一、录制rosbag
二、播放rosbag并用rviz查看topic,记下rgb和depth流话题名
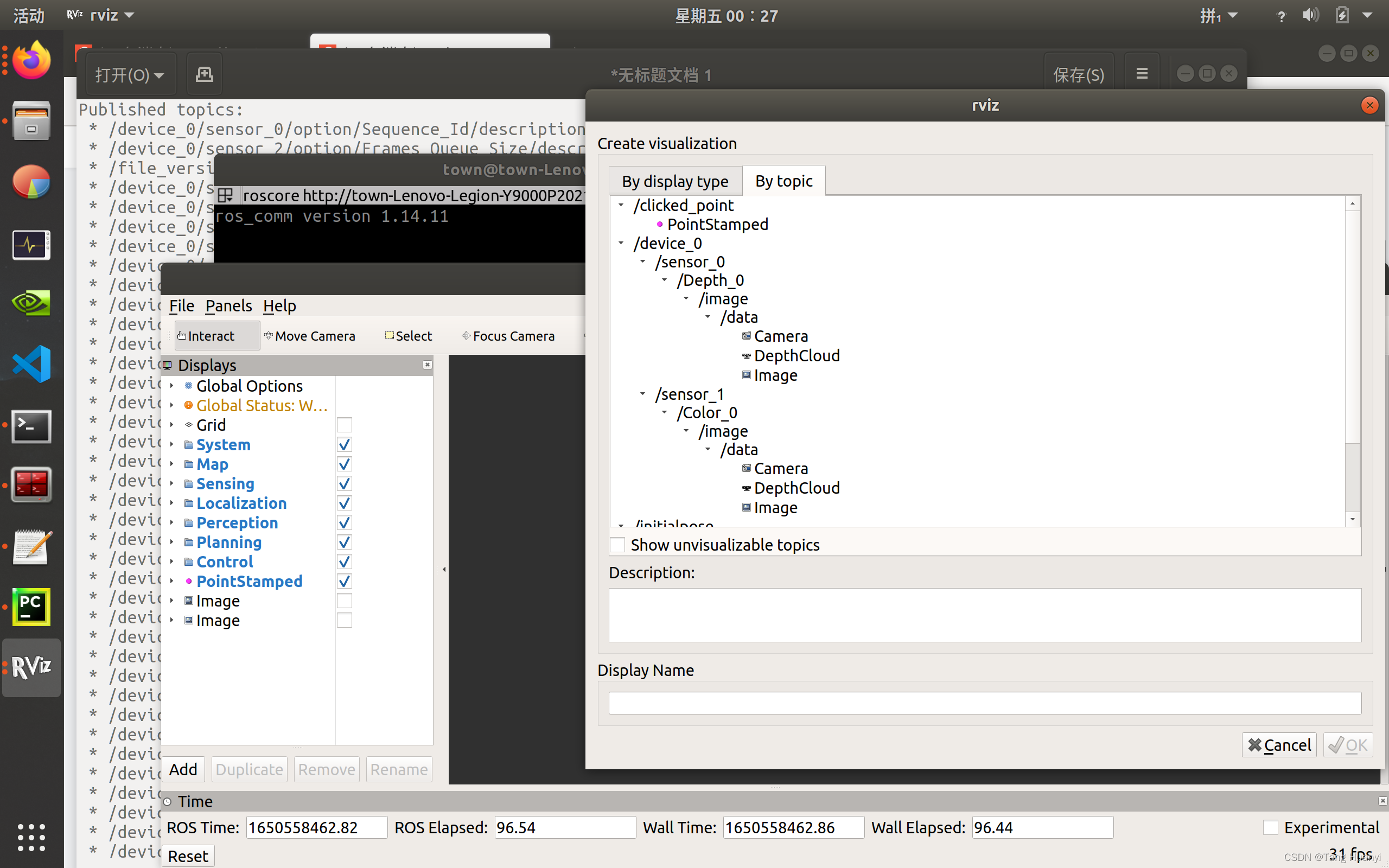
三、用如下脚本(python2而不是3)保存rgb和depth图片同时生成rgb.txt、depth.txt
import roslib
import rosbag
import rospy
import cv2
import os
from sensor_msgs.msg import Image
from cv_bridge import CvBridge
from cv_bridge import CvBridgeError
rgb = '/home/town/data/dataset/rgb/'
depth = '/home/town/data/dataset/depth/'
bridge = CvBridge()
file_handle1 = open('/home/town/data/dataset/rgb.txt', 'w')
file_handle2 = open('/home/town/data/dataset/depth.txt', 'w')
with rosbag.Bag('/home/town/data/20220423_054352.bag', 'r') as bag:
for topic,msg,t in bag.read_messages():
if topic == "/device_0/sensor_1/Color_0/image/data":
cv_image = bridge.imgmsg_to_cv2(msg,"bgr8")
timestr = "%.6f" % msg.header.stamp.to_sec()
image_name = timestr+ ".png"
path = "rgb/" + image_name
file_handle1.write(timestr + " " + path + '\n')
cv2.imwrite(rgb + image_name, cv_image)
if topic == "/device_0/sensor_0/Depth_0/image/data":
cv_image = bridge.imgmsg_to_cv2(msg)
timestr = "%.6f" % msg.header.stamp.to_sec()
image_name = timestr+ ".png"
path = "depth/" + image_name
file_handle2.write(timestr + " " + path + '\n')
cv2.imwrite(depth + image_name, cv_image)
file_handle1.close()
file_handle2.close()
可以把alias python='/usr/bin/python2.7’写进bashrc,用完后记得注释掉。
四、用该脚本associate.py生成associate.txt
import argparse
import sys
import os
import numpy
def read_file_list(filename):
"""
Reads a trajectory from a text file.
File format:
The file format is "stamp d1 d2 d3 ...", where stamp denotes the time stamp (to be matched)
and "d1 d2 d3.." is arbitary data (e.g., a 3D position and 3D orientation) associated to this timestamp.
Input:
filename -- File name
Output:
dict -- dictionary of (stamp,data) tuples
"""
file = open(filename)
data = file.read()
lines = data.replace(","," ").replace("\t"," ").split("\n")
list = [[v.strip() for v in line.split(" ") if v.strip()!=""] for line in lines if len(line)>0 and line[0]!="#"]
list = [(float(l[0]),l[1:]) for l in list if len(l)>1]
return dict(list)
def associate(first_list, second_list,offset,max_difference):
"""
