应用案例
之前学习了逻辑回归,我们现在来做一个案例。
一个图片验证码识别的案例:怎么从图片中准确的识别出正确的数字。
我们分了三步。
第一步:先生成150验证码图片,每个图片有5个数字。图片中有随机的噪点。
具体代码实现:
"""
Date: 2019--10 09:39
User: yz
Email: 1147570523@qq.com
Desc:
"""
from PIL import Image
from PIL import ImageDraw
from PIL import ImageFont
import random
def getRandomStr():
random_num=str(random.randint(0,9))
return random_num
def getRandomColor():
#生成三个0~255的随机数字
num1=random.randint(0,255)
num2=random.randint(0,255)
num3=random.randint(0,255)
return num1,num2,num3
def generate_captcha():
image=Image.new("RGB",(150,50),(255,255,255))
draw=ImageDraw.Draw(image)
font=ImageFont.truetype("LiberationSans-Bold.ttf",size=32)
lable=""
for i in range(5):
#得到一个随机的数字
random_char=getRandomStr()
lable +=random_char
draw.text((10+i*30,0),random_char,getRandomColor(),font=font)
width =150
height=30
for i in range(3):
x1=random.randint(0,width)
x2=random.randint(0,width)
y1=random.randint(0,height)
y2=random.randint(0,height)
draw.line((x1,y1,x2,y2),fill=(0,0,0))
for i in range(5):
draw.point([random.randint(0,width),random.randint(0,height)],fill=getRandomColor())
x=random.randint(0,width)
y=random.randint(0,height)
draw.arc((x,y,x+4,y+4),0,90,fill=(0,0,0))
#保存
image.save(open(''.join(['img/',lable,'.png']),'wb'),'png')
if __name__ == '__main__':
for i in range(150):
generate_captcha()
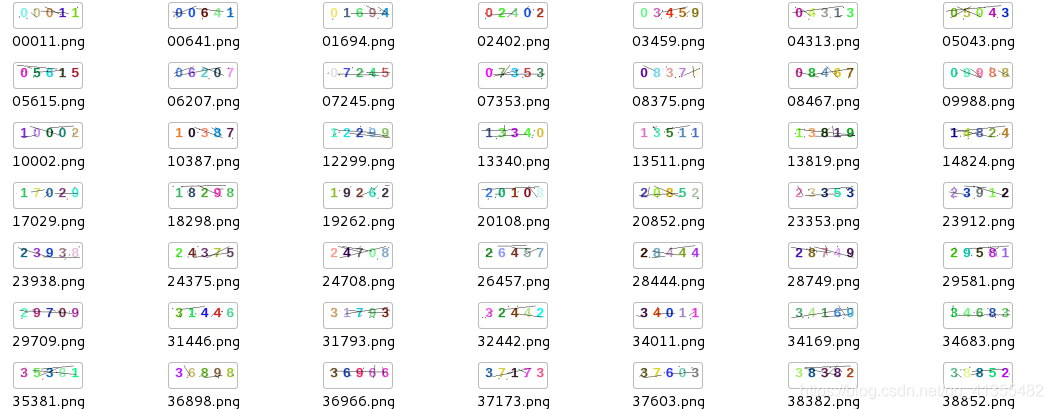
第二步: 对150张验证码图像进行处理。
(1)二值化:首先把图像从RGB3通道转化成Gray1通道,然后把灰度图(0~255)转化成二值图(0,1)
(2)降噪:通过处理孤立点,对二值化的图进行降噪
(3)图片切割:根据像素格,把图片中的所有(5个)数字,分别保存到对应的0~9文件夹下
具体代码实现:
"""
Date: 2019--10 10:49
User: yz
Email: 1147570523@qq.com
Desc:
"""
from PIL import Image
import numpy as np
import matplotlib.pyplot as plt
import os
def binarizaion(path):
img = Image.open(path)
img_gray = img.convert('L')
img_gray = np.array(img_gray)
w, h = img_gray.shape
for x in range(w):
for y in range(h):
gray = img_gray[x, y]
if gray <= 230:
img_gray[x, y] = 0
else:
img_gray[x, y] = 1
plt.figure('')
plt.imshow(img_gray, cmap='gray')
plt.axis('off')
# plt.show()
return img_gray
def noiseReduction(img_gray, label):
w, h = img_gray.shape
for x in range(w):
for y in range(h):
cnt = 0
