用过TensorRT的基本都接触过trtexec[1],可以方便快捷地将你的ONNX模型转换为TensorRT的engine:
./trtexec --onnx=model.onnx
其中原理是啥,这就涉及到了另外一个库onnx-tensorrt[2],可以解析onnx模型并且将onnx中的每一个op转换为TensorRT的op,进而构建得到engine,trtexec转模型的核心就是onnx-tensorrt。
如果没有onnx-tensorrt[3],我们该怎么使用TensorRT去加速你的模型的呢?
幸运的是TensorRT官方提供了API[4]去搭建网络,你可以像使用Pytorch一样去搓一个网络出来,比如TensorRTx[5]这个库,就包含了很多直接使用API搭建出来的TensorRT网络:
nvinfer1::IHostMemory* buildEngineYolov8n(nvinfer1::IBuilder* builder,
nvinfer1::IBuilderConfig* config, nvinfer1::DataType dt, const std::string& wts_path) {
std::map<std::string, nvinfer1::Weights> weightMap = loadWeights(wts_path);
nvinfer1::INetworkDefinition* network = builder->createNetworkV2(0U);
/*******************************************************************************************************
****************************************** YOLOV8 INPUT **********************************************
*******************************************************************************************************/
nvinfer1::ITensor* data = network->addInput(kInputTensorName, dt, nvinfer1::Dims3{3, kInputH, kInputW});
assert(data);
/*******************************************************************************************************
***************************************** YOLOV8 BACKBONE ********************************************
*******************************************************************************************************/
nvinfer1::IElementWiseLayer* conv0 = convBnSiLU(network, weightMap, *data, 16, 3, 2, 1, "model.0");
nvinfer1::IElementWiseLayer* conv1 = convBnSiLU(network, weightMap, *conv0->getOutput(0), 32, 3, 2, 1, "model.1");
nvinfer1::IElementWiseLayer* conv2 = C2F(network, weightMap, *conv1->getOutput(0), 32, 32, 1, true, 0.5, "model.2");
nvinfer1::IElementWiseLayer* conv3 = convBnSiLU(network, weightMap, *conv2->getOutput(0), 64, 3, 2, 1, "model.3");
nvinfer1::IElementWiseLayer* conv4 = C2F(network, weightMap, *conv3->getOutput(0), 64, 64, 2, true, 0.5, "model.4");
nvinfer1::IElementWiseLayer* conv5 = convBnSiLU(network, weightMap, *conv4->getOutput(0), 128, 3, 2, 1, "model.5");
nvinfer1::IElementWiseLayer* conv6 = C2F(network, weightMap, *conv5->getOutput(0), 128, 128, 2, true, 0.5, "model.6");
nvinfer1::IElementWiseLayer* conv7 = convBnSiLU(network, weightMap, *conv6->getOutput(0), 256, 3, 2, 1, "model.7");
nvinfer1::IElementWiseLayer* conv8 = C2F(network, weightMap, *conv7->getOutput(0), 256, 256, 1, true, 0.5, "model.8");
nvinfer1::IElementWiseLayer* conv9 = SPPF(network, weightMap, *conv8->getOutput(0), 256, 256, 5, "model.9");
...
