原始代码
import torch
import torch.nn as nn
import torch.nn.functional as F
import torchvision
from torchvision import datasets, transforms
import os, PIL, pathlib,random
device = torch.device("cuda:0" if torch.cuda.is_available() else "cpu")
data_dir = '/Users/montylee/NJUPT/Learn/Github/deeplearning/pytorch/P6/data/'
data_dir = pathlib.Path(data_dir)
data_paths = list(data_dir.glob('*'))
classNames = [str(path).split('/')[-1] for path in data_paths]
train_transforms = transforms.Compose([
transforms.Resize([224,224]),
transforms.RandomHorizontalFlip(), # 随机翻转和旋转
transforms.ToTensor(),
transforms.Normalize(mean = [0.485, 0.456, 0.406], # 均值
std = [0.229, 0.224, 0.225]) # 方差
])
total_dataset = datasets.ImageFolder(data_dir, transform=train_transforms)
total_dataset.class_to_idx
train_size = int(0.8 * len(total_dataset))
test_size = len(total_dataset) - train_size
train_dataset, test_dataset = torch.utils.data.random_split(total_dataset, [train_size, test_size])
train_dataset, test_dataset
batch_size = 32
train_loader = torch.utils.data.DataLoader(train_dataset, batch_size=batch_size, shuffle=True, num_workers=1)
test_loader = torch.utils.data.DataLoader(test_dataset, batch_size=batch_size, shuffle=True, num_workers=1)
[32]
# 构建 CNN 网络
import torch.nn.functional as F
num_classes = len(classNames)
class Model(nn.Module):
def __init__(self):
super().__init__()
# 特征提取网络
self.conv1 = nn.Conv2d(3,16,kernel_size=3)
self.bn1 = nn.BatchNorm2d(16)
self.pool1 = nn.MaxPool2d(kernel_size=2)
self.dropout = nn.Dropout(p=0.3)
self.conv2 = nn.Conv2d(16,32,kernel_size=3)
self.bn2 = nn.BatchNorm2d(32)
self.pool2 = nn.MaxPool2d(kernel_size=2)
self.dropout = nn.Dropout(p=0.3)
self.conv3 = nn.Conv2d(32,64,kernel_size=3)
self.bn3 = nn.BatchNorm2d(64)
self.pool3 = nn.MaxPool2d(kernel_size=2)
# self.dropout = nn.Dropout(p=0.3)
# self.conv4 = nn.Conv2d(128,256,kernel_size=3)
# self.pool4 = nn.MaxPool2d(kernel_size=2)
# 分类网络
self.fc1 = nn.Linear(64*26*26, 256)
