训练customdataset自定义数据集
一、修改:
1.将mmdetection/configs/yolox/yolox_s_8x8_300e_coco.py中的metric设置为mAP,如下图
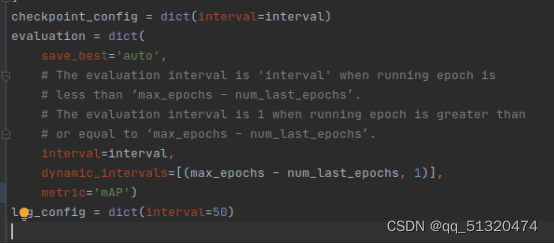
2.将mmdetection/mmdet/datasets/custom.py中的333行注释,新增334行内容,如下图。

3.修改mmdetection/configs/yolox/yolox_s_8x8_300e_coco.py中数据集以及pkl文件路径,data_root设置为自己的数据集根目录,如下图。
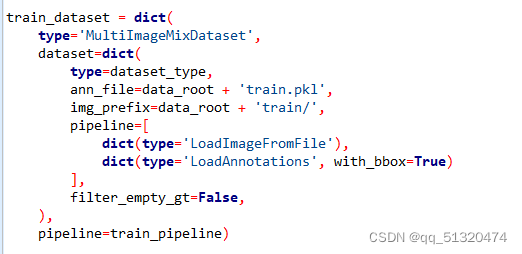
二、数据集格式转换:
将所有需要训练的图片以及xml标注文件存放入tmp文件夹中,以电线数据集为例,在tmp文件夹中存放了所以的图片以及xml文件,在与tmp文件夹同级目录下运行格式转换脚本xml_custom.py,即命令Python xml_custom.py,即可自动生成val、train、test、val.pkl、train.pkl、test.pkl文件,最后直接执行模型训练命令即可执行训练。
xml—custom转换脚本代码
# coding:utf-8
# pip install lxml
import glob
import json
import shutil
import numpy as np
import pickle
import os
import argparse
import xml.etree.ElementTree as ET
from pathlib import Path
def get(root, name):
return root.findall(name)
def get_and_check(root, name, length):
vars = root.findall(name)
if len(vars) == 0:
raise NotImplementedError('Can not find %s in %s.' % (name, root.tag))
if length > 0 and len(vars) != length:
raise NotImplementedError('The size of %s is supposed to be %d, but is %d.' % (name, length, len(vars)))
if length == 1:
vars = vars[0]
return vars
if __name__ == '__main__':
parser = argparse.ArgumentParser()
parser.add_argument('--image_xml_dir', type=str,
help='Directory of images and xml.')
parser.add_argument('--image_type', type=str, default='.png',
choices=['.jpg', '.png'], help='Type of image file.')
parser.add_argument('--output_dir', type=str,
help='Directory of output.')
a=parser.parse_args()
image_geshi = a.image_type # 设置图片的后缀名为png
origin_ann_dir = a.image_xml_dir # 设置存放所以xml和图片路径为tmp
path2 =a.output_dir
classes = []
for dirpaths, dirnames, filenames in os.walk(origin_ann_dir): # os.walk游走遍历目录名
for filename in filenames:
if filename.endswith('.xml'):
if os.path.isfile(os.path.join(origin_ann_dir, filename)): # 获取原始xml文件绝对路径,isfile()检测是否为文件 isdir检测是否为目录
origin_ann_path = os.path.join(r'%s%s' % (origin_ann_dir, filename)) # 如果是,获取绝对路径(重复代码)
# new_ann_path = os.path.join(r'%s%s' %(new_ann_dir, filename))
tree = ET.parse(origin_ann_path) # ET是一个xml文件解析库,ET.parse()打开xml文件。parse--"解析"
root = tree.getroot() # 获取根节点
for object in root.findall('object'):
xmlbox = object.find('bndbox') # 找到根节点下所有“object”节点
name = str(object.find('name').text) # 找到object节点下name子节点的值(字符串)
if name not in classes:
classes.append(name)
with open(path2 + r"/class.txt", "w") as f:
f.write('\n'.join(classes))
f.close()
START_BOUNDING_BOX_ID = 1
train_ratio = 0.7
val_ratio = 0.2
test_ratio = 0.1
xml_dir = origin_ann_dir
xml_list = glob.glob(xml_dir + "/*.xml")
xml_list = np.sort(xml_list)
np.random.seed(100)
np.random.shuffle(xml_list)
train_num = int(len(xml_list) * train_ratio)
val_num = int(len(xml_list) * val_ratio)
xml_list_train = xml_list[:train_num]
xml_list_val = xml_list[train_num:train_num + val_num]
xml_list_test = xml_list[train_num + val_num:]
f1 = open(path2 + r"/train.txt", "w")
for xml in xml_list_train:
img = xml[:-4] + image_geshi
f1.write(os.path.basename(xml)[:-4] + "\n")
f2 = open(path2+ r"/val.txt", "w")
for xml in xml_list_val:
img = xml[:-4] + image_geshi
f2.write(os.path.basename(xml)[:-4] + "\n")
f3 = open(path2 + r"/test.txt", "w")
for xml in xml_list_test:
img = xml[:-4] + image_geshi
f3.write(os.path.basename(xml)[:-4] + "\n")
f1.close()
f2.close()
f3.close()
print("-------------------------------")
print("train number:", len(xml_list_train))
print("val number:", len(xml_list_val))
print("test number:", len(xml_list_test))
with open(path2 + r"\train.txt", "r", encoding="utf-8") as f:
paths = [i.strip() for i in f.readlines()]
path3 = path2 +'/train'
if os.path.exists(path3):