}
这种方式的搭建,相比使用onnx-tensorrt[6]的优点:
不过缺点很显然,搭网络很耗时,还需要你熟悉TensorRT的api,入手期间可能会经历无数的坑。有那时间使用onnx2trt一行命令就转好了,没有onnx2trt灵活。
不过当然不能无脑使用onnx,遇到网络中不支持的算子,或者你的网络比较特殊的话,会直接GG,看看onnx2TensorRT仓库的issue,直到2023年还会有各种各样的op问题: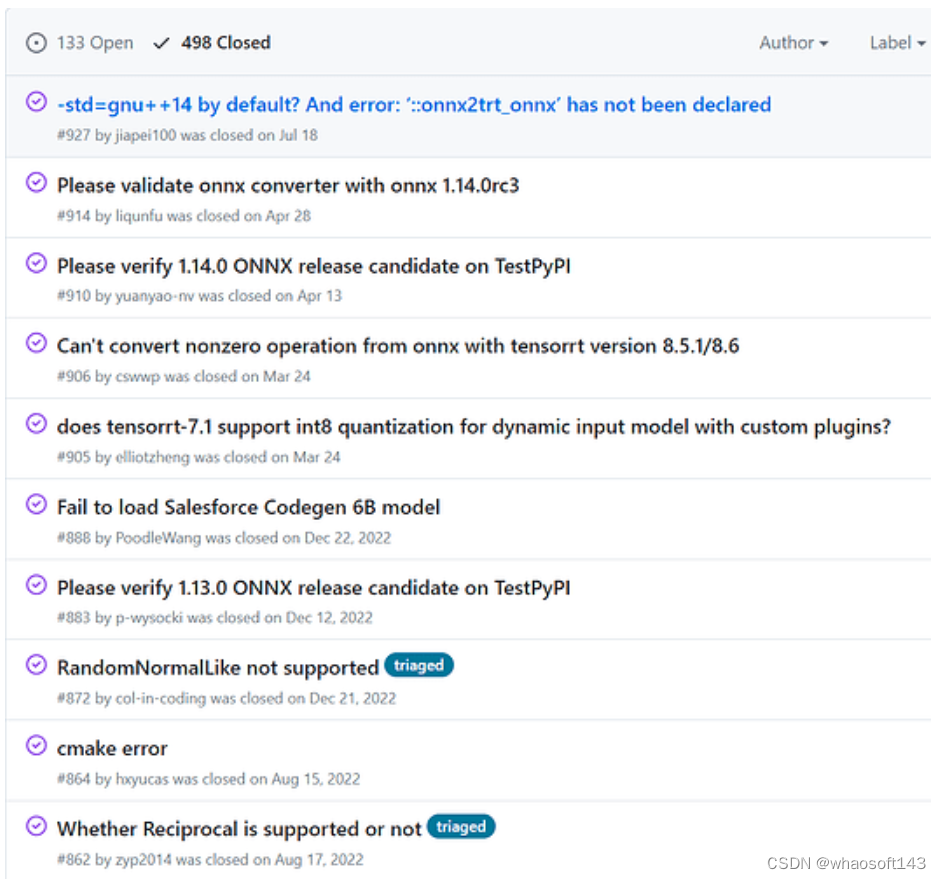
另外,当模型特别大(嗯我说的就是llm),层数特别多的话,onnx就不是很好用了,也不是不能导出来,就是当onnx比较大的时候,看网络结构、定位问题不是很好搞,总得经过onnx这个IR,而ONNX用起来有很多小坑,虽说最后可以完成任务,但过程总归是很辛苦的(苦力活,懂的都懂)。
那么有没有更好的方式呢?同时兼顾灵活性和性能?
更好的方式 v1
想必有些童鞋也用过类似于torch2trt[7]的TensorRT转换工具,通过遍历你的Pytorch网络,在遍历每一个op的时候将每个op转换为相应的TensorRT-op,搭建好网络后就可以build成TensorRT的engine:
model = deeplabv3_resnet50().cuda().eval().half()
data = torch.randn((1, 3, 224, 224)).cuda().half()
print('Running torch2trt...')
model_trt = torch2trt_dynamic(
model, [data], fp16_mode=True, max_workspace_size=1 << 25)
比如下述这个converter,当你模型遍历到torch.nn.functional.leaky_relu
这个op的时候,会执行这个转换脚本生成TensorRT-network的op:ctx.network.add_activation(input_trt, trt.ActivationType.LEAKY_RELU)
。
@tensorrt_converter('torch.nn.functional.leaky_relu')
@tensorrt_converter('torch.nn.functional.leaky_relu_')
def convert_leaky_relu(ctx):
input = get_arg(ctx, 'input', pos=0, default=None)
negative_slope = get_arg(ctx, 'negative_slope', pos=1, default=0.01)
output = ctx.method_return
input_trt = trt_(ctx.network, input)
layer = ctx.network.add_activation(input_trt,
trt.ActivationType.LEAKY_RELU)
layer.alpha = negative_slope
output._trt = layer.get_output(0)
这种方式的好处是修改网络比较简单,因为是直接从你pytorch模型去转换而不是经过onnx,虽然说经过onnx也可以修改网络,但是终归是要经过onnx这个IR,有些op从pytorch->onnx的时候会变,到时候出现了问题不好定位。
另外,需要debug的时候你可以很方便的设置哪些是output(直接在网络中找到你想要设置output的地方,将子模型单独截取出来转换即可),方便定位问题。如果是onnx的话,首先需要获取pytorch-onnx的对应层, 然后在onnx2trt脚本中设置才可以,虽然TensorRT官方也提供了Polygraphy[8]这样的debug工具,但是实际使用起来没有直接在pytorch网络上修改方便。
后续的trtorch,又或者叫torch-TensorRT[9]的工具,原理和torch2trt差不多,也是通过遍历torch的网络去一层一层转化为TensorRT的op:
更好的方式 v2
上述的v1方法,相比onnx2trt更直接一些,可以直接在pytorch模型中进行转换,不过我们拿到的只是build后的TensorRT-engine,中间TensorRT-network网络的搭建过程被隐藏起来了,之后网络中遇到问题,之后想要进一步debug的时候,对于网络的全局观还是要差那么一点,如果能直接debug使用TensorRT-API搭建的网络会更好更直观一点: whaosoft aiot http://143ai.com
class Centernet_dla34(object):
def __init__(self, weights) -> None:
super().__init__()
self.weights = weights
self.levels = [1, 1, 1, 2, 2, 1]
self.channels = [16, 32, 64, 128, 256, 512]
self.down_ratio = 4
self.last_level = 5
self.engine = self.build_engine()
def add_batchnorm_2d(self, input_tensor, parent):
gamma = self.weights[parent + '.weight'].numpy()
beta = self.weights[parent + '.bias'].numpy()
mean = self.weights[parent + '.running_mean'].numpy()
var = self.weights[parent + '.running_var'].numpy()
eps = 1e-5
scale = gamma / np.sqrt(var + eps)
shift = beta - mean * gamma / np.sqrt(var + eps)
power = np.ones_like(scale)
return self.network.add_scale(input=input_tensor.get_output(0), mode=trt.ScaleMode.CHANNEL, shift=shift, scale=scale, power=power)
...
def populate_network(self):
# Configure the network layers based on the self.weights provided.
input_tensor = self.network.add_input(
name=ModelData.INPUT_NAME, dtype=ModelData.DTYPE, shape=ModelData.INPUT_SHAPE)
