环境搭建可查看Python人脸识别微笑检测
数据集可在https://inc.ucsd.edu/mplab/wordpress/index.html%3Fp=398.html获取
数据如下:
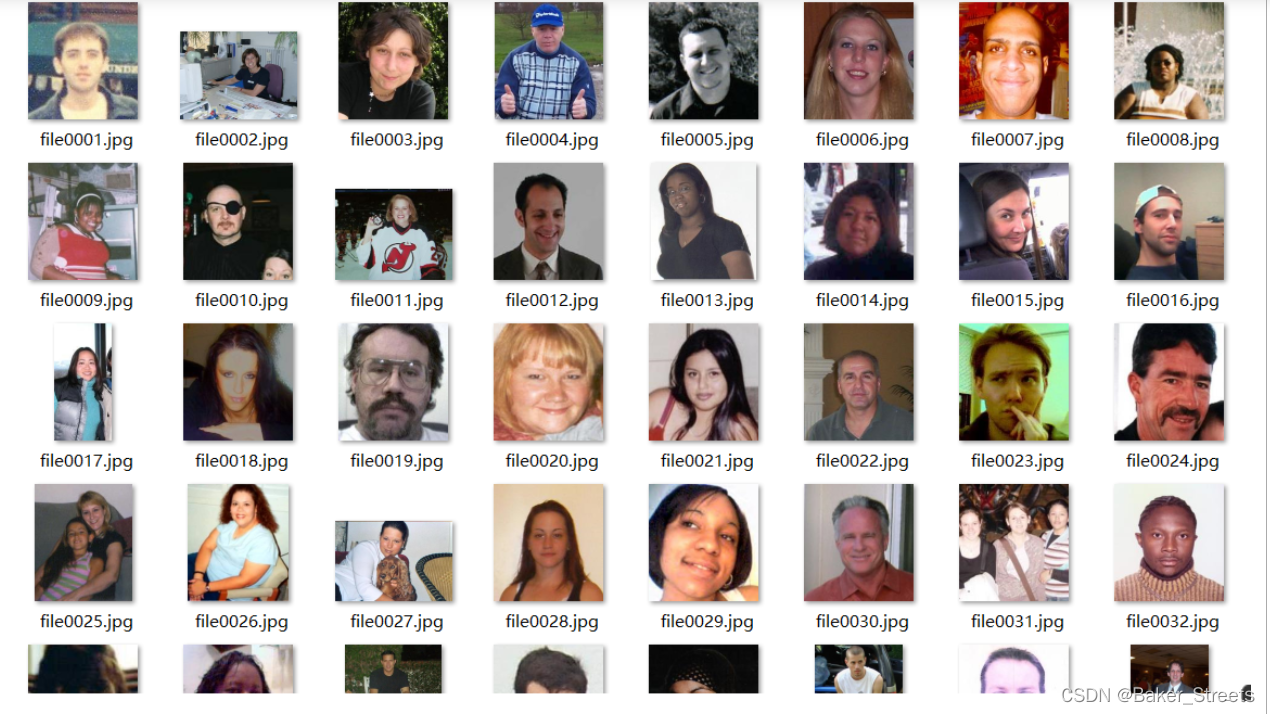
一、图片预处理
import dlib # 人脸识别的库dlib
import numpy as np # 数据处理的库numpy
import cv2 # 图像处理的库OpenCv
import os
# dlib预测器
detector = dlib.get_frontal_face_detector()
predictor = dlib.shape_predictor('shape_predictor_68_face_landmarks.dat')
# 读取图像的路径
path_read = ".\ImageFiles\\files"
num=0
for file_name in os.listdir(path_read):
#aa是图片的全路径
aa=(path_read +"/"+file_name)
#读入的图片的路径中含非英文
img=cv2.imdecode(np.fromfile(aa, dtype=np.uint8), cv2.IMREAD_UNCHANGED)
#获取图片的宽高
img_shape=img.shape
img_height=img_shape[0]
img_width=img_shape[1]
# 用来存储生成的单张人脸的路径
path_save=".\ImageFiles\\files1"
# dlib检测
dets = detector(img,1)
print("人脸数:", len(dets))
for k, d in enumerate(dets):
if len(dets)>1:
continue
num=num+1
# 计算矩形大小
# (x,y), (宽度width, 高度height)
pos_start = tuple([d.left(), d.top()])
pos_end = tuple([d.right(), d.bottom()])
# 计算矩形框大小
height = d.bottom()-d.top()
width = d.right()-d.left()
# 根据人脸大小生成空的图像
img_blank = np.zeros((height, width, 3), np.uint8)
for i in range(height):
if d.top()+i>=img_height:# 防止越界
continue
for j in range(width):
if d.left()+j>=img_width:# 防止越界
continue
img_blank[i][j] = img[d.top()+i][d.left()+j]
img_blank = cv2.resize(img_blank, (200, 200), interpolation=cv2.INTER_CUBIC)
cv2.imencode('.jpg', img_blank)[1].tofile(path_save+"\\"+"file"+str(num)+".jpg") # 正确方法
运行结果:
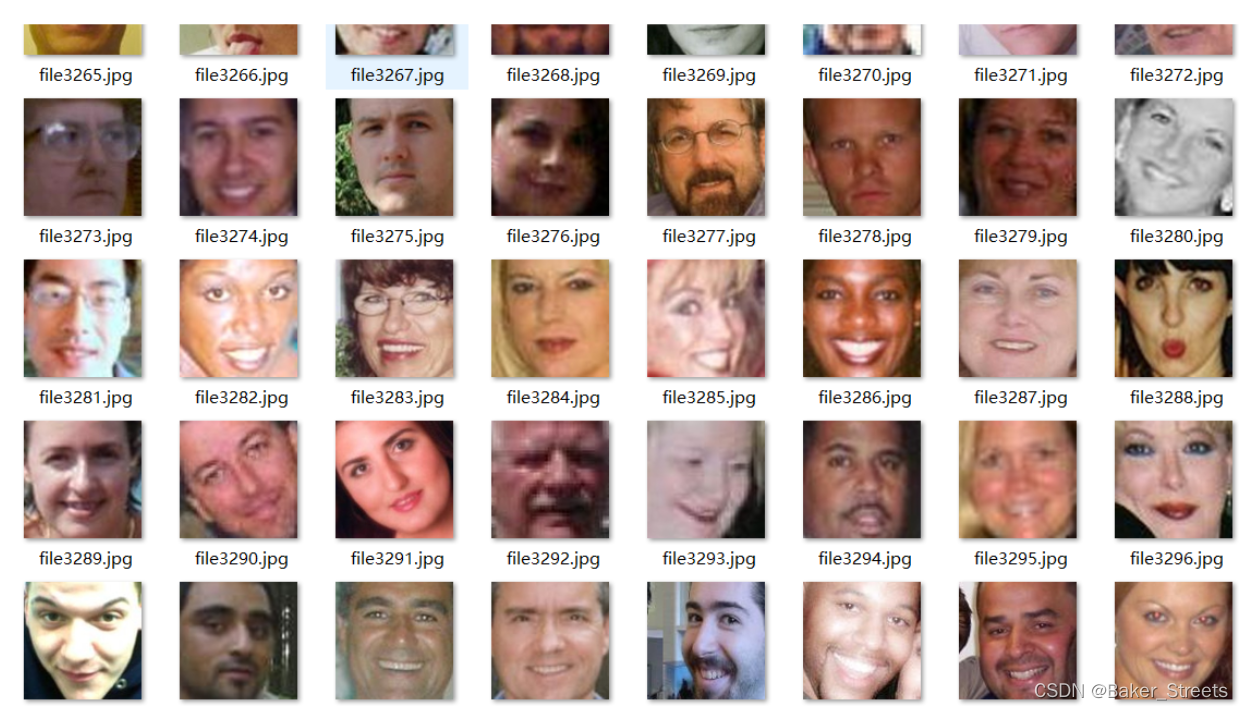
二、数据集划分
import os, shutil
# 原始数据集路径
original_dataset_dir = '.\ImageFiles\\files1'
# 新的数据集
base_dir = '.\ImageFiles\\files2'
os.mkdir(base_dir)
# 训练图像、验证图像、测试图像的目录
train_dir = os.path.join(base_dir, 'train')
os.mkdir(train_dir)
validation_dir = os.path.join(base_dir, 'validation')
os.mkdir(validation_dir)
test_dir = os.path.join(base_dir, 'test')
os.mkdir(test_dir)