shutil.rmtree(path3)
os.mkdir(path3)
else:
os.mkdir(path3)
dst_dir=path2 + "/train"
for i in paths:
img_path =xml_dir+i+image_geshi
xml_path=xml_dir+i+".xml"
shutil.copy(img_path,dst_dir+"/"+i+image_geshi)
shutil.copy(xml_path,dst_dir+"/"+i+".xml")
with open(path2 + r"/class.txt", "r", encoding="utf-8") as f:
a = [i.strip() for i in f.readlines()]
classes = a
xml_dir1 = path2 +"/train/"
xml_list = glob.glob(xml_dir1 + "/*.xml")
xml_list = np.sort(xml_list)
pre_define_categories = {}
pkl_dict = []
categories = pre_define_categories.copy()
bnd_id = START_BOUNDING_BOX_ID
all_categories = {}
number = 0
for index, line in enumerate(xml_list):
box_data = []
box_data1 = []
labels_data = []
xml_f = line
tree = ET.parse(xml_f)
root = tree.getroot()
number = number + 1
filename = os.path.basename(xml_f)[:-4] + image_geshi
image_id = 20190000001 + index
size = get_and_check(root, 'size', 1)
width = int(get_and_check(size, 'width', 1).text)
height = int(get_and_check(size, 'height', 1).text)
for obj in get(root, 'object'):
category = get_and_check(obj, 'name', 1).text
bndbox = get_and_check(obj, 'bndbox', 1)
xmin = int(float(get_and_check(bndbox, 'xmin', 1).text))
ymin = int(float(get_and_check(bndbox, 'ymin', 1).text))
xmax = int(float(get_and_check(bndbox, 'xmax', 1).text))
ymax = int(float(get_and_check(bndbox, 'ymax', 1).text))
assert (xmax > xmin), "xmax <= xmin, {}".format(line)
assert (ymax > ymin), "ymax <= ymin, {}".format(line)
o_width = abs(xmax - xmin)
o_height = abs(ymax - ymin)
box_data1 = [xmin, ymin, xmax, ymax]
box_data.append(box_data1)
name = int(obj.find('name').text)
labels_data.append(name)
image = {'filename': filename, 'width': width, 'height': height, 'ann': {'bboxes': np.array(box_data),
'labels': np.array(labels_data).T}}
pkl_dict.append(image)
print(number)
with open(path2 + r"/train.pkl","wb") as f:
pickle.dump(pkl_dict,f)
print("success-train")
# -------------------------------------
with open(path2 + r"/val.txt", "r", encoding="utf-8") as f:
paths = [i.strip() for i in f.readlines()]
path3 =path2 + '/val'
if os.path.exists(path3):
shutil.rmtree(path3)
os.mkdir(path3)
else:
os.mkdir(path3)
dst_dir=path2 + "/val"
for i in paths:
img_path =xml_dir+i+image_geshi
xml_path=xml_dir+i+".xml"
shutil.copy(img_path,dst_dir+"/"+i+image_geshi)
shutil.copy(xml_path,dst_dir+"/"+i+".xml")
with open(path2 + r"/class.txt", "r", encoding="utf-8") as f:
a = [i.strip() for i in f.readlines()]
classes = a
xml_dir2 = path2 + "/val/"
xml_list = glob.glob(xml_dir2 + "/*.xml")
xml_list = np.sort(xml_list)
pre_define_categories = {}
pkl_dict = []
categories = pre_define_categories.copy()
bnd_id = START_BOUNDING_BOX_ID
all_categories = {}
number = 0
for index, line in enumerate(xml_list):
box_data = []
box_data1 = []
labels_data = []
xml_f = line
tree = ET.parse(xml_f)
root = tree.getroot()
number = number + 1
filename = os.path.basename(xml_f)[:-4] + image_geshi
image_id = 20190000001 + index
size = get_and_check(root, 'size', 1)
width = int(get_and_check(size, 'width', 1).text)
height = int(get_and_check(size, 'height', 1).text)
for obj in get(root, 'object'):
category = get_and_check(obj, 'name', 1).text
bndbox = get_and_check(obj, 'bndbox', 1)
xmin = int(float(get_and_check(bndbox, 'xmin', 1).text))
ymin = int(float(get_and_check(bndbox, 'ymin', 1).text))
xmax = int(float(get_and_check(bndbox, 'xmax', 1).text))
ymax = int(float(get_and_check(bndbox, 'ymax', 1).text))
assert (xmax > xmin), "xmax <= xmin, {}".format(line)
assert (ymax > ymin), "ymax <= ymin, {}".format(line)
o_width = abs(xmax - xmin)
o_height = abs(ymax - ymin)
box_data1 = [xmin, ymin, xmax, ymax]