y = self.add_base(input_tensor, 'module.base')
first_level = int(np.log2(self.down_ratio))
last_level = self.last_level
dla_up = self.add_dla_up(y, first_level, 'module.dla_up')
ida_up = self.add_ida_up(dla_up[:last_level-first_level], self.channels[first_level], [
2 ** i for i in range(last_level - first_level)], 0, 'module.ida_up')
hm = self.add_head(ida_up[-1], 80, 'module.hm')
wh = self.add_head(ida_up[-1], 2, 'module.wh')
reg = self.add_head(ida_up[-1], 2, 'module.reg')
hm.get_output(0).name = 'hm'
wh.get_output(0).name = 'wh'
reg.get_output(0).name = 'reg'
self.network.mark_output(tensor=hm.get_output(0))
self.network.mark_output(tensor=wh.get_output(0))
self.network.mark_output(tensor=reg.get_output(0))
...
但上文也提到过,这种搭建网络的方式较为费事费力,有没有稍微自动化的方法呢?
用过fx[10]的童鞋应该记得有个to_folder方法
model = centernet().cuda()
dummy_input = torch.randn(1, 3, 1024, 1024).cuda()
res_origin = model(dummy_input)
from torch.fx import symbolic_trace
m = symbolic_trace(model.fx_model.cpu())
m.to_folder("fx_debug","centernet_res50")
可以将fx trace后的网络生成出来:
class centernet_res50(torch.nn.Module):
def __init__(self):
super().__init__()
self.backbone = torch.load(r'fx_debug/backbone.pt') # Module( (conv1): Conv2d(3, 64, kernel_size=(7, 7), stride=(2, 2), padding=(3, 3), bias=False) (bn1): BatchNorm2d(64, eps=1e-05, momentum=0.1, affine=True, track_running_stats=True) (relu): ReLU(inplace=True) (maxpool): MaxPool2d(kernel_size=3, stride=2, padding=1, dilation=1, ceil_mode=False) (layer1): Module( (0): Module( (conv1): Conv2d(64, 64, kernel_size=(1, 1), stride=(1, 1), bias=False) (bn1): BatchNorm2d(64, eps=1e-05, momentum=0.1, affine=True, track_running_stats=True) (relu): ReLU(inplace=True) (conv2): Conv2d(64, 64, kernel_size=(3, 3), stride=(1, 1), padding=(1, 1), bias=False) (bn2): BatchNorm2d(64, eps=1e-05, momentum=0.1, affine=True, track_running_stats=True) (conv3): Conv2d(64, 256, kernel_size=(1, 1), stride=(1, 1), bias=False) (bn3): BatchNorm2d(256, eps=1e-05, momentum=0.1, affine=True, track_running_stats=True) (downsample): Module( (0): Conv2d(64, 256, kernel_size=(1, 1), stride=(1, 1), bias=False) (1): BatchNorm2d(256, eps=1e-05, momentum=0.1, affine=True, track_running_stats=True) ) ) (1): Module( (conv1): Conv2d(256, 64, kernel_size=(1, 1), stride=(1, 1), bias=False) (bn1): BatchNorm2d(64, eps=1e-05, momentum=0.1, affine=True, track_running_stats=True) (relu): ReLU(inplace=True) (conv2): Conv2d(64, 64, kernel_size=(3, 3), stride=(1, 1), padding=(1, 1), bias=False) (bn2): BatchNorm2d(64, eps=1e-05, momentum=0.1, affine=True, track_running_stats=True) (conv3): Conv2d(64, 256, kernel_size=(1, 1), stride=(1, 1), bias=False) (bn3): BatchNorm2d(256, eps=1e-05, momentum=0.1, affine=True, track_running_stats=True) ) (2): Module( (conv1): Conv2d(256, 64, kernel_size=(1, 1), stride=(1, 1), bias=False) (bn1): BatchNorm2d(64, eps=1e-05, momentum=0.1, affine=True, track_running_stats=True) (relu): ReLU(inplace=True) (conv2): Conv2d(64, 64, kernel_size=(3, 3), stride=(1, 1), padding=(1, 