整体结构图
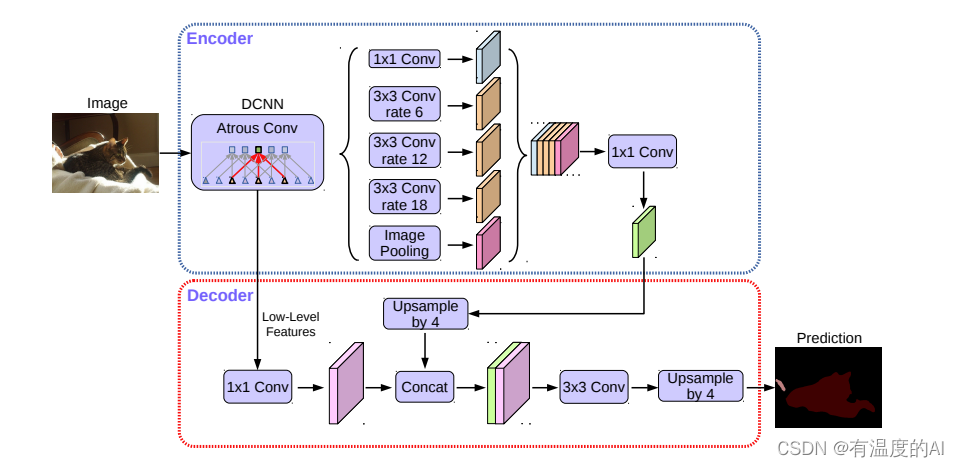
超详细结构图(Mobilenetv2主干)

主干网络搭建
import math
import os
import torch
import torch.nn as nn
import torch.utils.model_zoo as model_zoo
BatchNorm2d = nn.BatchNorm2d
def conv_bn(inp, oup, stride):
return nn.Sequential(
nn.Conv2d(inp, oup, 3, stride, 1, bias=False),
BatchNorm2d(oup),
nn.ReLU6(inplace=True)
)
def conv_1x1_bn(inp, oup):
return nn.Sequential(
nn.Conv2d(inp, oup, 1, 1, 0, bias=False),
BatchNorm2d(oup),
nn.ReLU6(inplace=True)
)
class InvertedResidual(nn.Module):
def __init__(self, inp, oup, stride, expand_ratio):
super(InvertedResidual, self).__init__()
self.stride = stride
assert stride in [1, 2]
hidden_dim = round(inp * expand_ratio)
self.use_res_connect = self.stride == 1 and inp == oup
if expand_ratio == 1:
self.conv = nn.Sequential(
#--------------------------------------------#
# 进行3x3的逐层卷积,进行跨特征点的特征提取
#--------------------------------------------#
nn.Conv2d(hidden_dim, hidden_dim, 3, stride, 1, groups=hidden_dim, bias=False),
BatchNorm2d(hidden_dim),
nn.ReLU6(inplace=True),
#-----------------------------------#
# 利用1x1卷积进行通道数的调整
#-----------------------------------#
nn.Conv2d(hidden_dim, oup, 1, 1, 0, bias=False),
BatchNorm2d(oup),
)
else:
self.conv = nn.Sequential(
#-----------------------------------#
# 利用1x1卷积进行通道数的上升
#-----------------------------------#
nn.Conv2d(inp, hidden_dim, 1, 1, 0, bias=False),
BatchNorm2d(hidden_dim),
nn.ReLU6(inplace=True),
#--------------------------------------------#
# 进行3x3的逐层卷积,进行跨特征点的特征提取
#--------------------------------------------#
nn.Conv2d(hidden_dim, hidden_dim, 3, stride, 1, groups=hidden_dim, bias=False),
BatchNorm2d(hidden_dim),
nn.ReLU6(inplace=True),
#-----------------------------------#
# 利用1x1卷积进行通道数的下降
#-----------------------------------#
nn.Conv2d(hidden_dim, oup, 1, 1, 0, bias=False),
BatchNorm2d(oup),
)
def forward(self, x):
if self.use_res_connect:
return x + self.conv(x)
else:
return self.conv(x)
class MobileNetV2(nn.Module):
def __init__(self, n_class=1000, input_size=224, width_mult=1.):
super(MobileNetV2, self).__init__()
block = InvertedResidual
input_channel = 32
last_channel = 1280
interverted_residual_setting = [
# 输入图像大小为512,512,3
# t, c, n, s
[1, 16, 1, 1], # 256, 256, 32 -> 256, 256, 16
[6, 24, 2, 2], # 256, 256, 16 -> 128, 128, 24 2
[6, 32, 3, 2], # 128, 128, 24 -> 64, 64, 32 4
[6, 64, 4, 2], # 64, 64, 32 -> 32, 32, 64 7
[6, 96, 3, 1], # 32, 32, 64 -> 32, 32, 96
[6, 160, 3, 2], # 32, 32, 96 -> 16, 16, 160 14
[6, 320, 1, 1], # 16, 16, 160 -> 16, 16, 320
]
assert input_size % 32 == 0
input_channel = int(input_channel * width_mult)
self.last_channel = int(last_channel * width_mult) if width_mult > 1.0 else last_channel
# 512, 512, 3 -> 256, 256, 32
self.features = [conv_bn(3, input_channel, 2)]
for t, c, n, s in interverted_residual_setting:
output_channel = int(c * width_mult)
for i in range(n):
if i == 0:
