distance(self):
temp_distance = 0.0
for i in range(1, city_num):
start, end = self.path[i], self.path[i - 1]
temp_distance += distance_graph[start][end]
# 回路
end = self.path[0] # 最后将end置为原点,回到原点
temp_distance += distance_graph[start][end]
self.total_distance = temp_distance
# 移动操作
def __move(self, next_city):
self.path.append(next_city)
self.open_table_city[next_city] = False
self.total_distance += distance_graph[self.current_city][next_city]
self.current_city = next_city
self.move_count += 1
# 搜索路径
def search_path(self):
# 初始化数据
self.__clean_data()
# 搜素路径,遍历完所有城市为止
while self.move_count < city_num:
# 移动到下一个城市
next_city = self.__choice_next_city()
self.__move(next_city)
# 计算路径总长度
self.__cal_total_distance()
# ----------- TSP问题 -----------
class TSP(object):
def __init__(self, root, width=800, height=600, n=city_num):
# 创建画布
self.root = root
self.width = width
self.height = height
# 城市数目初始化为city_num
self.n = n
# tkinter.Canvas
self.canvas = tkinter.Canvas(
root,
width=self.width,
height=self.height,
bg="#EBEBEB", # 背景白色
xscrollincrement=1,
yscrollincrement=1
)
self.canvas.pack(expand=tkinter.YES, fill=tkinter.BOTH)
self.title("TSP蚁群算法(n:初始化 e:开始搜索 s:停止搜索 q:退出程序)")
self.__r = 5 # 节点椭圆半径
self.__lock = threading.RLock() # 线程锁
self.__bindEvents()
self.new()
# 计算城市之间的距离
for i in range(city_num):
for j in range(city_num):
temp_distance = pow((distance_x[i] - distance_x[j]), 2) + pow((distance_y[i] - distance_y[j]), 2)
temp_distance = pow(temp_distance, 0.5)
distance_graph[i][j] = float(int(temp_distance + 0.5))
# 按键响应程序
def __bindEvents(self):
self.root.bind("q", self.quite) # 退出程序
self.root.bind("n", self.new) # 初始化
self.root.bind("e", self.search_path) # 开始搜索
self.root.bind("s", self.stop) # 停止搜索
# 更改标题
def title(self, s):
self.root.title(s)
# 初始化
def new(self, evt=None):
# 停止线程
self.__lock.acquire()
self.__running = False
self.__lock.release()
self.clear() # 清除信息
self.nodes = [] # 节点坐标
self.nodes2 = [] # 节点对象
# 初始化城市节点
for i in range(len(distance_x)):
# 在画布上随机初始坐标
x = distance_x[i]
y = distance_y[i]
self.nodes.append((x, y))
# 生成节点椭圆,半径为self.__r
node = self.canvas.create_oval(x - self.__r,y - self.__r,
x + self.__r, y + self.__r,
fill="#ff0000", # 填充红色
outline="#000000", # 轮廓白色
tags="node",
)
self.nodes2.append(node)
# 显示坐标
self.canvas.create_text(x, y - 10, # 使用create_text方法在坐标(302,77)处绘制文字
text='(' + str(x) + ',' + str(y) + ')', # 所绘制文字的内容
fill='black' # 所绘制文字的颜色为灰色
)
# 顺序连接城市
# self.line(range(city_num))
# 初始城市之间的距离和信息素
for i in range(city_num):
for j in range(city_num):
pheromone_graph[i][j] = 1.0
self.ants = [Ant(ID) for ID in range(ant_num)] # 初始蚁群
self.best_ant = Ant(-1) # 初始最优解
self.best_ant.total_distance = 1 << 31 # 初始最大距离
self.iter = 1 # 初始化迭代次数
# 将节点按order顺序连线
def line(self, order):
# 删除原线
self.canvas.delete("line")
def line2(i1, i2):
p1, p2 = self.nodes[i1], self.nodes[i2]
self.canvas.create_line(p1, p2, fill="#000000", tags="line")
return i2
# order[-1]为初始值
reduce(line2, order, order[-1])
# 清除画布
def clear(self):
for item in self.canvas.find_all():
self.canvas.delete(item)
# 退出程序
def quite(self, evt):
self.__lock.acquire()
self.__running = False
self.__lock.release()
self.root.destroy()
print(u"\n程序已退出...")
sys.exit()
# 停止搜索
def stop(self, evt):
self.__lock.acquire()
self.__running = False
self.__lock.release()
# 开始搜索
def search_path(self, evt=None):
# 开启线程
self.__lock.acquire()
self.__running = True
self.__lock.release()
while self.__running:
# 遍历每一只蚂蚁
for ant in self.ants:
# 搜索一条路径
ant.search_path()
# 与当前最优蚂蚁比较
if ant.total_distance < self.best_ant.total_distance:
# 更新最优解
self.best_ant = copy.deepcopy(ant)
# 更新信息素
self.__update_pheromone_gragh()
print(u"迭代次数:", self.iter, u"最佳路径总距离:", int(self.best_ant.total_distance))
# 连线
self.line(self.best_ant.path)
# 设置标题
self.title("TSP蚁群算法(n:随机初始 e:开始搜索 s:停止搜索 q:退出程序) 迭代次数: %d" % self.iter)
# 更新画布
self.canvas.update()
self.iter += 1
# 更新信息素
def __update_pheromone_gragh(self):
# 获取每只蚂蚁在其路径上留下的信息素
temp_pheromone = [[0.0 for col in range(city_num)] for raw in range(city_num)]
for ant in self.ants:
for i in range(1, city_num):
start, end = ant.path[i - 1], ant.path[i]
# 在路径上的每两个相邻城市间留下信息素,与路径总距离反比
temp_pheromone[start][end] += Q / ant.total_distance
temp_pheromone[end][start] = temp_pheromone[start][end]
# 更新所有城市之间的信息素,旧信息素衰减加上新迭代信息素
for i in range(city_num):
for j in range(city_num):
pheromone_graph[i][j] = pheromone_graph[i][j] * RHO + temp_pheromone[i][j]
# 主循环
def mainloop(self):
self.root.mainloop()
# ----------- 程序的入口处 -----------
if __name__ == '__main__':
TSP(tkinter.Tk()).mainloop()