self.fc2 = nn.Linear(256, num_classes)
def forward(self, x):
x = self.pool1(F.relu(self.conv1(x)))
x = self.dropout(x)
x = self.pool2(F.relu(self.conv2(x)))
x = self.dropout(x)
x = self.pool3(F.relu(self.conv3(x)))
# x = self.dropout(x)
# x = self.pool4(F.relu(self.conv4(x)))
# print(x.shape)
x = torch.flatten(x, start_dim=1)
x = F.relu(self.fc1(x))
x = self.fc2(x)
return x
loss_fn = nn.CrossEntropyLoss()
learn_rate = 1e-2
opt = torch.optim.SGD(model.parameters(), lr=learn_rate)
def train(dataloader, model, loss_fn, optimizer):
size = len(dataloader.dataset)
num_batches = len(dataloader)
train_loss, train_acc = 0 , 0
for x , y in dataloader:
x, y = x.to(device), y.to(device)
pred = model(x)
loss = loss_fn(pred, y)
optimizer.zero_grad()
loss.backward()
optimizer.step()
train_acc += (pred.argmax(1) == y).type(torch.float).sum().item()
train_loss += loss.item()
train_acc /= size
train_loss /= num_batches
return train_acc, train_loss
def test(dataloader, model, loss_fn):
size = len(dataloader.dataset)
num_batches = len(dataloader)
test_loss, test_acc = 0 , 0
with torch.no_grad():
for imgs, target in dataloader:
imgs, target = imgs.to(device), target.to(device)
target_pred = model(imgs)
loss = loss_fn(target_pred, target)
test_loss += loss.item()
test_acc += (target_pred.argmax(1) == target).type(torch.float).sum().item()
test_acc /= size
test_loss /= num_batches
return test_acc, test_loss
epochs = 10
train_loss = []
train_acc = []
test_loss = []
test_acc = []
for epoch in range(epochs):
model.train()
epoch_train_acc, epoch_train_loss = train(train_loader, model, loss_fn, opt)
model.eval()
epoch_test_acc, epoch_test_loss = test(test_loader, model, loss_fn)
train_acc.append(epoch_train_acc)
train_loss.append(epoch_train_loss)
test_acc.append(epoch_test_acc)
test_loss.append(epoch_test_loss)
template = ('Epoch:{:2d},Train_acc:{:.1f}%,Train_loss:{:.3f},Test_acc:{:.1f}%,Test_loss:{:.3f}')