box_data.append(box_data1)
name = int(obj.find('name').text)
labels_data.append(name)
image = {'filename': filename, 'width': width, 'height': height, 'ann': {'bboxes': np.array(box_data),
'labels': np.array(labels_data).T}}
pkl_dict.append(image)
print(number)
with open(path2 + r"\val.pkl","wb") as f:
pickle.dump(pkl_dict,f)
print("success-val")
# -------------------------------------------
with open(path2 + r"\test.txt", "r", encoding="utf-8") as f:
paths = [i.strip() for i in f.readlines()]
path3 =path2 + r'/test'
if os.path.exists(path3):
shutil.rmtree(path3)
os.mkdir(path3)
else:
os.mkdir(path3)
dst_dir=path2 + "/test"
for i in paths:
img_path =xml_dir+i+image_geshi
xml_path =xml_dir +i+".xml"
shutil.copy(img_path,dst_dir+"/"+i+image_geshi)
shutil.copy(xml_path,dst_dir+"/"+i+".xml")
with open(path2 + r"/class.txt", "r", encoding="utf-8") as f:
a = [i.strip() for i in f.readlines()]
classes = a
xml_dir3 =path2 + "/test/"
xml_list = glob.glob(xml_dir3 + "/*.xml")
xml_list = np.sort(xml_list)
pre_define_categories = {}
pkl_dict = []
categories = pre_define_categories.copy()
bnd_id = START_BOUNDING_BOX_ID
all_categories = {}
number = 0
for index, line in enumerate(xml_list):
box_data = []
box_data1 = []
labels_data = []
xml_f = line
tree = ET.parse(xml_f)
root = tree.getroot()
number = number + 1
filename = os.path.basename(xml_f)[:-4] + image_geshi
image_id = 20190000001 + index
size = get_and_check(root, 'size', 1)
width = int(get_and_check(size, 'width', 1).text)
height = int(get_and_check(size, 'height', 1).text)
for obj in get(root, 'object'):
category = get_and_check(obj, 'name', 1).text
bndbox = get_and_check(obj, 'bndbox', 1)
xmin = int(float(get_and_check(bndbox, 'xmin', 1).text))
ymin = int(float(get_and_check(bndbox, 'ymin', 1).text))
xmax = int(float(get_and_check(bndbox, 'xmax', 1).text))
ymax = int(float(get_and_check(bndbox, 'ymax', 1).text))
assert (xmax > xmin), "xmax <= xmin, {}".format(line)
assert (ymax > ymin), "ymax <= ymin, {}".format(line)
o_width = abs(xmax - xmin)
o_height = abs(ymax - ymin)
box_data1 = [xmin, ymin, xmax, ymax]
box_data.append(box_data1)
name = int(obj.find('name').text)
labels_data.append(name)
image = {'filename': filename, 'width': width, 'height': height, 'ann': {'bboxes': np.array(box_data),
'labels': np.array(labels_data).T}}
pkl_dict.append(image)
print(number)
with open(path2 + r"/test.pkl","wb") as f:
pickle.dump(pkl_dict,f)
print("success-test")
yolo/xml—Coco转换脚本代码
# pylint: disable=no-member
"""
在参数列表里修改对应的参数,--image_dir是指图片存放的路径,--label_dir是指标签存放的路径
--res_dir是指标完框的图片存放的位置 --concat_dir是指所有图片拼接之后存放的路径
--num是指拼接图片的尺寸 例如num=3,拼接完的图片为3*3,
--type是指label的类型,选项有xml_cp 、 xml_lt 、 txt_cp 、 txt_lt
xml_cp是指标签文件为xml且需要将xml文件转换为txt文件,txt文件保存内容为类别、中心点坐标(x,y)以及宽w、高h,txt文件会被保存在原标签文件夹下
xml_lt是指标签文件为xml但是不需要转化txt
txt_cp是指标签文件为txt,且文件内容为类别、label中心点坐标(x,y)以及宽w、高h
txt_lt是指标签文件为txt,且文件内容为类别、label左上角和右下角坐标
此外,还需要修改dic,用来存放标注的标签数字与真实的类别一一对应的字典,例如dic{'0':'red'},表示标签0对应red
如果标注时,并没有简化成0、1、2...这样的数字,可以不修改,图片上会直接显示标注时的名称red
"""
import os
import argparse
import logging
from typing import List, Tuple, Union
from xml.dom import minidom
import imagesize
import cv2
import numpy as np
from tqdm import tqdm
class_names = []
def read_xml_bbox(xml_path_list: List[str]) -> List[List[Union[List, Tuple]]]:
"""Get bounding boxes from XML files"""
ans = []
for curr_xml_path in tqdm(xml_path_list, desc='Loading XML files'):
curr_image = []
ans.append(curr_image)
dom = minidom.parse(curr_xml_path)
for obj_node in dom.getElementsByTagName('object'):
class_name = obj_node.getElementsByTagName('name')[0].firstChild.data