1), bias=False) (bn2): BatchNorm2d(64, eps=1e-05, momentum=0.1, affine=True, track_running_stats=True) (conv3): Conv2d(64, 256, kernel_size=(1, 1), stride=(1, 1), bias=False) (bn3): BatchNorm2d(256, eps=1e-05, momentum=0.1, affine=True, track_running_stats=True) ) ) (layer2): Module( (0): Module( (conv1): Conv2d(256, 128, kernel_size=(1, 1), stride=(1, 1), bias=False) (bn1): BatchNorm2d(128, eps=1e-05, momentum=0.1, affine=True, track_running_stats=True) (relu): ReLU(inplace=True) (conv2): Conv2d(128, 128, kernel_size=(3, 3), stride=(2, 2), padding=(1, 1), bias=False) (bn2): BatchNorm2d(128, eps=1e-05, momentum=0.1, affine=True, track_running_stats=True) (conv3): Conv2d(128, 512, kernel_size=(1, 1), stride=(1, 1), bias=False) (bn3): BatchNorm2d(512, eps=1e-05, momentum=0.1, affine=True, track_running_stats=True) (downsample): Module( (0): Conv2d(256, 512, kernel_size=(1, 1), stride=(2, 2), bias=False) (1): BatchNorm2d(512, eps=1e-05, momentum=0.1, affine=True, track_running_stats=True) ) ) (1): Module( (conv1): Conv2d(512, 128, kernel_size=(1, 1), stride=(1, 1), bias=False) (bn1): BatchNorm2d(128, eps=1e-05, momentum=0.1, affine=True, track_running_stats=True) (relu): ReLU(inplace=True) (conv2): Conv2d(128, 128, kernel_size=(3, 3), stride=(1, 1), padding=(1, 1), bias=False) (bn2): BatchNorm2d(128, eps=1e-05, momentum=0.1, affine=True, track_running_stats=True) (conv3): Conv2d(128, 512, kernel_size=(1, 1), stride=(1, 1), bias=False) (bn3): BatchNorm2d(512, eps=1e-05, momentum=0.1, affine=True, track_running_stats=True) ) (2): Module( (conv1): Conv2d(512, 128, kernel_size=(1, 1), stride=(1, 1), bias=False) (bn1): BatchNorm2d(128, eps=1e-05, momentum=0.1, affine=True, track_running_stats=True) (relu): ReLU(inplace=True) (conv2): Conv2d(128, 128, kernel_size=(3, 3), stride=(1, 1), padding=(1, 1), bias=False) (bn2): BatchNorm2d(128, eps=1e-05, momentum=0.1, affine=True, track_running_stats=True) (conv3): Conv2d(128, 512, kernel_size=(1, 1), stride=(1, 1), bias=False) (bn3): BatchNorm2d(512, eps=1e-05, momentum=0.1, affine=True, track_running_stats=True) ) (3): Module( (conv1): Conv2d(512, 128, kernel_size=(1, 1), stride=(1, 1), bias=False) (bn1): BatchNorm2d(128, eps=1e-05, momentum=0.1, affine=True, track_running_stats=True) (relu): ReLU(inplace=True) (conv2): Conv2d(128, 128, kernel_size=(3, 3), stride=(1, 1), padding=(1, 1), bias=False) (bn2): BatchNorm2d(128, eps=1e-05, momentum=0.1, affine=True, track_running_stats=True) (conv3): Conv2d(128, 512, kernel_size=(1, 1), stride=(1, 1), bias=False) (bn3): BatchNorm2d(512, eps=1e-05, momentum=0.1, affine=True, track_running_stats=True) ) ) (layer3): Module( (0): Module( (conv1): Conv2d(512, 256, kernel_size=(1, 1), stride=(1, 1), bias=False) (bn1): BatchNorm2d(256, eps=1e-05, momentum=0.1, affine=True, track_running_stats=True) (relu): ReLU(inplace=True) (conv2): Conv2d(256, 256, kernel_size=(3, 3), stride=(2, 2), padding=(1, 1), bias=False) (bn2): BatchNorm2d(256, eps=1e-05, momentum=0.1, affine=True, track_running_stats=True) (conv3): Conv2d(256, 