Associate two dictionaries of (stamp,data). As the time stamps never match exactly, we aim
to find the closest match for every input tuple.
Input:
first_list -- first dictionary of (stamp,data) tuples
second_list -- second dictionary of (stamp,data) tuples
offset -- time offset between both dictionaries (e.g., to model the delay between the sensors)
max_difference -- search radius for candidate generation
Output:
matches -- list of matched tuples ((stamp1,data1),(stamp2,data2))
"""
first_keys = first_list.keys()
second_keys = second_list.keys()
potential_matches = [(abs(a - (b + offset)), a, b)
for a in first_keys
for b in second_keys
if abs(a - (b + offset)) < max_difference]
potential_matches.sort()
matches = []
for diff, a, b in potential_matches:
if a in first_keys and b in second_keys:
first_keys.remove(a)
second_keys.remove(b)
matches.append((a, b))
matches.sort()
return matches
if __name__ == '__main__':
parser = argparse.ArgumentParser(description='''
This script takes two data files with timestamps and associates them
''')
parser.add_argument('first_file', help='first text file (format: timestamp data)')
parser.add_argument('second_file', help='second text file (format: timestamp data)')
parser.add_argument('--first_only', help='only output associated lines from first file', action='store_true')
parser.add_argument('--offset', help='time offset added to the timestamps of the second file (default: 0.0)',default=0.0)
parser.add_argument('--max_difference', help='maximally allowed time difference for matching entries (default: 0.02)',default=0.02)
args = parser.parse_args()
first_list = read_file_list(args.first_file)
second_list = read_file_list(args.second_file)
matches = associate(first_list, second_list,float(args.offset),float(args.max_difference))
if args.first_only:
for a,b in matches:
print("%f %s"%(a," ".join(first_list[a])))
else:
for a,b in matches:
print("%f %s %f %s"%(a," ".join(first_list[a]),b-float(args.offset)," ".join(second_list[b])))
python2 associate.py depth.txt rgb.txt > associate.txt
python2 associate.py rgb.txt depth.txt > associate.txt
确保associate.txt内rgb在前?