self.features.append(block(input_channel, output_channel, s, expand_ratio=t))
else:
self.features.append(block(input_channel, output_channel, 1, expand_ratio=t))
input_channel = output_channel
self.features.append(conv_1x1_bn(input_channel, self.last_channel))
self.features = nn.Sequential(*self.features)
self.classifier = nn.Sequential(
nn.Dropout(0.2),
nn.Linear(self.last_channel, n_class),
)
self._initialize_weights()
def forward(self, x):
x = self.features(x)
x = x.mean(3).mean(2)
x = self.classifier(x)
return x
def _initialize_weights(self):
for m in self.modules():
if isinstance(m, nn.Conv2d):
n = m.kernel_size[0] * m.kernel_size[1] * m.out_channels
m.weight.data.normal_(0, math.sqrt(2. / n))
if m.bias is not None:
m.bias.data.zero_()
elif isinstance(m, BatchNorm2d):
m.weight.data.fill_(1)
m.bias.data.zero_()
elif isinstance(m, nn.Linear):
n = m.weight.size(1)
m.weight.data.normal_(0, 0.01)
m.bias.data.zero_()
def load_url(url, model_dir='./model_data', map_location=None):
if not os.path.exists(model_dir):
os.makedirs(model_dir)
filename = url.split('/')[-1]
cached_file = os.path.join(model_dir, filename)
if os.path.exists(cached_file):
return torch.load(cached_file, map_location=map_location)
else:
return model_zoo.load_url(url,model_dir=model_dir)
def mobilenetv2(pretrained=False, **kwargs):
model = MobileNetV2(n_class=1000, **kwargs)
if pretrained:
model.load_state_dict(load_url('https://github.com/bubbliiiing/deeplabv3-plus-pytorch/releases/download/v1.0/mobilenet_v2.pth.tar'), strict=False)
return model
if __name__ == "__main__":
model = mobilenetv2()
for i, layer in enumerate(model.features):
print(i, layer)
整体网络搭建
import torch
import torch.nn as nn
import torch.nn.functional as F
from nets.mobilenetv2 import mobilenetv2
from nets.xception import xception
class MobileNetV2(nn.Module):
def __init__(self, downsample_factor=8, pretrained=True):
super(MobileNetV2, self).__init__()
from functools import partial
model = mobilenetv2(pretrained)
self.features = model.features[:-1]
self.total_idx = len(self.features)
self.down_idx = [2, 4, 7, 14]
if downsample_factor == 8:
for i in range(self.down_idx[-2], self.down_idx[-1]):
self.features[i].apply(
partial(self._nostride_dilate, dilate=2)
)
for i in range(self.down_idx[-1], self.total_idx):
self.features[i].apply(
partial(self._nostride_dilate, dilate=4)
)
elif downsample_factor == 16:
for i in range(self.down_idx[-1], self.total_idx):
self.features[i].apply(
partial(self._nostride_dilate, dilate=2)
)
def _nostride_dilate(self, m, dilate):
classname = m.__class__.__name__
if classname.find('Conv') != -1:
if m.stride == (2, 2):
m.stride = (1, 1)
if m.kernel_size == (3, 3):
m.dilation = (dilate//2, dilate//2)
m.padding = (dilate//2, dilate//2)
else:
if m.kernel_size == (3, 3):
m.dilation = (dilate, dilate)
m.padding = (dilate, dilate)
def forward(self, x):
#输出两个有效特征层