bbox_node = obj_node.getElementsByTagName('bndbox')[0]
xmin, ymin, xmax, ymax = (
int(float(bbox_node.getElementsByTagName(name)[0].firstChild.data)) - 1
for name in ['xmin', 'ymin', 'xmax', 'ymax']) # x in [0, width), y in [0, height)
curr_image.append([class_name, (xmin, ymin), (xmax, ymin), (xmax, ymax), (xmin, ymax)])
return ans
def save_xml(bbox_list, save_dir, image_path_list):
"""
Save bounding boxes for all images
:param bbox_list: Bounding box list
:param save_dir: Save directory
:param image_path_list: Image path list
:return: None
"""
for bounding_boxes, image_path in tqdm(zip(bbox_list, image_path_list),
total=len(image_path_list),
desc='Saving XML files'):
doc = minidom.Document()
root_node = doc.createElement('annotation')
doc.appendChild(root_node)
create_element_with_text(doc, root_node, 'folder', os.path.split(image_path)[-2])
create_element_with_text(doc, root_node, 'filename', os.path.split(image_path)[-1])
create_element_with_text(doc, root_node, 'path', image_path)
source_node = doc.createElement('source')
create_element_with_text(doc, source_node, 'database', 'Unknown')
root_node.appendChild(source_node)
size_node = doc.createElement('size')
for element_name, value in zip(['width', 'height', 'depth'],
[*imagesize.get(image_path), 3]):
elem = doc.createElement(element_name)
elem.appendChild(doc.createTextNode(str(value)))
size_node.appendChild(elem)
root_node.appendChild(size_node)
create_element_with_text(doc, root_node, 'segmented', '0')
for bbox in bounding_boxes:
obj_node = doc.createElement('object')
create_element_with_text(doc, obj_node, 'name', get_class_name(bbox[0]))
create_element_with_text(doc, obj_node, 'pose', 'Unspecified')
create_element_with_text(doc, obj_node, 'truncated', '0')
create_element_with_text(doc, obj_node, 'difficult', '0')
bndbox_node = doc.createElement('bndbox')
for element_name, value in zip(['xmin', 'ymin', 'xmax', 'ymax'],
[min(bbox[1:], key=lambda x: x[0])[0],
min(bbox[1:], key=lambda x: x[1])[1],
max(bbox[1:], key=lambda x: x[0])[0],
max(bbox[1:], key=lambda x: x[1])[1]]):
create_element_with_text(doc, bndbox_node, element_name, str(value + 1))
obj_node.appendChild(bndbox_node)
root_node.appendChild(obj_node)
with open(os.path.join(save_dir, os.path.split(image_path)[-1][:-4] + '.xml'),
'w', encoding='utf-8') as file:
doc.writexml(file, indent='', addindent='\t', newl='\n', encoding='utf-8')
def create_element_with_text(doc, node, element_name, text):
element_node = doc.createElement(element_name)
element_node.appendChild(doc.createTextNode(text))
node.appendChild(element_node)
def read_txt_bbox(txt_path_list: List[str],
image_path_list: List[str]) -> List[List[Union[List, Tuple]]]:
"""Get bounding boxes from TXT files"""
ans = []
for i in tqdm(range(len(txt_path_list)), desc='Loading TXT files'):
curr_image = []
ans.append(curr_image)
with open(txt_path_list[i], 'r') as file:
s = file.read().strip()
# image_height, image_width = \
# cv2.imdecode(np.fromfile(image_path_list[i], dtype=np.uint8), -1).shape[:2]
image_width, image_height = imagesize.get(image_path_list[i])
for line in s.strip().split('\n'):
if not line:
continue
class_id, x, y, w, h = line.split()
class_id = int(class_id)
x, y = float(x) * image_width, float(y) * image_height
w, h = float(w) * image_width, float(h) * image_height
xmin, ymin, xmax, ymax = \
int(x - w / 2), int(y - h / 2), int(x + w / 2) - 1, int(y + h / 2) - 1
curr_image.append([class_id, (xmin, ymin), (xmax, ymin), (xmax, ymax), (xmin, ymax)])
return ans
def save_txt(bbox_list, output_label_dir, image_path_list):
for bounding_boxes, image_path in tqdm(zip(bbox_list, image_path_list),