1024, kernel_size=(1, 1), stride=(1, 1), bias=False) (bn3): BatchNorm2d(1024, eps=1e-05, momentum=0.1, affine=True, track_running_stats=True) (downsample): Module( (0): Conv2d(512, 1024, kernel_size=(1, 1), stride=(2, 2), bias=False) (1): BatchNorm2d(1024, eps=1e-05, momentum=0.1, affine=True, track_running_stats=True) ) ) (1): Module( (conv1): Conv2d(1024, 256, kernel_size=(1, 1), stride=(1, 1), bias=False) (bn1): BatchNorm2d(256, eps=1e-05, momentum=0.1, affine=True, track_running_stats=True) (relu): ReLU(inplace=True) (conv2): Conv2d(256, 256, kernel_size=(3, 3), stride=(1, 1), padding=(1, 1), bias=False) (bn2): BatchNorm2d(256, eps=1e-05, momentum=0.1, affine=True, track_running_stats=True) (conv3): Conv2d(256, 1024, kernel_size=(1, 1), stride=(1, 1), bias=False) (bn3): BatchNorm2d(1024, eps=1e-05, momentum=0.1, affine=True, track_running_stats=True) ) (2): Module( (conv1): Conv2d(1024, 256, kernel_size=(1, 1), stride=(1, 1), bias=False) (bn1): BatchNorm2d(256, eps=1e-05, momentum=0.1, affine=True, track_running_stats=True) (relu): ReLU(inplace=True) (conv2): Conv2d(256, 256, kernel_size=(3, 3), stride=(1, 1), padding=(1, 1), bias=False) (bn2): BatchNorm2d(256, eps=1e-05, momentum=0.1, affine=True, track_running_stats=True) (conv3): Conv2d(256, 1024, kernel_size=(1, 1), stride=(1, 1), bias=False) (bn3): BatchNorm2d(1024, eps=1e-05, momentum=0.1, affine=True, track_running_stats=True) ) (3): Module( (conv1): Conv2d(1024, 256, kernel_size=(1, 1), stride=(1, 1), bias=False) (bn1): BatchNorm2d(256, eps=1e-05, momentum=0.1, affine=True, track_running_stats=True) (relu): ReLU(inplace=True) (conv2): Conv2d(256, 256, kernel_size=(3, 3), stride=(1, 1), padding=(1, 1), bias=False) (bn2): BatchNorm2d(256, eps=1e-05, momentum=0.1, affine=True, track_running_stats=True) (conv3): Conv2d(256, 1024, kernel_size=(1, 1), stride=(1, 1), bias=False) (bn3): BatchNorm2d(1024, eps=1e-05, momentum=0.1, affine=True, track_running_stats=True) ) (4): Module( (conv1): Conv2d(1024, 256, kernel_size=(1, 1), stride=(1, 1), bias=False) (bn1): BatchNorm2d(256, eps=1e-05, momentum=0.1, affine=True, track_running_stats=True) (relu): ReLU(inplace=True) (conv2): Conv2d(256, 256, kernel_size=(3, 3), stride=(1, 1), padding=(1, 1), bias=False) (bn2): BatchNorm2d(256, eps=1e-05, momentum=0.1, affine=True, track_running_stats=True) (conv3): Conv2d(256, 1024, kernel_size=(1, 1), stride=(1, 1), bias=False) (bn3): BatchNorm2d(1024, eps=1e-05, momentum=0.1, affine=True, track_running_stats=True) ) (5): Module( (conv1): Conv2d(1024, 256, kernel_size=(1, 1), stride=(1, 1), bias=False) (bn1): BatchNorm2d(256, eps=1e-05, momentum=0.1, affine=True, track_running_stats=True) (relu): ReLU(inplace=True) (conv2): Conv2d(256, 256, kernel_size=(3, 3), stride=(1, 1), padding=(1, 1), bias=False) (bn2): BatchNorm2d(256, eps=1e-05, momentum=0.1, affine=True, track_running_stats=True) (conv3): Conv2d(256, 1024, kernel_size=(1, 1), stride=(1, 1), bias=False) (bn3): BatchNorm2d(1024, eps=1e-05, momentum=0.1, affine=True, track_running_stats=True) ) ) (layer4): Module( (0): Module( (conv1): Conv2d(1024, 