low_level_features = self.features[:4](x) #浅层有效特征层,即interverted_residual_setting中第二行的输出 128,128,24
x = self.features[4:](low_level_features) #深层有效特征层,即interverted_residual_setting中最后一行的输出 32,32,320
return low_level_features, x
#-----------------------------------------#
# ASPP特征提取模块
# 利用不同膨胀率的膨胀卷积进行特征提取
#-----------------------------------------#
class ASPP(nn.Module):
def __init__(self, dim_in, dim_out, rate=1, bn_mom=0.1):
super(ASPP, self).__init__()
self.branch1 = nn.Sequential(
nn.Conv2d(dim_in, dim_out, 1, 1, padding=0, dilation=rate, bias=True), #dilation=1即没使用膨胀卷积
nn.BatchNorm2d(dim_out, momentum=bn_mom),
nn.ReLU(inplace=True), #30,30,256
)
self.branch2 = nn.Sequential(
nn.Conv2d(dim_in, dim_out, 3, 1, padding=6*rate, dilation=6*rate, bias=True), #dilation=6的膨胀卷积
nn.BatchNorm2d(dim_out, momentum=bn_mom),
nn.ReLU(inplace=True), #30,30,256
)
self.branch3 = nn.Sequential(
nn.Conv2d(dim_in, dim_out, 3, 1, padding=12*rate, dilation=12*rate, bias=True), #dilation12的膨胀卷积
nn.BatchNorm2d(dim_out, momentum=bn_mom),
nn.ReLU(inplace=True), #30,30,256
)
self.branch4 = nn.Sequential(
nn.Conv2d(dim_in, dim_out, 3, 1, padding=18*rate, dilation=18*rate, bias=True), #dilation=18的膨胀卷积
nn.BatchNorm2d(dim_out, momentum=bn_mom),
nn.ReLU(inplace=True), #30,30,256
)
self.branch5 = nn.Sequential(
nn.AdaptiveAvgPool2d((1, 1)),
nn.Conv2d(dim_in, dim_out, 1, 1, 0, bias=True),
nn.BatchNorm2d(dim_out, momentum=bn_mom),
nn.ReLU(inplace=True)
)
# self.branch5_conv = nn.Conv2d(dim_in, dim_out, 1, 1, 0, bias=True)
# self.branch5_bn = nn.BatchNorm2d(dim_out, momentum=bn_mom)
# self.branch5_relu = nn.ReLU(inplace=True)
self.conv_cat = nn.Sequential(
nn.Conv2d(dim_out*5, dim_out, 1, 1, padding=0, bias=True),
nn.BatchNorm2d(dim_out, momentum=bn_mom),
nn.ReLU(inplace=True), #30,30,256
)
def forward(self, x):
#获取输入特征图的高宽
[b, c, row, col] = x.size()
#-----------------------------------------#
# 一共五个分支
#-----------------------------------------#
conv1x1 = self.branch1(x) #30,30,256
# print("X1.shape", conv1x1.size())
conv3x3_1 = self.branch2(x) #30,30,256
# print("X2.shape", conv3x3_1.size())
conv3x3_2 = self.branch3(x) #30,30,256
# print("X3.shape", conv3x3_2.size())
conv3x3_3 = self.branch4(x) #30,30,256
# print("X4.shape", conv3x3_3.size())
#-----------------------------------------#
# 第五个分支,全局平均池化+卷积
#-----------------------------------------#
# global_feature = torch.mean(x,2,True)
# global_feature = torch.mean(global_feature,3,True)
# global_feature = self.branch5_conv(global_feature)
# global_feature = self.branch5_bn(global_feature)
# global_feature = self.branch5_relu(global_feature)
global_feature = self.branch5(x)
# print("X5.shape", global_feature.size())
global_feature = F.interpolate(global_feature, (row, col), None, 'bilinear', True) #30,30,256
#-----------------------------------------#
# 将五个分支的内容堆叠起来
# 然后1x1卷积整合特征。
#-----------------------------------------#