total=len(image_path_list),
desc='Saving TXT files'):
image_width, image_height = imagesize.get(image_path)
txt_path = os.path.join(output_label_dir, os.path.split(image_path)[-1][:-4] + '.txt')
with open(txt_path, 'w') as file:
for bbox in bounding_boxes:
xmin = min(bbox[1:], key=lambda x: x[0])[0]
ymin = min(bbox[1:], key=lambda x: x[1])[1]
xmax = max(bbox[1:], key=lambda x: x[0])[0]
ymax = max(bbox[1:], key=lambda x: x[1])[1]
cx, cy = (xmin + xmax) / 2, (ymin + ymax) / 2
w, h = xmax - xmin, ymax - ymin
file.write(str(class_names.index(bbox[0])) + ' ' +
str(cx / image_width) + ' ' +
str(cy / image_height) + ' ' +
str(w / image_width) + ' ' +
str(h / image_height) + '\n')
def read_txt_rotate_bbox(txt_path_list, image_path_list):
"""Get bounding boxes from TXT files with rotation"""
ans = []
for i in tqdm(range(len(txt_path_list)), desc='Loading TXT files'):
curr_image = []
ans.append(curr_image)
with open(txt_path_list[i], 'r') as file:
s = file.read().strip()
# image_height, image_width = \
# cv2.imdecode(np.fromfile(image_path_list[i], dtype=np.uint8), -1).shape[:2]
image_width, image_height = imagesize.get(image_path_list[i])
for line in s.strip().split('\n'):
if not line:
continue
class_id, cx, cy, w, h, theta = line.split()
class_id = int(class_id)
cx, cy = float(cx) * image_width, float(cy) * image_height
w, h = float(w) * image_width, float(h) * image_height
theta = float(theta)
xmin, ymin, xmax, ymax = \
int(cx - w / 2), int(cy - h / 2), int(cx + w / 2) - 1, int(cy + h / 2) - 1
points = []
for p in [(xmin, ymin), (xmax, ymin), (xmax, ymax), (xmin, ymax)]:
p = rotated_point(p, (cx, cy), theta)
points.append((int(p[0]), int(p[1])))
curr_image.append([class_id, *points])
return ans
def save_txt_rotate(bbox_list, output_label_dir, image_path_list):
for bounding_boxes, image_path in tqdm(zip(bbox_list, image_path_list),
total=len(image_path_list),
desc='Saving TXT files'):
image_width, image_height = imagesize.get(image_path)
txt_path = os.path.join(output_label_dir, os.path.split(image_path)[-1][:-4] + '.txt')
with open(txt_path, 'w') as file:
for bbox in bounding_boxes:
rect = cv2.minAreaRect(np.array(bbox[1:]))
(cx, cy), (w, h), theta = rect
file.write(str(get_class_id(bbox[0])) + ' ' +
str(cx / image_width) + ' ' +
str(cy / image_height) + ' ' +
str(w / image_width) + ' ' +
str(h / image_height) + ' ' +
str(180 - theta) + '\n')
def rotated_point(p, q, theta):
"""Return The coordinate of point p after
rotating counterclockwise by angle theta around point q."""
# The order of the coordinate axes in the coordinate system of the image
# is opposite to that in the Cartesian coordinate system, so we need to reverse the angle.
theta = -theta
x = (p[0] - q[0]) * np.cos(np.deg2rad(theta)) - (p[1] - q[1]) * np.sin(np.deg2rad(theta)) + q[0]
y = (p[0] - q[0]) * np.sin(np.deg2rad(theta)) + (p[1] - q[1]) * np.cos(np.deg2rad(theta)) + q[1]
return x, y
def get_path_list(root_dir):
"""Return all file names in the specified directory"""
return [os.path.join(root_dir, file_name) for file_name in os.listdir(root_dir)]
def read_class_names(class_path):
global class_names
with open(class_path, 'r') as file:
class_names = file.read().strip().split('\n')
def get_class_name(class_id: Union[str, int]) -> str:
global class_names
if isinstance(class_id, str):
return class_id
return class_names[class_id]
def get_class_id(class_name: Union[str, int]) -> int:
global class_names
if isinstance(class_name, int):
return class_name
return class_names.index(class_name)
def draw_one_image(image_path, bbox_list, output_path):
"""
Draw all bounding boxes in one image.