512, kernel_size=(1, 1), stride=(1, 1), bias=False) (bn1): BatchNorm2d(512, eps=1e-05, momentum=0.1, affine=True, track_running_stats=True) (relu): ReLU(inplace=True) (conv2): Conv2d(512, 512, kernel_size=(3, 3), stride=(2, 2), padding=(1, 1), bias=False) (bn2): BatchNorm2d(512, eps=1e-05, momentum=0.1, affine=True, track_running_stats=True) (conv3): Conv2d(512, 2048, kernel_size=(1, 1), stride=(1, 1), bias=False) (bn3): BatchNorm2d(2048, eps=1e-05, momentum=0.1, affine=True, track_running_stats=True) (downsample): Module( (0): Conv2d(1024, 2048, kernel_size=(1, 1), stride=(2, 2), bias=False) (1): BatchNorm2d(2048, eps=1e-05, momentum=0.1, affine=True, track_running_stats=True) ) ) (1): Module( (conv1): Conv2d(2048, 512, kernel_size=(1, 1), stride=(1, 1), bias=False) (bn1): BatchNorm2d(512, eps=1e-05, momentum=0.1, affine=True, track_running_stats=True) (relu): ReLU(inplace=True) (conv2): Conv2d(512, 512, kernel_size=(3, 3), stride=(1, 1), padding=(1, 1), bias=False) (bn2): BatchNorm2d(512, eps=1e-05, momentum=0.1, affine=True, track_running_stats=True) (conv3): Conv2d(512, 2048, kernel_size=(1, 1), stride=(1, 1), bias=False) (bn3): BatchNorm2d(2048, eps=1e-05, momentum=0.1, affine=True, track_running_stats=True) ) (2): Module( (conv1): Conv2d(2048, 512, kernel_size=(1, 1), stride=(1, 1), bias=False) (bn1): BatchNorm2d(512, eps=1e-05, momentum=0.1, affine=True, track_running_stats=True) (relu): ReLU(inplace=True) (conv2): Conv2d(512, 512, kernel_size=(3, 3), stride=(1, 1), padding=(1, 1), bias=False) (bn2): BatchNorm2d(512, eps=1e-05, momentum=0.1, affine=True, track_running_stats=True) (conv3): Conv2d(512, 2048, kernel_size=(1, 1), stride=(1, 1), bias=False) (bn3): BatchNorm2d(2048, eps=1e-05, momentum=0.1, affine=True, track_running_stats=True) ) ) )
self.upsampler = torch.load(r'fx_debug/upsampler.pt') # Module( (deconv_layers): Module( (0): ConvTranspose2d(2048, 256, kernel_size=(4, 4), stride=(2, 2), padding=(1, 1), bias=False) (1): BatchNorm2d(256, eps=1e-05, momentum=0.1, affine=True, track_running_stats=True) (2): ReLU(inplace=True) (3): ConvTranspose2d(256, 256, kernel_size=(4, 4), stride=(2, 2), padding=(1, 1), bias=False) (4): BatchNorm2d(256, eps=1e-05, momentum=0.1, affine=True, track_running_stats=True) (5): ReLU(inplace=True) (6): ConvTranspose2d(256, 256, kernel_size=(4, 4), stride=(2, 2), padding=(1, 1), bias=False) (7): BatchNorm2d(256, eps=1e-05, momentum=0.1, affine=True, track_running_stats=True) (8): ReLU(inplace=True) ) )
self.head = torch.load(r'fx_debug/head.pt') # Module( (hm): Module( (0): Conv2d(256, 64, kernel_size=(3, 3), stride=(1, 1), padding=(1, 1)) (1): ReLU(inplace=True) (2): Conv2d(64, 3, kernel_size=(1, 1), stride=(1, 1)) ) (wh): Module( (0): Conv2d(256, 64, kernel_size=(3, 3), stride=(1, 1), padding=(1, 1)) (1): ReLU(inplace=True) (2): Conv2d(64, 2, kernel_size=(1, 1), stride=(1, 1)) ) (reg): Module( (0): Conv2d(256, 64, kernel_size=(3, 3), stride=(1, 1), padding=(1, 1)) (1): ReLU(inplace=True) (2): Conv2d(64, 2, kernel_size=(1, 1), stride=(1, 1)) ) )