if img_gray[x,y]==1:
continue
if x == 0 or y == 0 or x == w - 1 or y == h - 1:
img_gray[x, y] = 1
else:
if img_gray[x, y - 1] == 0:
cnt += 1
if img_gray[x, y + 1] == 0:
cnt += 1
if img_gray[x + 1, y] == 0:
cnt += 1
if img_gray[x - 1, y] == 0:
cnt += 1
if img_gray[x - 1, y + 1] == 0:
cnt += 1
if img_gray[x - 1, y - 1] == 0:
cnt += 1
if img_gray[x + 1, y - 1] == 0:
cnt += 1
if img_gray[x + 1, y + 1] == 0:
cnt += 1
if cnt < 4:
img_gray[x, y] = 1
plt.figure('')
plt.imshow(img_gray, cmap='gray')
plt.axis('off')
# plt.show()
plt.savefig(''.join(['imgs_logistic/', label, '.png']))
def cutImg(label):
labels = list(label)
img = Image.open(''.join(['imgs_logistic/', label, '.png']))
for i in range(5):
pic = img.crop((100*(1+i), 170, 100*(1+i)+100, 280))
plt.imshow(pic)
seq = get_save_seq(labels[i])
pic.save(''.join(['cut_number/', str(labels[i]), '/', str(seq), '.png']))
def get_save_seq(num):
numlist = os.listdir(''.join(['cut_number/', num, '/']))
if len(numlist) == 0 or numlist is None:
return 0
else:
max_file = 0
for file in numlist:
if int(file.split('.')[0]) > max_file:
max_file = int(file.split('.')[0])
return int(max_file)+1
def create_dir():
for i in range(10):
os.mkdir(''.join(['cut_number/', str(i)]))
def img_2_clean():
captchas = os.listdir(''.join(['img/']))
for captcha in captchas:
label = captcha.split('.')[0]
img_path = ''.join(['img/', captcha])
# 二值化
im = binarizaion(img_path)
# 降噪
noiseReduction(im, label)
def clean_to_cut():
captchas = os.listdir(''.join(['imgs_logistic/']))
for captcha in captchas:
label = captcha.split('.')[0]
cutImg(label)
if __name__ == '__main__':
img_2_clean()
clean_to_cut()
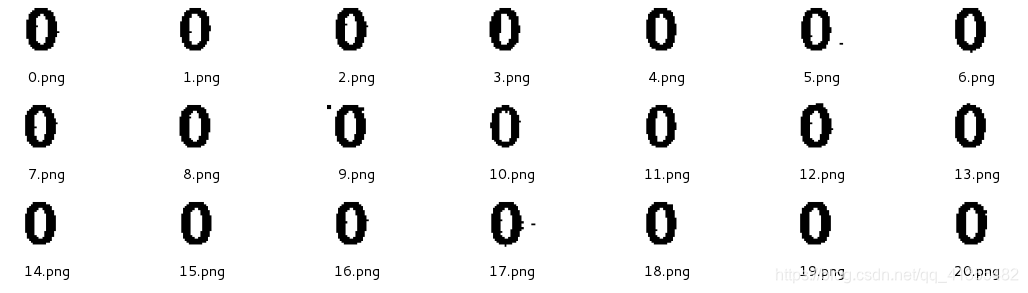
第三步: 利用逻辑回归做模型,来测试一张图片验证码。这里生成模型过程比较漫长,我们可以先生成模型,在做测试。
-
把数据带入逻辑回归进行建模
(1)把切割好的数据,按照X(二位数组),Y(一维数组)的方式传入logisticRegression.fit()函数进行拟合
我们可以通过网格搜索(GridSearch)来进行调参
(2)通过joblib包,把模型保存到本地
-
得到模型后,进行图像验证
(1)根据步骤1,重复操作新的图像
(2)对切割好的每个图像,独立的进行预测
(3)把最后预测结果进行拼接
具体代码实现:
"""
Date: 2019--10 14:26
User: yz
Email: 1147570523@qq.com
Desc:
"""
import os
from PIL import Image
import numpy as np
from sklearn.model_selection import train_test_split