五、测试
/Examples/RGB-D/rgbd_tum Vocabulary/ORBvoc.txt Examples/RGB-D/TUM1.yaml ./rgbd_dataset_freiburg1_desk/ ./fr1_desk.txt
/Examples/RGB-D/rgbd_tum ./Vocabulary/ORBvoc.txt ./Examples/RGB-D/D435i.yaml ./dataset/ ./dataset/associate.txt
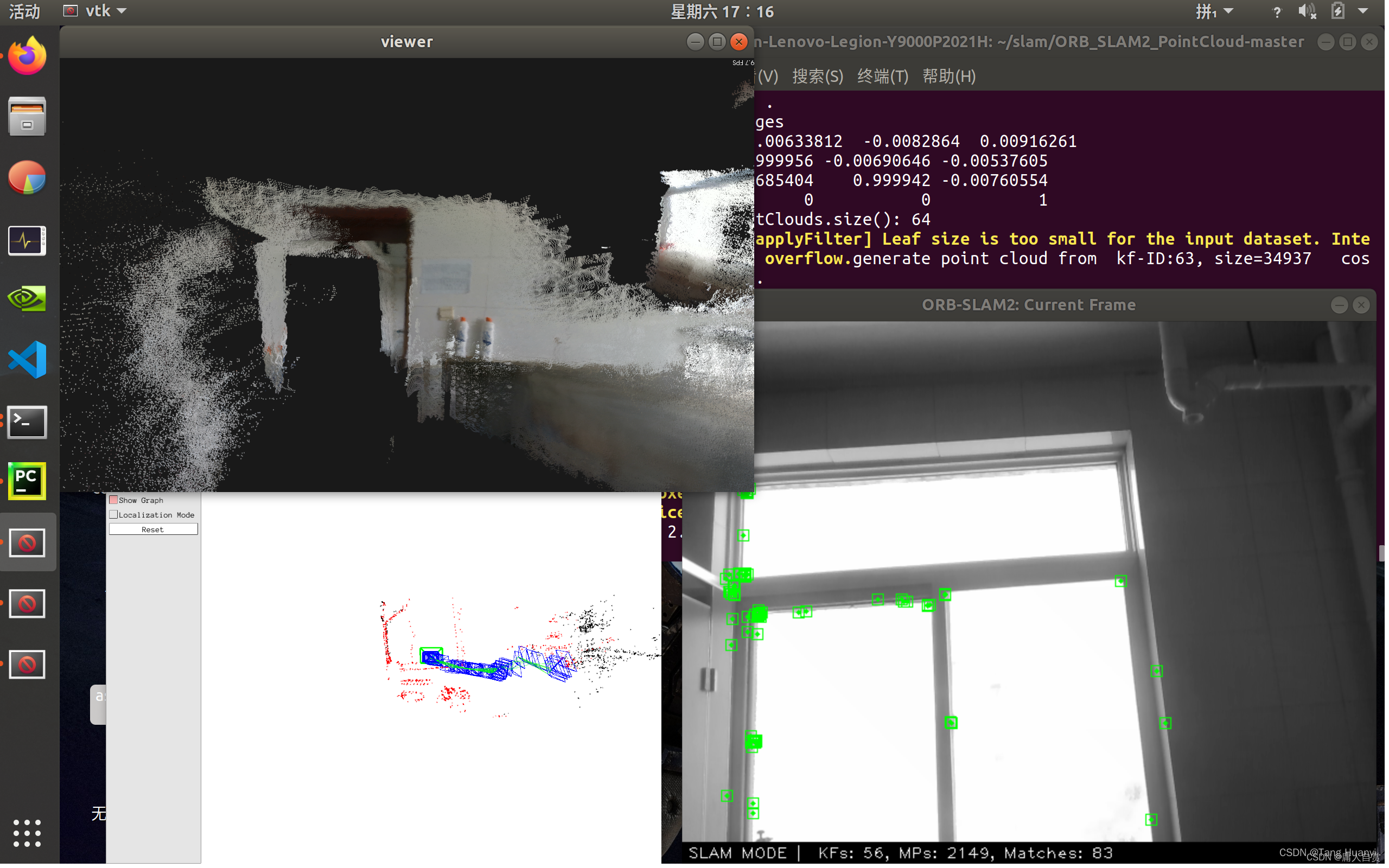
若遇到Track lost soon after initialisation, reseting…,不妨把数据集前一部分图片舍弃后再试试。
#附一份D435i.yaml
%YAML:1.0
Camera.fx: 615.9417724609375
Camera.fy: 616.0935668945312
Camera.cx: 322.3533630371094
Camera.cy: 240.44674682617188
Camera.k1: 0.0
Camera.k2: 0.0
Camera.p1: 0.0
Camera.p2: 0.0
Camera.p3: 0.0
Camera.width: 640
Camera.height: 480
Camera.fps: 30.0
Camera.bf: 30.797
Camera.RGB: 1
ThDepth: 40.0
DepthMapFactor: 1000.0
ORBextractor.nFeatures: 1000
ORBextractor.scaleFactor: 1.2
ORBextractor.nLevels: 8
ORBextractor.iniThFAST: 20
ORBextractor.minThFAST: 7
Viewer.KeyFrameSize: 0.05
Viewer.KeyFrameLineWidth: 1
Viewer.GraphLineWidth: 0.9
Viewer.PointSize: 2
Viewer.CameraSize: 0.08