self.load_state_dict(torch.load(r'fx_debug/state_dict.pt'))
def forward(self, input):
input_1 = input
backbone_conv1 = self.backbone.conv1(input_1); input_1 = None
backbone_bn1 = self.backbone.bn1(backbone_conv1); backbone_conv1 = None
backbone_relu = self.backbone.relu(backbone_bn1); backbone_bn1 = None
backbone_maxpool = self.backbone.maxpool(backbone_relu); backbone_relu = None
...
head_reg_1 = getattr(self.head.reg, "1")(head_reg_0); head_reg_0 = None
head_reg_2 = getattr(self.head.reg, "2")(head_reg_1); head_reg_1 = None
return (head_hm_2, head_wh_2, head_reg_2)
if __name__ == '__main__':
model = centernet_res50()
dummy_input = torch.randn(1, 3, 1024, 1024)
output = model(dummy_input)
通过这种方式我们可以简单将trace后模型直接导出成py文件,然后自然而然地可以看到模型的网络结构,这里是拿到了Pytorch模型。
既然可以生成Pytorch模型,那么可不可以生成直接利用TensorRT-API搭建的网络呢?
我们先仿照TensorRT-API的方式去实现类似于Pytorch的network接口:
class Downsample2D(Module):
def __init__(self,
channels,
use_conv=False,
out_channels=None,
padding=1) -> None:
super().__init__()
self.channels = channels
self.out_channels = out_channels or channels
self.use_conv = use_conv
self.padding = padding
stride = (2, 2)
if use_conv:
self.conv = Conv2d(self.channels,
self.out_channels, (3, 3),
stride=stride,
padding=(padding, padding))
else:
assert self.channels == self.out_channels
self.conv = AvgPool2d(kernel_size=stride, stride=stride)
def forward(self, hidden_states):
assert not hidden_states.is_dynamic()
batch, channels, _, _ = hidden_states.size()
assert channels == self.channels
hidden_states = self.conv(hidden_states)
return hidden_states
是不是很像Pytorch的网络结构,但这里继承的Module是模仿nn.Module单独实现的一个模块。 细节先不介绍了,这里的类成员Conv2d看起来和Pytorch版本的区别不大:
class Conv2d(Module):
def __init__(
self,
in_channels: int,
out_channels: int,
kernel_size: Tuple[int, int],
stride: Tuple[int, int] = (1, 1),
padding: Tuple[int, int] = (0, 0),
dilation: Tuple[int, int] = (1, 1),
groups: int = 1,
bias: bool = True,
padding_mode: str = 'zeros', # TODO: refine this type
dtype=None) -> None:
super().__init__()
if groups <= 0:
raise ValueError('groups must be a positive integer')
if in_channels % groups != 0:
raise ValueError('in_channels must be divisible by groups')
if out_channels % groups != 0:
raise ValueError('out_channels must be divisible by groups')
self.in_channels = in_channels
self.out_channels = out_channels
self.kernel_size = kernel_size
self.stride = stride
self.padding = padding
self.dilation = dilation
self.groups = groups
self.padding_mode = padding_mode
self.weight = Parameter(shape=(out_channels, in_channels // groups,
*kernel_size),
dtype=dtype)
if bias:
self.bias = Parameter(shape=(out_channels, ), dtype=dtype)
else:
self.register_parameter('bias', None)
def forward(self, input):
return conv2d(input, self.weight.value,
None if self.bias is None else self.bias.value,
self.stride, self.padding, self.dilation, self.groups)
是不是很像Pytorch的网络结构,但这里继承的Module是模仿nn.Module单独实现的一个模块。细节先不介绍了,这里的类成员Conv2d看起来和Pytorch版本的区别不大:
