1、配置Mask_RCNN ,并运行demo
1.1环境
CUDA9.0 + tensorflow_gpu1.12 + python3
1.2下载github
git clone https://github.com/matterport/Mask_RCNN
1.3安装依赖库
cd 到下载好的 Mask_RCNN 文件夹下,然后执行以下语句:
pip3 install -r requirements.txt
1.4安装Mask_RCNN
仍然在 Mask_RCNN根目录下,执行以下语句
python3 setup.py install
1.5下载coco已训练好模型
https://github.com/matterport/Mask_RCNN/releases
中的 mask_rcnn_balloon.h5
外网资源下载较慢,有可能会中断,已放到了百度云:
https://download.csdn.net/download/xjtdw/11269068
1.6安装 pycocotools
Linux: https://github.com/waleedka/coco
下载源文件后
cd coco/PythonAPI ,
make install
python3 setup.py install --user
PS:这里需要注意一下,执行make指令时,将makefile文件中的 python setup.py改为python3 setup.py,使用python3版本才可以,否则后面demo.py会报错。
将会生成 pycocotools 文件夹,复制到Mask-RCNN文件夹下
1.7使用 Jupyter 运行 demo.ipynb
在./samples/demo.ipynb,运行可见类似下图:
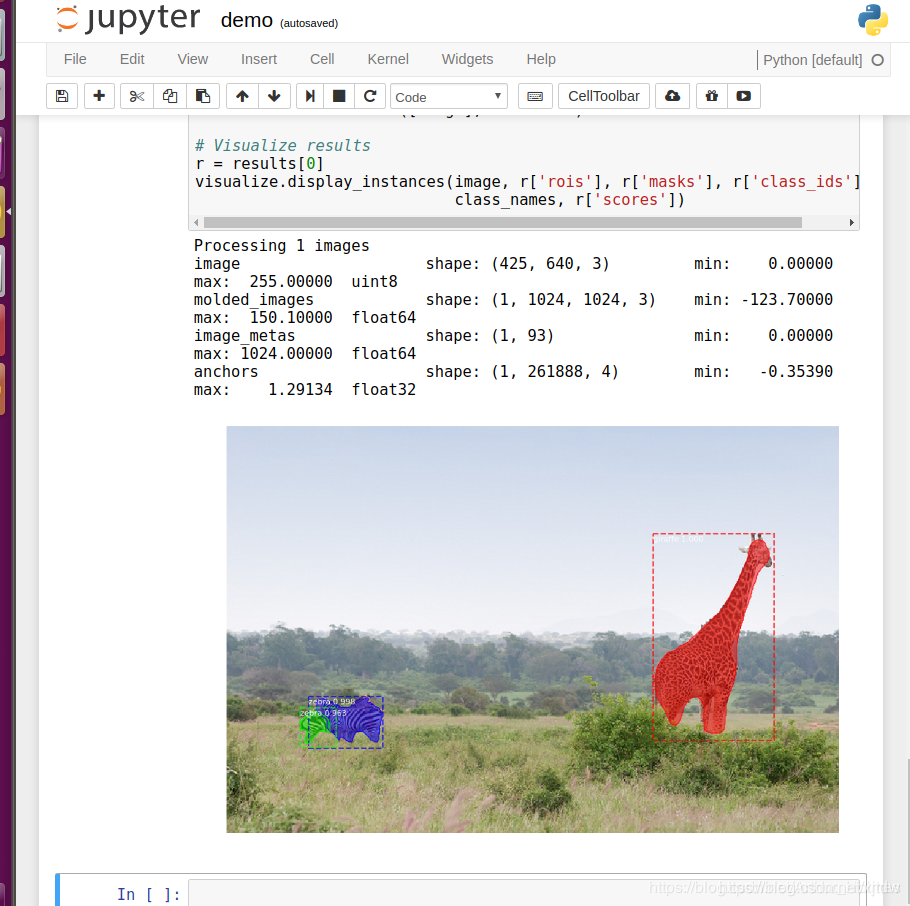
也可以将 demo.ipynb 转化为 demo.py执行
https://blog.csdn.net/qq_15192373/article/details/81673419
2、训练
2.1下载coco数据集
转:
https://blog.csdn.net/daniaokuye/article/details/78699138
https://blog.csdn.net/u014734886/article/details/78830713
https://blog.csdn.net/weixin_38293440/article/details/81196428
这几篇文章对coco数据集总结的不错,前面两篇是coco下载链接,后面一篇是对COCO数据集的介绍。
2.2训练网络-coco数据集
进入samples/coco/coco.py
#Train a new model starting from pre-trained COCO weights
python3 samples/coco/coco.py train --dataset=/path/to/coco/ --model=coco
#Train a new model starting from ImageNet weights
python3 samples/coco/coco.py train --dataset=/path/to/coco/ --model=imagenet
#Continue training a model that you had trained earlier
python3 samples/coco/coco.py train --dataset=/path/to/coco/ --model=/path/to/weights.h5
#Continue training the last model you trained. This will find
#the last trained weights in the model directory.