:param image_path: Image path
:param bbox_list: Bounding box list
:param output_path: Output path to the final image
:return: None
"""
image = cv2.imread(image_path)
for bbox in bbox_list:
# draw current bounding box
for i in range(2, len(bbox)):
cv2.line(image, bbox[i - 1], bbox[i], (0, 255, 0), 2)
cv2.line(image, bbox[-1], bbox[1], (0, 255, 0), 2)
# draw class name
top_left = min(bbox[1:])
class_name = get_class_name(bbox[0])
x, y = min((top_left[0], image.shape[1] - 16 * len(class_name))), max(top_left[1], 20)
cv2.putText(image, class_name, (x, y), cv2.FONT_HERSHEY_SIMPLEX, 0.75,
(0, 255, 0), 1, cv2.LINE_AA, False)
cv2.imwrite(output_path, image)
def concat_images_into_one(image_path_list, output_path, row, col, width, height):
"""
Concatenate images into one image.
:param image_path_list: paths of input images
:param output_path: output image path
:param row: Number of images in one column of the output image
:param col: Number of images in one row of the output image
:param width: width of one column
:param height: height of one row
:return: None
"""
if row * col < len(image_path_list):
raise ValueError('Cannot concat too many images')
output_image = np.zeros((height * row, width * col, 3))
for i in range(row):
for j in range(col):
idx = i * col + j
if idx >= len(image_path_list):
cv2.imwrite(output_path, output_image)
return
image = cv2.imdecode(np.fromfile(image_path_list[idx], dtype=np.uint8), -1)
curr_image = cv2.resize(image, (width, height))
output_image[height * i:height * (i + 1), width * j:width * (j + 1)] = curr_image
cv2.imwrite(output_path, output_image)
def concat_all_images(image_path_list, output_dir, row, col, width, height):
"""
Concatenate images in groups.
:param image_path_list: paths of input images
:param output_dir: output image path
:param row: Number of images in one column of the output image
:param col: Number of images in one row of the output image
:param width: width of one column
:param height: height of one row
:return: None
"""
i = 0
count = 0
pbar = tqdm(total=len(image_path_list), desc='Concatenating')
while i + row * col - 1 < len(image_path_list):
concat_images_into_one(image_path_list[i:i + row * col],
os.path.join(output_dir, str(count) + '.png'),
row, col, width, height)
i += row * col
count += 1
pbar.update(row * col)
if i + col - 1 < len(image_path_list):
curr_row = (len(image_path_list) - i) // col
concat_images_into_one(image_path_list[i:i + curr_row * col],
os.path.join(output_dir, str(count) + '.png'),
curr_row, col, width, height)
i += curr_row * col
count += 1
pbar.update(curr_row * col)
if i < len(image_path_list):
concat_images_into_one(image_path_list[i:],
os.path.join(output_dir, str(count) + '.png'),
1, len(image_path_list) - i, width, height)
pbar.update(len(image_path_list) - i)
pbar.close()
if __name__ == '__main__':
parser = argparse.ArgumentParser()
parser.add_argument('--image_dir', type=str, default='resize_img_30_new/resize_img_30_new',
help='Directory of images.')
parser.add_argument('--image_type', type=str, default='png',
choices=['jpg', 'png'], help='Type of image file.')
parser.add_argument('--label_dir', type=str, default='xml_resize_30_2',
help='Directory of labels.')
parser.add_argument('--label_type', type=str, default='xml',
choices=['xml', 'txt', 'txt_rotate'], help='Type of input label file.')
parser.add_argument('--class_path', type=str, default='class.txt',
help='Path to the file which stores class names.')
parser.add_argument('--draw_dir', type=str, default='',
help='Output directory of drawn images.')
parser.add_argument('--concat_dir', type=str, default='',
help='Output directory of concatenated images.')
parser.add_argument('--concat_row', type=int, default=4,
help='The number of rows of the images in each concatenated image.')
parser.add_argument('--concat_col', type=int, default=4,
help='The number of columns of the images in each concatenated image.')
parser.add_argument('--concat_width', type=int, default=800,
help='The width of each image in each concatenated image.')
parser.add_argument('--concat_height', type=int, default=600,
help='The height of each image in each concatenated image.')
parser.add_argument('--output_label_type', type=str, default='txt',
choices=['xml', 'txt', 'txt_rotate'], help='Type of output label file.')