print(template.format(epoch+1,epoch_train_acc*100,epoch_train_loss,epoch_test_acc*100,epoch_test_loss))
print("Done")
import matplotlib.pyplot as plt
import warnings
warnings.filterwarnings('ignore')
plt.rcParams['font.sans-serif'] = ['SimHei']
plt.rcParams['axes.unicode_minus'] = False
plt.rcParams['figure.dpi'] = 100
epochs_range = range(epochs)
plt.figure(figsize = (12,3))
plt.subplot(1,2,1)
plt.plot(epochs_range,train_acc,label='Training Accuracy')
plt.plot(epochs_range,test_acc,label='Test Accuracy')
plt.legend()
plt.title("Training and Validation Accuracy")
plt.subplot(1,2,2)
plt.plot(epochs_range,train_loss,label="Training Loss")
plt.plot(epochs_range,test_loss,label='Testing Loss')
plt.legend()
plt.title('Training and Validation Loss')
plt.show()
结果:
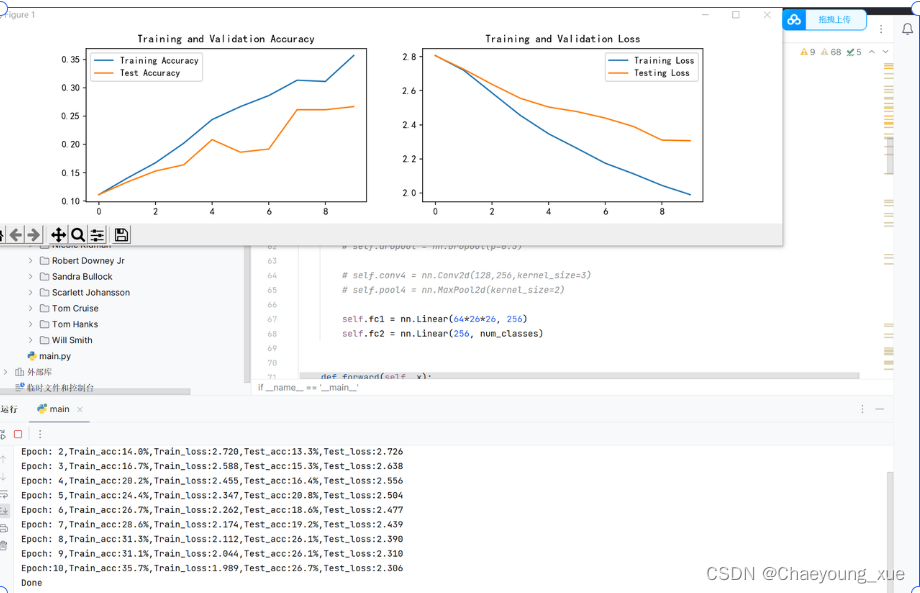

问题:
1.test_accuracy太低,小于30%,且没有收敛
2.只训练了10个epoch
解决方法
数据增强
数据集数量太少,而且将数据集分为训练集与测试集之后,数量更为稀少。
可以通过平移、旋转、缩放、裁剪、翻转、变形等,对图片进行处理,增加数据集数量
代码
(下面只对图像进行了翻转)
def data_aug(input_path, output_path, new_data_path):
imgaug(input_path, output_path)
rename_img(output_path)
merge_files(new_data_path, input_path, output_path)
def imgaug(input_path, output_path):
input_dirs = [d for d in os.listdir(input_path) if os.path.isdir(os.path.join(input_path, d))]
output_dirs = [d for d in os.listdir(output_path) if os.path.isdir(os.path.join(output_path, d))]
for i in range(len(input_dirs)):
input_dirs[i] = input_path + "\\" + input_dirs[i]
for i in range(len(output_dirs)):
output_dirs[i] = output_path + "\\" + output_dirs[i]
seq = iaa.Sequential([iaa.Flipud(1.0)])
len_class = len(input_dirs)
for i in range(len_class):
for file_name in os.listdir(input_dirs[i]):
file_path = os.path.join(input_dirs[i], file_name)
img = Image.open(file_path)
img_arr = np.array(img)
img_aug = seq(images=img_arr)
img_aug = Image.fromarray(img_aug)
if img_aug.mode == 'RGBA':