from sklearn.linear_model import LogisticRegression
from sklearn.externals import joblib
from captcha_logistic import *
def load_data():
X,Y=[],[]
cut_list=os.listdir('cut_number/')
for numC in cut_list:
num_list_dir=''.join(['cut_number/',str(numC),'/'])
nums_dir=os.listdir(num_list_dir)
for num_file in nums_dir:
img=Image.open(''.join(['cut_number/',str(numC),'/',num_file]))
img_gray=img.convert('L')
img_array=np.array(img_gray)
w,h=img_array.shape
for x in range(w):
for y in range(h):
gray = img_array[x, y]
if gray <= 240:
img_array[x, y] = 0
else:
img_array[x, y] = 1
img_re=img_array.reshape(1,-1)
X.append(img_re[0])
Y.append(int(numC))
return np.array(X),np.array(Y)
def generate_model(X,Y):
X_train,X_test,Y_train,Y_test=train_test_split(X,Y,test_size=0.3)
log_clf=LogisticRegression(multi_class='ovr',solver='sag',max_iter=10000)
log_clf.fit(X_train,Y_train)
joblib.dump(log_clf,'captcha_model/captcha_model.model')
def get_model():
model=joblib.load('captcha_model/captcha_model.model')
return model
def captcha_predict():
path='captcha_predict/unknown.png'
pre_img_gray=binarizaion(path)
noiseReduction(pre_img_gray,'unknown')
labels=['0','1','2','3','4']
img=Image.open(''.join(['imgs_logistic/unknown.png']))
for i in range(5):
pic=img.crop((100*(1+i),170,100*(1+i)+100,280))
plt.imshow(pic)
pic.save(''.join(['captcha_predict/',labels[i],'.png']))
result=''
model=get_model()
for i in range(5):
path=''.join(['captcha_predict/',labels[i],'.png'])
img=Image.open(path)
img_gray = img.convert('L')
img_array = np.array(img_gray)
w, h = img_array.shape
for x in range(w):
for y in range(h):
gray = img_array[x, y]
if gray <= 240:
img_array[x, y] = 0
else:
img_array[x, y] = 1
img_re = img_array.reshape(1, -1)
X=img_re[0]
Y_pre=model.predict([X])
result=''.join([result,str(Y_pre[0])])
return result
if __name__ == '__main__':
#先生成本地模型,本地模型只需生成一次。
# X,Y=load_data()
# generate_model(X,Y)
#测试。
model=get_model()
result=captcha_predict()
print(result)
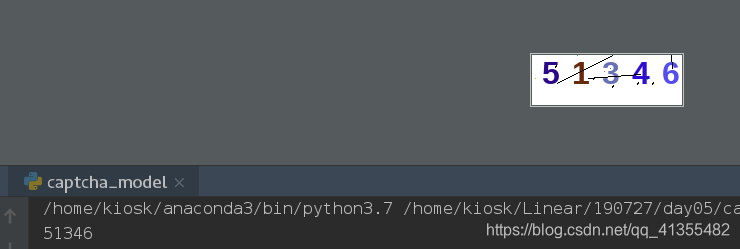
我们的结果测试是很准确的。
本文内容由网友自发贡献,版权归原作者所有,本站不承担相应法律责任。如您发现有涉嫌抄袭侵权的内容,请联系:hwhale#tublm.com(使用前将#替换为@)