python3 samples/coco/coco.py train --dataset=/path/to/coco/ --model=last
cooc.py代码中 498-522行,为三种训练模型,按需选取其中一种即可,把另外两种删除。
PS:可能报错:
“It looks like you are subclassing Model
and you forgot to call super(YourClass, self).__init__()
. Always start with this line.”
解决方法:
找到 mrcnn/parallel_model.py ,修改如下代码:
lass ParallelModel(KM.Model):
def __init__(self, keras_model, gpu_count):
"""Class constructor.
keras_model: The Keras model to parallelize
gpu_count: Number of GPUs. Must be > 1
"""
super(ParallelModel, self).__init__()
self.inner_model = keras_model
self.gpu_count = gpu_count
merged_outputs = self.make_parallel()
super(ParallelModel, self).__init__(inputs=self.inner_model.inputs,
outputs=merged_outputs)
然后执行代码:
pip3 install keras==2.1.3.
我安装的keras==2.1.4就会依然报错,,,无语
2.3 训练自己数据集
参考:
http://duqingfeng.net/2018/04/16/Mask RCNN训练自己的数据集/
https://blog.csdn.net/qq_15192373/article/details/81697753
2.3.1首先要使用 Labelme 制作自己的数据集
参考:
https://blog.csdn.net/xjtdw/article/details/94741984
这是我自己整理的关于 labelme 标注工具安装使用教程。
另外我自己标注的一些猫狗图像数据,大家可做参考:
https://download.csdn.net/download/xjtdw/11329600
PS:labelme有个比较坑的地方,就是不同的版本,生成的标注文件有差别,使得maskrcnn在运行时候,报错如下错误:
Mask_RCNN del labels[0] keyerror(0)
ERROR:root:Error processing image {'mask_path': 'train_data/cv2_mask/7.png', 'height': 1857, 'width': 1280, 'yaml_path': 'train_data/labelme_json/7_json/info.yaml', 'path': 'train_data/pic/7.jpg', 'source': 'shapes', 'id': 14}
Traceback (most recent call last):
File "E:\Mask_RCNN-master-try\mrcnn\model.py", line 1710, in data_generator
use_mini_mask=config.USE_MINI_MASK)
File "E:\Mask_RCNN-master-try\mrcnn\model.py", line 1213, in load_image_gt
mask, class_ids = dataset.load_mask(image_id)
File "<ipython-input-3-e3b8787a20d8>", line 80, in load_mask
labels = self.from_yaml_get_class(image_id)
File "<ipython-input-3-e3b8787a20d8>", line 13, in from_yaml_get_class
del labels[0]
原因就在于,最新版本标注生成的 info.yaml文件,如左图所示;而旧版本的如右图所示,,,是不是很坑,,,
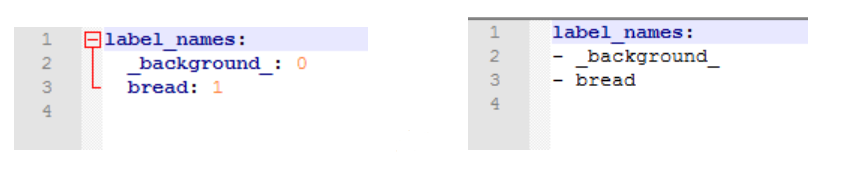
参考:https://blog.csdn.net/weixin_41685316/article/details/84204105
这样就导致标签读入错误,删除之前的labelme,安装labelme3.2,重新标注即可。
2.3.2训练
新建 train.py放在mask_rcnn/mrcnn下执行
import os
import sys
import random
import math
import re
import time
import numpy as np
import cv2
import matplotlib
import matplotlib.pyplot as plt
from config import Config
import utils
import model as modellib
import visualize
import yaml
from model import log
from PIL import Image
# Root directory of the project
ROOT_DIR = os.getcwd()
# Directory to save logs and trained model
MODEL_DIR = os.path.join(ROOT_DIR, "logs")
iter_num = 0
# Local path to trained weights file
COCO_MODEL_PATH = os.path.join(ROOT_DIR, "mask_rcnn_coco.h5")