Viewer.CameraLineWidth: 3
Viewer.ViewpointX: 0
Viewer.ViewpointY: -0.7
Viewer.ViewpointZ: -1.8
Viewer.ViewpointF: 500
T265.yaml
%YAML:1.0
Camera.type: "PinHole"
Camera.fx: 286.419189453125
Camera.fy: 286.384307861328
Camera.cx: 101.355010986328
Camera.cy: 102.183197021484
Camera.k1: 0.0
Camera.k2: 0.0
Camera.p1: 0.0
Camera.p2: 0.0
Camera.width: 848
Camera.height: 800
Camera.fps: 30.0
Camera.bf: 40.0
Camera.RGB: 1
ThDepth: 40.0
DepthMapFactor: 1.0
ORBextractor.nFeatures: 1000
ORBextractor.scaleFactor: 1.2
ORBextractor.nLevels: 8
ORBextractor.iniThFAST: 20
ORBextractor.minThFAST: 7
Viewer.KeyFrameSize: 0.05
Viewer.KeyFrameLineWidth: 1
Viewer.GraphLineWidth: 0.9
Viewer.PointSize: 2
Viewer.CameraSize: 0.08
Viewer.CameraLineWidth: 3
Viewer.ViewpointX: 0
Viewer.ViewpointY: -0.7
Viewer.ViewpointZ: -1.8
Viewer.ViewpointF: 500
https://dgzc.ganahe.top/ganahe/2021/wrjzzdhcgqtgrfbh.html
本文内容由网友自发贡献,版权归原作者所有,本站不承担相应法律责任。如您发现有涉嫌抄袭侵权的内容,请联系:hwhale#tublm.com(使用前将#替换为@)