parser.add_argument('--output_label_dir', type=str, default='dx_crop_sf30_txt',
help='Directory of output label files.')
args = parser.parse_args()
# Get file path list
image_path_list = get_path_list(args.image_dir)
label_path_list = get_path_list(args.label_dir)
# Read class names
read_class_names(args.class_path)
# Filter path
image_path_list = list(filter(lambda p: p[-4:] == '.' + args.image_type, image_path_list))
if args.label_type == 'txt_rotate':
label_path_list = list(filter(lambda p: p[-4:] == '.txt', label_path_list))
else:
label_path_list = list(filter(lambda p: p[-4:] == '.' + args.label_type, label_path_list))
# Filter uncommon files
common_names = set(os.path.split(path)[-1][:-4] for path in label_path_list)
common_names.intersection_update(os.path.split(path)[-1][:-4] for path in image_path_list)
if len(common_names) < len(image_path_list) or len(common_names) < len(label_path_list):
logging.warning('Files in the label folder and the image folder are inconsistent.')
image_path_list = list(filter(lambda p: os.path.split(p)[-1][:-4] in common_names,
image_path_list))
label_path_list = list(filter(lambda p: os.path.split(p)[-1][:-4] in common_names,
label_path_list))
image_path_list.sort()
label_path_list.sort()
# Read bounding boxes
if args.label_type == 'xml':
bbox_list = read_xml_bbox(label_path_list)
elif args.label_type == 'txt':
bbox_list = read_txt_bbox(label_path_list, image_path_list)
else:
bbox_list = read_txt_rotate_bbox(label_path_list, image_path_list)
# Output labels
if args.output_label_type and args.output_label_dir:
if not os.path.exists(args.output_label_dir):
os.makedirs(args.output_label_dir)
if args.output_label_type == 'xml':
save_xml(bbox_list, args.output_label_dir, image_path_list)
elif args.output_label_type == 'txt':
save_txt(bbox_list, args.output_label_dir, image_path_list)
else:
save_txt_rotate(bbox_list, args.output_label_dir, image_path_list)
else:
logging.warning('Since the directory or type of output labels is not specified, '
'skip output labels.')
# Draw bounding boxes
if args.draw_dir:
if not os.path.exists(args.draw_dir):
os.makedirs(args.draw_dir)
for image_path, bbox in tqdm(zip(image_path_list, bbox_list),
total=len(image_path_list), desc='Drawing bounding boxes'):
draw_one_image(image_path, bbox,
os.path.join(args.draw_dir, os.path.split(image_path)[-1]))
else:
logging.warning('Since the directory of drawn images is not specified, '
'skip drawing bounding boxes.')
# Concatenate images
if args.draw_dir and args.concat_dir:
if not os.path.exists(args.concat_dir):
os.makedirs(args.concat_dir)
labeled_image_path_list = [os.path.join(args.draw_dir, name + '.' + args.image_type)
for name in common_names]
concat_all_images(labeled_image_path_list, args.concat_dir,
args.concat_row, args.concat_col, args.concat_width, args.concat_height)
else:
logging.warning('Since draw directory or concat directory is not specified, '
'skip concatenating labels.')
#coding:utf-8
# pip install lxml
import os
import glob
import json
import shutil
import numpy as np
import xml.etree.ElementTree as ET
path2 = "."
image_geshi = ".png"
START_BOUNDING_BOX_ID = 1
def get(root, name):
return root.findall(name)
def get_and_check(root, name, length):
vars = root.findall(name)
if len(vars) == 0:
raise NotImplementedError('Can not find %s in %s.'%(name, root.tag))
if length > 0 and len(vars) != length:
raise NotImplementedError('The size of %s is supposed to be %d, but is %d.'%(name, length, len(vars)))
if length == 1:
vars = vars[0]
return vars
def convert(xml_list, json_file):
json_dict = {"images": [], "type": "instances", "annotations": [], "categories": []}
categories = pre_define_categories.copy()
bnd_id = START_BOUNDING_BOX_ID
all_categories = {}
for index, line in enumerate(xml_list):
# print("Processing %s"%(line))
xml_f = line
tree = ET.parse(xml_f)
root = tree.getroot()
filename = os.path.basename(xml_f)[:-4] + image_geshi
image_id = 20190000001 + index
size = get_and_check(root, 'size', 1)
width = int(get_and_check(size, 'width', 1).text)
height = int(get_and_check(size, 'height', 1).text)
image = {'file_name': filename, 'height': height, 'width': width, 'id':image_id}