# Download COCO trained weights from Releases if needed
if not os.path.exists(COCO_MODEL_PATH):
utils.download_trained_weights(COCO_MODEL_PATH)
class ShapesConfig(Config):
"""Configuration for training on the toy shapes dataset.
Derives from the base Config class and overrides values specific
to the toy shapes dataset.
"""
# Give the configuration a recognizable name
NAME = "shapes"
# Train on 1 GPU and 8 images per GPU. We can put multiple images on each
# GPU because the images are small. Batch size is 8 (GPUs * images/GPU).
GPU_COUNT = 1
IMAGES_PER_GPU = 1
# Number of classes (including background)
NUM_CLASSES = 1 + 2 # background + 3 shapes
# Use small images for faster training. Set the limits of the small side
# the large side, and that determines the image shape.
IMAGE_MIN_DIM = 400
IMAGE_MAX_DIM = 1600
# Use smaller anchors because our image and objects are small
RPN_ANCHOR_SCALES = (8 * 6, 16 * 6, 32 * 6, 64 * 6, 128 * 6) # anchor side in pixels
# Reduce training ROIs per image because the images are small and have
# few objects. Aim to allow ROI sampling to pick 33% positive ROIs.
TRAIN_ROIS_PER_IMAGE = 32
# Use a small epoch since the data is simple
STEPS_PER_EPOCH = 50
# use small validation steps since the epoch is small
VALIDATION_STEPS = 2
config = ShapesConfig()
config.display()
class DrugDataset(utils.Dataset):
# 得到该图中有多少个实例(物体)
def get_obj_index(self, image):
n = np.max(image)
return n
# 解析labelme中得到的yaml文件,从而得到mask每一层对应的实例标签
def from_yaml_get_class(self, image_id):
info = self.image_info[image_id]
with open(info['yaml_path']) as f:
temp = yaml.load(f.read())
labels = temp['label_names']
del labels[0]
return labels
# 重新写draw_mask
def draw_mask(self, num_obj, mask, image, image_id):
# print("draw_mask-->",image_id)
# print("self.image_info",self.image_info)
info = self.image_info[image_id]
# print("info-->",info)
# print("info[width]----->",info['width'],"-info[height]--->",info['height'])
for index in range(num_obj):
for i in range(info['width']):
for j in range(info['height']):
# print("image_id-->",image_id,"-i--->",i,"-j--->",j)
# print("info[width]----->",info['width'],"-info[height]--->",info['height'])
at_pixel = image.getpixel((i, j))
if at_pixel == index + 1:
mask[j, i, index] = 1
return mask
# 重新写load_shapes,里面包含自己的自己的类别
# 并在self.image_info信息中添加了path、mask_path 、yaml_path
# yaml_pathdataset_root_path = "/tongue_dateset/"
# img_floder = dataset_root_path + "rgb"
# mask_floder = dataset_root_path + "mask"
# dataset_root_path = "/tongue_dateset/"
def load_shapes(self, count, img_floder, mask_floder, imglist, dataset_root_path):
"""Generate the requested number of synthetic images.
count: number of images to generate.
height, width: the size of the generated images.