feature_cat = torch.cat([conv1x1, conv3x3_1, conv3x3_2, conv3x3_3, global_feature], dim=1) #30,30,256*5
result = self.conv_cat(feature_cat) #堆叠完后利用1*1卷积对通道数进行调整,30,30,256
return result
class DeepLab(nn.Module):
def __init__(self, num_classes, backbone="mobilenet", pretrained=True, downsample_factor=16):
super(DeepLab, self).__init__()
if backbone=="xception":
#----------------------------------#
# 获得两个特征层
# 浅层特征 [128,128,256]
# 主干部分 [30,30,2048]
#----------------------------------#
self.backbone = xception(downsample_factor=downsample_factor, pretrained=pretrained)
in_channels = 2048
low_level_channels = 256
elif backbone=="mobilenet":
#----------------------------------#
# 获得两个特征层
# 浅层特征 [128,128,24]
# 主干部分 [30,30,320]
#----------------------------------#
self.backbone = MobileNetV2(downsample_factor=downsample_factor, pretrained=pretrained)
in_channels = 320
low_level_channels = 24
else:
raise ValueError('Unsupported backbone - `{}`, Use mobilenet, xception.'.format(backbone))
#-----------------------------------------#
# ASPP特征提取模块
# 利用不同膨胀率的膨胀卷积进行特征提取
#-----------------------------------------#
self.aspp = ASPP(dim_in=in_channels, dim_out=256, rate=16//downsample_factor)
#----------------------------------#
# 浅层特征边
#----------------------------------#
self.shortcut_conv = nn.Sequential(
nn.Conv2d(low_level_channels, 48, 1),
nn.BatchNorm2d(48),
nn.ReLU(inplace=True)
)
self.cat_conv = nn.Sequential(
nn.Conv2d(48+256, 256, 3, stride=1, padding=1),
nn.BatchNorm2d(256),
nn.ReLU(inplace=True),
nn.Dropout(0.5),
nn.Conv2d(256, 256, 3, stride=1, padding=1),
nn.BatchNorm2d(256),
nn.ReLU(inplace=True),
nn.Dropout(0.1),
)
self.cls_conv = nn.Conv2d(256, num_classes, 1, stride=1)
def forward(self, x): #此处传入的x为原图b,3,512,512
H, W = x.size(2), x.size(3)
#-----------------------------------------#
# 获得两个特征层
# low_level_features: 浅层特征-进行卷积处理 128,128,24
# x : 主干部分-利用ASPP结构进行加强特征提取 30,30,256
#-----------------------------------------#
low_level_features, x = self.backbone(x)
x = self.aspp(x) #aspp后的输出为
#浅层特征网络经过一个1*1卷积,128,128,24->128,128,48
low_level_features = self.shortcut_conv(low_level_features)
#-----------------------------------------#
# 将加强特征边上采样
# 与浅层特征堆叠后利用卷积进行特征提取
#-----------------------------------------#
x = F.interpolate(x, size=(low_level_features.size(2), low_level_features.size(3)), mode='bilinear', align_corners=True) #x:128,128,256
x = self.cat_conv(torch.cat((x, low_level_features), dim=1)) #128,128,256+48->128,128,256
x = self.cls_conv(x) #128,128,256->128,128,num_classes
x = F.interpolate(x, size=(H, W), mode='bilinear', align_corners=True) #512,512,num_classes
return x
# if __name__ == '__main__':
# # 测试输出尺寸使用
# aspp = ASPP(320, 256)
# input = torch.randn(2, 320, 176, 240);print('input_size:', input.size())
# out = aspp(input)
reference
【DeepLabV3源码讲解(Pytorch)】 https://www.bilibili.com/video/BV1TD4y1c7Wx?share_source=copy_web&vd_source=95705b32f23f70b32dfa1721628d5874