json_dict['images'].append(image)
## Cruuently we do not support segmentation
# segmented = get_and_check(root, 'segmented', 1).text
# assert segmented == '0'
for obj in get(root, 'object'):
category = get_and_check(obj, 'name', 1).text
if category in all_categories:
all_categories[category] += 1
else:
all_categories[category] = 1
if category not in categories:
if only_care_pre_define_categories:
continue
new_id = len(categories) + 1
print("[warning] category '{}' not in 'pre_define_categories'({}), create new id: {} automatically".format(category, pre_define_categories, new_id))
categories[category] = new_id
category_id = categories[category]
bndbox = get_and_check(obj, 'bndbox', 1)
xmin = int(float(get_and_check(bndbox, 'xmin', 1).text))
ymin = int(float(get_and_check(bndbox, 'ymin', 1).text))
xmax = int(float(get_and_check(bndbox, 'xmax', 1).text))
ymax = int(float(get_and_check(bndbox, 'ymax', 1).text))
assert(xmax > xmin), "xmax <= xmin, {}".format(line)
assert(ymax > ymin), "ymax <= ymin, {}".format(line)
o_width = abs(xmax - xmin)
o_height = abs(ymax - ymin)
ann = {'area': o_width*o_height, 'iscrowd': 0, 'image_id':
image_id, 'bbox':[xmin, ymin, o_width, o_height],
'category_id': category_id, 'id': bnd_id, 'ignore': 0,
'segmentation': []}
json_dict['annotations'].append(ann)
bnd_id = bnd_id + 1
for cate, cid in categories.items():
cat = {'supercategory': 'none', 'id': cid, 'name': cate}
json_dict['categories'].append(cat)
json_fp = open(json_file, 'w')
json_str = json.dumps(json_dict)
json_fp.write(json_str)
json_fp.close()
print("------------create {} done--------------".format(json_file))
print("find {} categories: {} -->>> your pre_define_categories {}: {}".format(len(all_categories), all_categories.keys(), len(pre_define_categories), pre_define_categories.keys()))
print("category: id --> {}".format(categories))
print(categories.keys())
print(categories.values())
if __name__ == '__main__':
with open(r"class.txt", "r", encoding="utf-8") as f:
a = [i.strip() for i in f.readlines()]
classes = a
pre_define_categories = {}
for i, cls in enumerate(classes):
pre_define_categories[cls] = i + 1
# pre_define_categories = {'a1': 1, 'a3': 2, 'a6': 3, 'a9': 4, "a10": 5}
only_care_pre_define_categories = True
# only_care_pre_define_categories = False
train_ratio = 0.7
val_ratio = 0.2
test_ratio = 0.1
save_json_train = 'instances_train2017.json'
save_json_val = 'instances_val2017.json'
save_json_test = 'instances_test2017.json'
xml_dir = "./dx_coco_sf60_crop_np/tmp/"
xml_list = glob.glob(xml_dir + "/*.xml")
xml_list = np.sort(xml_list)
np.random.seed(100)
np.random.shuffle(xml_list)
train_num = int(len(xml_list)*train_ratio)
val_num = int(len(xml_list) * val_ratio)
xml_list_train = xml_list[:train_num]
xml_list_val = xml_list[train_num:train_num+val_num]
xml_list_test = xml_list[train_num+val_num:]
convert(xml_list_train, save_json_train)
convert(xml_list_val, save_json_val)
convert(xml_list_test, save_json_test)
if os.path.exists(path2 + "/annotations"):
shutil.rmtree(path2 + "/annotations")
os.makedirs(path2 + "/annotations")
if os.path.exists(path2 + "/train2017"):
shutil.rmtree(path2 + "/train2017")
os.makedirs(path2 + "/train2017")
if os.path.exists(path2 + "/val2017"):
shutil.rmtree(path2 +"/val2017")
os.makedirs(path2 + "/val2017")
if os.path.exists(path2 + "/test2017"):
shutil.rmtree(path2 + "/test2017")
os.makedirs(path2 + "/test2017")
f1 = open("train.txt", "w")
for xml in xml_list_train:
img = xml[:-4] + image_geshi
f1.write(os.path.basename(xml)[:-4] + "\n")
shutil.copyfile(img, path2 + "/train2017/" + os.path.basename(img))
f2 = open("val.txt", "w")
for xml in xml_list_val:
img = xml[:-4] + image_geshi
f2.write(os.path.basename(xml)[:-4] + "\n")
shutil.copyfile(img, path2 + "/val2017/" + os.path.basename(img))
f3 = open("test.txt", "w")
for xml in xml_list_test:
img = xml[:-4] + image_geshi
f3.write(os.path.basename(xml)[:-4] + "\n")
shutil.copyfile(img, path2 + "/test2017/" + os.path.basename(img))
f1.close()
f2.close()
f3.close()
print("-------------------------------")
print("train number:", len(xml_list_train))
print("val number:", len(xml_list_val))
print("test number:", len(xml_list_test))