"""
# Add classes
self.add_class("shapes", 1, "cat")
self.add_class("shapes", 2, "dog")
for i in range(count):
# 获取图片宽和高
filestr = imglist[i].split(".")[0]
# print(imglist[i],"-->",cv_img.shape[1],"--->",cv_img.shape[0])
# print("id-->", i, " imglist[", i, "]-->", imglist[i],"filestr-->",filestr)
# filestr = filestr.split("_")[1]
mask_path = mask_floder + "/" + filestr + "_gt" + ".png"
print ('mask_path: ', mask_path)
yaml_path = dataset_root_path + "labelme_json/" + filestr + "_json/info.yaml"
# print(dataset_root_path + "labelme_json/" + filestr + "_json/img.png")
cv_img = cv2.imread(dataset_root_path + "labelme_json/" + filestr + "_json/" + filestr + ".png")
self.add_image("shapes", image_id=i, path=img_floder + "/" + imglist[i],
width=cv_img.shape[1], height=cv_img.shape[0], mask_path=mask_path, yaml_path=yaml_path)
# 重写load_mask
def load_mask(self, image_id):
"""Generate instance masks for shapes of the given image ID.
"""
global iter_num
print("image_id", image_id)
info = self.image_info[image_id]
count = 1 # number of object
img = Image.open(info['mask_path'])
num_obj = self.get_obj_index(img)
mask = np.zeros([info['height'], info['width'], num_obj], dtype=np.uint8)
mask = self.draw_mask(num_obj, mask, img, image_id)
occlusion = np.logical_not(mask[:, :, -1]).astype(np.uint8)
for i in range(count - 2, -1, -1):
mask[:, :, i] = mask[:, :, i] * occlusion
occlusion = np.logical_and(occlusion, np.logical_not(mask[:, :, i]))
labels = []
labels = self.from_yaml_get_class(image_id)
labels_form = []
for i in range(len(labels)):
if labels[i].find("cat") != -1:
# print "box"
labels_form.append("cat")
elif labels[i].find("dog") != -1:
# print "box"
labels_form.append("dog")
class_ids = np.array([self.class_names.index(s) for s in labels_form])
return mask, class_ids.astype(np.int32)
def get_ax(rows=1, cols=1, size=8):
"""Return a Matplotlib Axes array to be used in
all visualizations in the notebook. Provide a
central point to control graph sizes.
Change the default size attribute to control the size
of rendered images
"""
_, ax = plt.subplots(rows, cols, figsize=(size * cols, size * rows))
return ax
# 这里主要设置数据集的路径,不清楚的,可以下载我自己制作的数据集,一看便知:https://download.csdn.net/download/xjtdw/11329600
# 基础设置
dataset_root_path = "/media/cv/DataA/Mask_RCNN/Mask_RCNN_dataset/"
img_floder = dataset_root_path + "cat" #原图路径
mask_floder = dataset_root_path + "cv2_mask" #原图对应的标注灰度图路径
# yaml_floder = dataset_root_path
imglist = os.listdir(img_floder)
count = len(imglist)
# train与val数据集准备
dataset_train = DrugDataset()
dataset_train.load_shapes(count, img_floder, mask_floder, imglist, dataset_root_path)
dataset_train.prepare()
# print("dataset_train-->",dataset_train._image_ids)
dataset_val = DrugDataset()
dataset_val.load_shapes(3, img_floder, mask_floder, imglist, dataset_root_path)
dataset_val.prepare()
# print("dataset_val-->",dataset_val._image_ids)
# Load and display random samples
# image_ids = np.random.choice(dataset_train.image_ids, 4)
# for image_id in image_ids:
# image = dataset_train.load_image(image_id)
# mask, class_ids = dataset_train.load_mask(image_id)
# visualize.display_top_masks(image, mask, class_ids, dataset_train.class_names)
# Create model in training mode
model = modellib.MaskRCNN(mode="training", config=config,
model_dir=MODEL_DIR)