img_aug = img_aug.convert('RGB')
output_file_path = os.path.join(output_dirs[i], file_name)
img_aug.save(output_file_path)
def rename_img(folder_path):
counters = {}
for folder_name in os.listdir(folder_path):
folder = os.path.join(folder_path, folder_name)
if os.path.isdir(folder):
file_types = ['*.jpg', '*.jpeg', '*.png']
total_files = 0
for file_type in file_types:
total_files += len(glob.glob(os.path.join(folder, file_type)))
counters[folder_name] = total_files
counters = {key: value + 1 for key, value in counters.items()}
for subfolder in os.listdir(folder_path):
subfolder_path = os.path.join(folder_path, subfolder)
if os.path.isdir(subfolder_path):
counter = counters[subfolder]
for name in os.listdir(subfolder_path):
if name.endswith('.jpg') or name.endswith('.jpeg') or name.endswith('.png')
keyword = subfolder
number = counter
extension = os.path.splitext(name)[1][1:]
new_name = keyword + str(number) + '.' + extension
os.rename(os.path.join(subfolder_path, name), os.path.join(subfolder_path, new_name))
counter += 1
def merge_files(new_data_path, input_path, output_path):
new_data_dirs = [d for d in os.listdir(new_data_path) if os.path.isdir(os.path.join(new_data_path, d))]
for i in range(len(new_data_dirs)):
new_data_dirs[i] = new_data_path + "\\" + new_data_dirs[i]
len_class = len(new_data_dirs)
input_dirs = [d for d in os.listdir(input_path) if os.path.isdir(os.path.join(input_path, d))]
output_dirs = [d for d in os.listdir(output_path) if os.path.isdir(os.path.join(output_path, d))]
for i in range(len(input_dirs)):
input_dirs[i] = input_path + "\\" + input_dirs[i]
for i in range(len(output_dirs)):
output_dirs[i] = output_path + "\\" + output_dirs[i]
for i in range(len_class):
for filename in os.listdir(input_dirs[i]):
if filename.endswith(".jpg"):
src_path = os.path.join(input_dirs[i], filename)
dst_path = os.path.join(new_data_dirs[i], filename)
shutil.copyfile(src_path, dst_path)