class Conv2d(Module):
def __init__(
self,
in_channels: int,
out_channels: int,
kernel_size: Tuple[int, int],
stride: Tuple[int, int] = (1, 1),
padding: Tuple[int, int] = (0, 0),
dilation: Tuple[int, int] = (1, 1),
groups: int = 1,
bias: bool = True,
padding_mode: str = 'zeros', # TODO: refine this type
dtype=None) -> None:
super().__init__()
if groups <= 0:
raise ValueError('groups must be a positive integer')
if in_channels % groups != 0:
raise ValueError('in_channels must be divisible by groups')
if out_channels % groups != 0:
raise ValueError('out_channels must be divisible by groups')
self.in_channels = in_channels
self.out_channels = out_channels
self.kernel_size = kernel_size
self.stride = stride
self.padding = padding
self.dilation = dilation
self.groups = groups
self.padding_mode = padding_mode
self.weight = Parameter(shape=(out_channels, in_channels // groups,
*kernel_size),
dtype=dtype)
if bias:
self.bias = Parameter(shape=(out_channels, ), dtype=dtype)
else:
self.register_parameter('bias', None)
def forward(self, input):
return conv2d(input, self.weight.value,
None if self.bias is None else self.bias.value,
self.stride, self.padding, self.dilation, self.groups)
那我们看核心实现conv2d(input, self.weight.value,...
:
def conv2d(input: Tensor,
weight: Tensor,
bias: Optional[Tensor] = None,
stride: Tuple[int, int] = (1, 1),
padding: Tuple[int, int] = (0, 0),
dilation: Tuple[int, int] = (1, 1),
groups: int = 1) -> Tensor:
assert not input.is_dynamic()
ndim = input.ndim()
if ndim == 3:
input = expand_dims(input, 0)
noutput = weight.size()[0]
kernel_size = (weight.size()[-2], weight.size()[-1])
is_weight_constant = (weight.producer is not None
and weight.producer.type == trt.LayerType.CONSTANT)
weight = weight.producer.weights if is_weight_constant else trt.Weights()
if bias is not None:
is_bias_constant = (bias.producer is not None
and bias.producer.type == trt.LayerType.CONSTANT)
bias = bias.producer.weights if is_bias_constant else trt.Weights()
layer = default_trtnet().add_convolution_nd(input.trt_tensor, noutput,
kernel_size, weight, bias)
layer.stride_nd = stride
layer.padding_nd = padding
layer.dilation = dilation
layer.num_groups = groups
if not is_weight_constant:
layer.set_input(1, weight.trt_tensor)
if bias is not None and not is_bias_constant:
layer.set_input(2, bias.trt_tensor)
output = _create_tensor(layer.get_output(0), layer)
if ndim == 3:
return output.view(
concat([output.size(1),
output.size(2),
output.size(3)]))
return output
可以看到conv2d的核心实现就是利用TensorRT-API去搭建conv网络。
看到这里,想一想如果可以直接将trace后的网络直接使用类似于Pytorch的TensorRT-API搭建,然后生成,是不是就类似于直接生成一个利用TensorRT-API搭建的网络?
后记
当然这只是个抛砖引玉,很多细节其实还没有提到,我之前也用过一些其他公司的类似于TensorRT的工具,在转换完模型后可以直接生成利用该推理后端API搭建的网络文件(可以是cpp,也可以是python),当然权重和参数也在里头了,如果是量化的话,量化参数也可以放到里头,可以做的事情有很多。这种方式的话,我们可以对推理框架即将要优化的网络一目了然,在修改或者调试的情况下都比较方便。
这里仅是简单的讨论,至于后续的细节实现,之后老潘也会继续写一些文章,大家有想法也可以留言哈~
参考
-
https://pytorch.org/docs/stable/fx.html
-
https://mp.weixin.qq.com/s/CQaqirFwTDUSuBFJ3Hz7_w
-
https://github.com/wang-xinyu/tensorrtx/tree/master/yolov8