# Which weights to start with?
init_with = "coco" # imagenet, coco, or last
if init_with == "imagenet":
model.load_weights(model.get_imagenet_weights(), by_name=True)
elif init_with == "coco":
# Load weights trained on MS COCO, but skip layers that
# are different due to the different number of classes
# See README for instructions to download the COCO weights
model.load_weights(COCO_MODEL_PATH, by_name=True,
exclude=["mrcnn_class_logits", "mrcnn_bbox_fc",
"mrcnn_bbox", "mrcnn_mask"])
elif init_with == "last":
# Load the last model you trained and continue training
model.load_weights(model.find_last()[1], by_name=True)
# Train the head branches
# Passing layers="heads" freezes all layers except the head
# layers. You can also pass a regular expression to select
# which layers to train by name pattern.
model.train(dataset_train, dataset_val,
learning_rate=config.LEARNING_RATE,
epochs=6,
layers='heads')
# Fine tune all layers
# Passing layers="all" trains all layers. You can also
# pass a regular expression to select which layers to
# train by name pattern.
# model.train(dataset_train, dataset_val,
# learning_rate=config.LEARNING_RATE / 10,
# epochs=30,
# layers="all")
2.3.3测试 inference
新建 infer.py,放在 mask_rcnn/samples下执行
import os
import sys
import random
import math
import numpy as np
import skimage.io
import matplotlib
import matplotlib.pyplot as plt
import coco
from mrcnn import utils
import mrcnn.model as modellib
from mrcnn import visualize
from mrcnn.config import Config
# Root directory of the project
ROOT_DIR = os.getcwd()
# Directory to save logs and trained model
MODEL_DIR = os.path.join(ROOT_DIR, "logs")
# Local path to trained weights file
COCO_MODEL_PATH = os.path.join(ROOT_DIR, "mask_rcnn_shapes_0006.h5")
# Download COCO trained weights from Releases if needed
if not os.path.exists(COCO_MODEL_PATH):
utils.download_trained_weights(COCO_MODEL_PATH)
# Directory of images to run detection on
IMAGE_DIR = os.path.join(ROOT_DIR, "cat")
class ShapesConfig(Config):
"""Configuration for training on the toy shapes dataset.
Derives from the base Config class and overrides values specific
to the toy shapes dataset.
"""
# Give the configuration a recognizable name
NAME = "shapes"
# Train on 1 GPU and 8 images per GPU. We can put multiple images on each
# GPU because the images are small. Batch size is 8 (GPUs * images/GPU).
GPU_COUNT = 1
IMAGES_PER_GPU = 1
# Number of classes (including background)
NUM_CLASSES = 1 + 2 # background + 3 shapes
# Use small images for faster training. Set the limits of the small side
# the large side, and that determines the image shape.
IMAGE_MIN_DIM = 400
IMAGE_MAX_DIM = 1600
# Use smaller anchors because our image and objects are small
RPN_ANCHOR_SCALES = (8 * 6, 16 * 6, 32 * 6, 64 * 6, 128 * 6) # anchor side in pixels