for filename in os.listdir(output_dirs[i]):
if filename.endswith(".jpg"):
src_path = os.path.join(output_dirs[i], filename)
dst_path = os.path.join(new_data_dirs[i], filename)
shutil.copyfile(src_path, dst_path)
if name == "__main__":
input_path = r"C:\Users\STARRY\PycharmProjects\pythonProject1\data"# 原数据集路径
output_path = r"C:\Users\STARRY\PycharmProjects\pythonProject1\data2" # 反转后存放数据集的路径
new_data_path = r"C:\Users\STARRY\PycharmProjects\pythonProject1\data3" # 最终两个数据集合并后的路径
data_aug(input_path, output_path, new_data_path)
结果
epoch=10
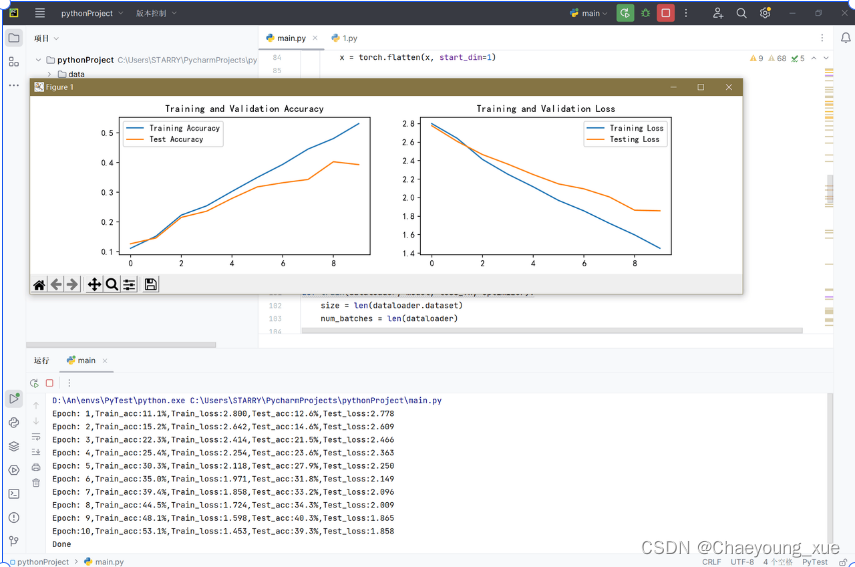
epoch=20
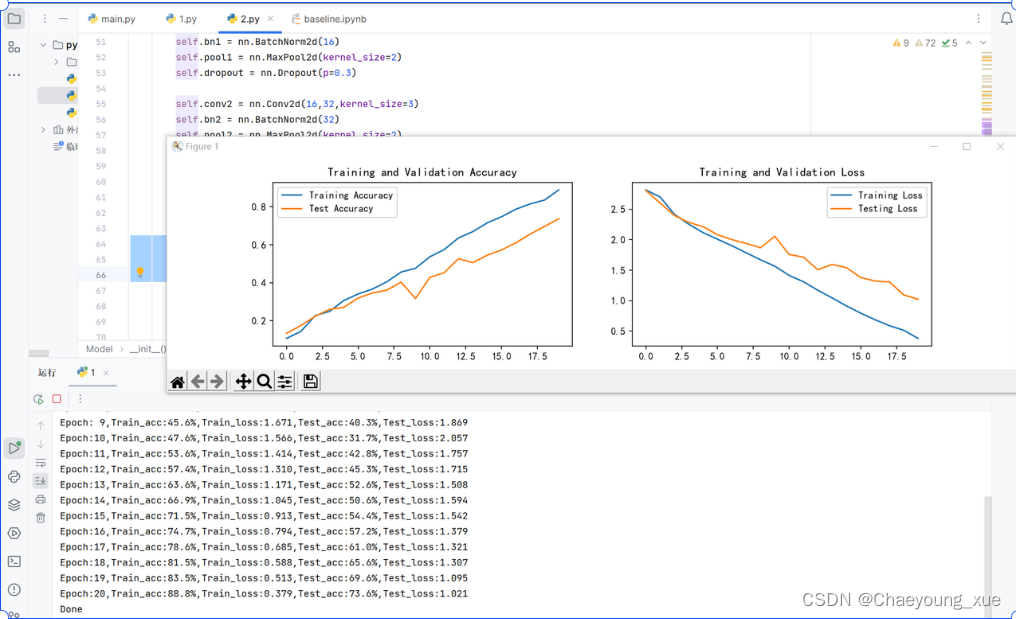
建立更好的神经网络
1.可以使用ResNet50或者VGG16网络模型来替代
2.这里我是直接在原有的网络结构基础上加了
self.dropout = nn.Dropout(p=0.3)
self.conv4 = nn.Conv2d(128,256,kernel_size=3)
self.pool4 = nn.MaxPool2d(kernel_size=2)
代码
(只有神经网络部分做了改动)
class Model(nn.Module):
def __init__(self):
super().__init__()
self.conv1 = nn.Conv2d(3,16,kernel_size=3)
self.bn1 = nn.BatchNorm2d(16)
self.pool1 = nn.MaxPool2d(kernel_size=2)
self.dropout = nn.Dropout(p=0.3)
self.conv2 = nn.Conv2d(16,32,kernel_size=3)
self.bn2 = nn.BatchNorm2d(32)
self.pool2 = nn.MaxPool2d(kernel_size=2)
self.dropout = nn.Dropout(p=0.3)
self.conv3 = nn.Conv2d(32,128,kernel_size=3)
self.bn3 = nn.BatchNorm2d(64)
self.pool3 = nn.MaxPool2d(kernel_size=2)
self.dropout = nn.Dropout(p=0.3)
self.conv4 = nn.Conv2d(128,256,kernel_size=3)
self.pool4 = nn.MaxPool2d(kernel_size=2)
self.fc1 = nn.Linear(36864, 256)
self.fc2 = nn.Linear(256, num_classes)
def forward(self, x):
x = self.pool1(F.relu(self.conv1(x)))
x = self.dropout(x)
x = self.pool2(F.relu(self.conv2(x)))
x = self.dropout(x)
x = self.pool3(F.relu(self.conv3(x)))
x = self.dropout(x)
x = self.pool4(F.relu(self.conv4(x))
x = torch.flatten(x, start_dim=1)
x = F.relu(self.fc1(x))
x = self.fc2(x)
return x
结果
epoch=20
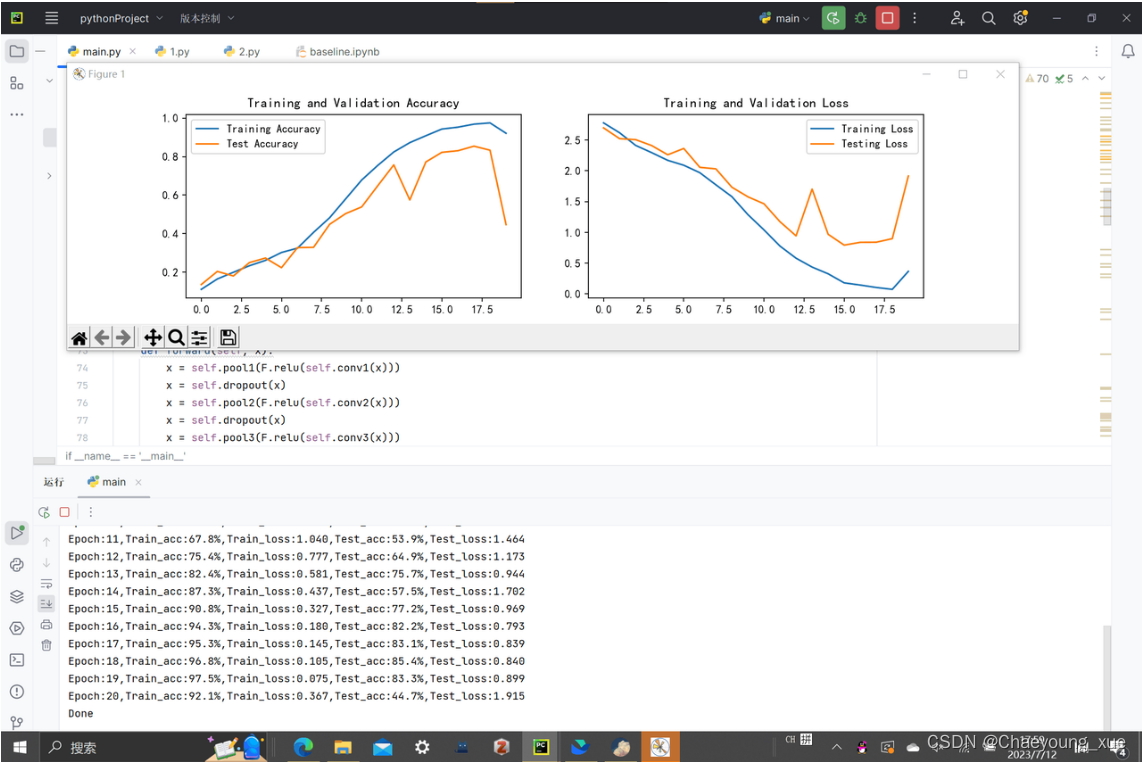
这里最高是到了85.4%
调整一些参数
可以调节优化器的学习速率以及batc_size的大小去提高准确度