# Reduce training ROIs per image because the images are small and have
# few objects. Aim to allow ROI sampling to pick 33% positive ROIs.
TRAIN_ROIS_PER_IMAGE = 32
# Use a small epoch since the data is simple
STEPS_PER_EPOCH = 100
# use small validation steps since the epoch is small
VALIDATION_STEPS = 5
#import train_tongue
#class InferenceConfig(coco.CocoConfig):
class InferenceConfig(ShapesConfig):
# Set batch size to 1 since we'll be running inference on
# one image at a time. Batch size = GPU_COUNT * IMAGES_PER_GPU
GPU_COUNT = 1
IMAGES_PER_GPU = 1
config = InferenceConfig()
model = modellib.MaskRCNN(mode="inference", model_dir=MODEL_DIR, config=config)
# Load weights trained on MS-COCO
# model.load_weights(COCO_MODEL_PATH, by_name=True)
model_path = model.find_last()[1]
# Load trained weights (fill in path to trained weights here)
assert model_path != "", "Provide path to trained weights"
print("Loading weights from ", model_path)
model.load_weights(COCO_MODEL_PATH, by_name=True)
class_names = ['BG', 'cat','dog']
# Load a random image from the images folder
file_names = next(os.walk(IMAGE_DIR))[2]
# image = skimage.io.imread(os.path.join(IMAGE_DIR, random.choice(file_names)))
image = skimage.io.imread('/home/cv/Downloads/4.jpg')
# Run detection
results = model.detect([image], verbose=1)
# Visualize results
r = results[0]
visualize.display_instances(image, r['rois'], r['masks'], r['class_ids'],
class_names, r['scores'])
2.3.4结果
测试结果如下:
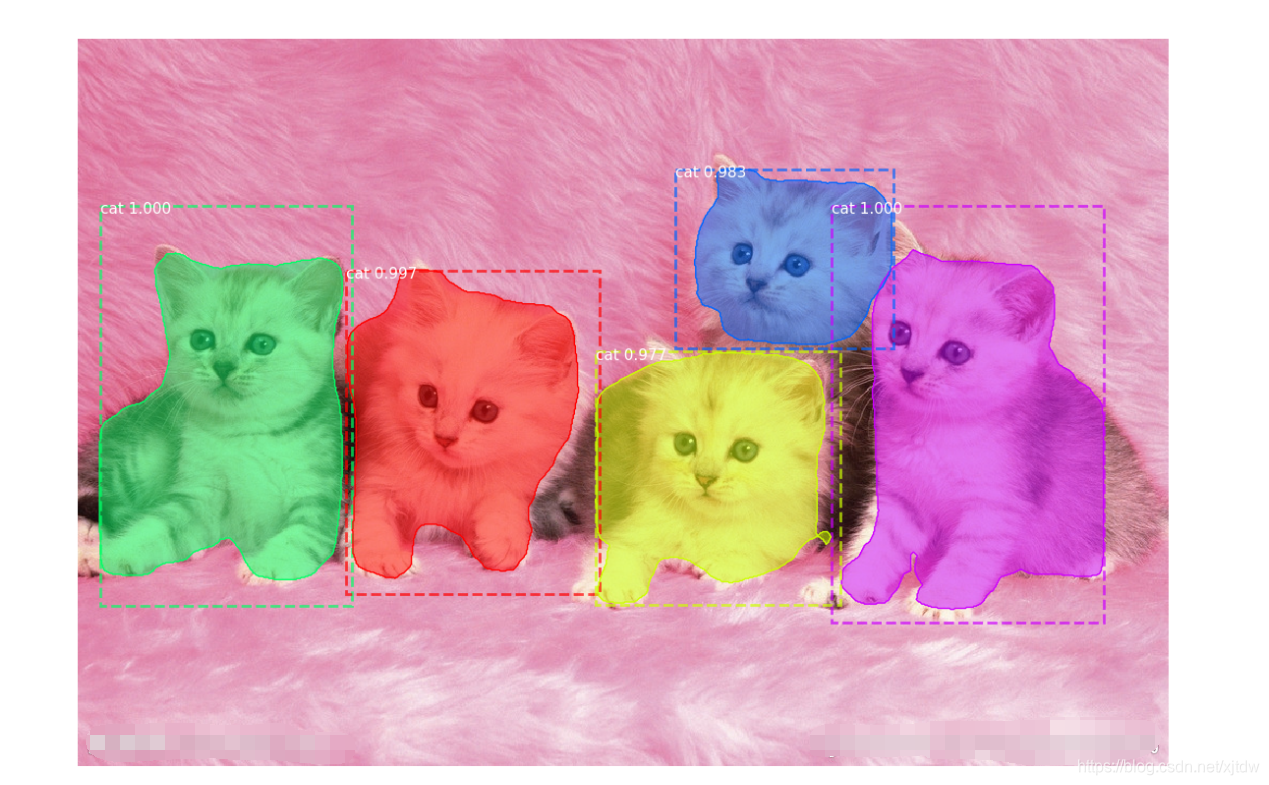