train_cats_dir = os.path.join(train_dir, 'smile')
os.mkdir(train_cats_dir)
train_dogs_dir = os.path.join(train_dir, 'unsmile')
os.mkdir(train_dogs_dir)
validation_cats_dir = os.path.join(validation_dir, 'smile')
os.mkdir(validation_cats_dir)
validation_dogs_dir = os.path.join(validation_dir, 'unsmile')
os.mkdir(validation_dogs_dir)
test_cats_dir = os.path.join(test_dir, 'smile')
os.mkdir(test_cats_dir)
test_dogs_dir = os.path.join(test_dir, 'unsmile')
os.mkdir(test_dogs_dir)
# 复制1000张笑脸图片到train_c_dir
fnames = ['file{}.jpg'.format(i) for i in range(1,900)]
for fname in fnames:
src = os.path.join(original_dataset_dir, fname)
dst = os.path.join(train_cats_dir, fname)
shutil.copyfile(src, dst)
fnames = ['file{}.jpg'.format(i) for i in range(900, 1350)]
for fname in fnames:
src = os.path.join(original_dataset_dir, fname)
dst = os.path.join(validation_cats_dir, fname)
shutil.copyfile(src, dst)
# Copy next 500 cat images to test_cats_dir
fnames = ['file{}.jpg'.format(i) for i in range(1350, 1800)]
for fname in fnames:
src = os.path.join(original_dataset_dir, fname)
dst = os.path.join(test_cats_dir, fname)
shutil.copyfile(src, dst)
fnames = ['file{}.jpg'.format(i) for i in range(2127,3000)]
for fname in fnames:
src = os.path.join(original_dataset_dir, fname)
dst = os.path.join(train_dogs_dir, fname)
shutil.copyfile(src, dst)
# Copy next 500 dog images to validation_dogs_dir
fnames = ['file{}.jpg'.format(i) for i in range(3000,3304)]
for fname in fnames:
src = os.path.join(original_dataset_dir, fname)
dst = os.path.join(validation_dogs_dir, fname)
shutil.copyfile(src, dst)
# # Copy next 500 dog images to test_dogs_dir
# fnames = ['file{}.jpg'.format(i) for i in range(3000,3878)]
# for fname in fnames:
# src = os.path.join(original_dataset_dir, fname)
# dst = os.path.join(test_dogs_dir, fname)
# shutil.copyfile(src, dst)
运行结果:
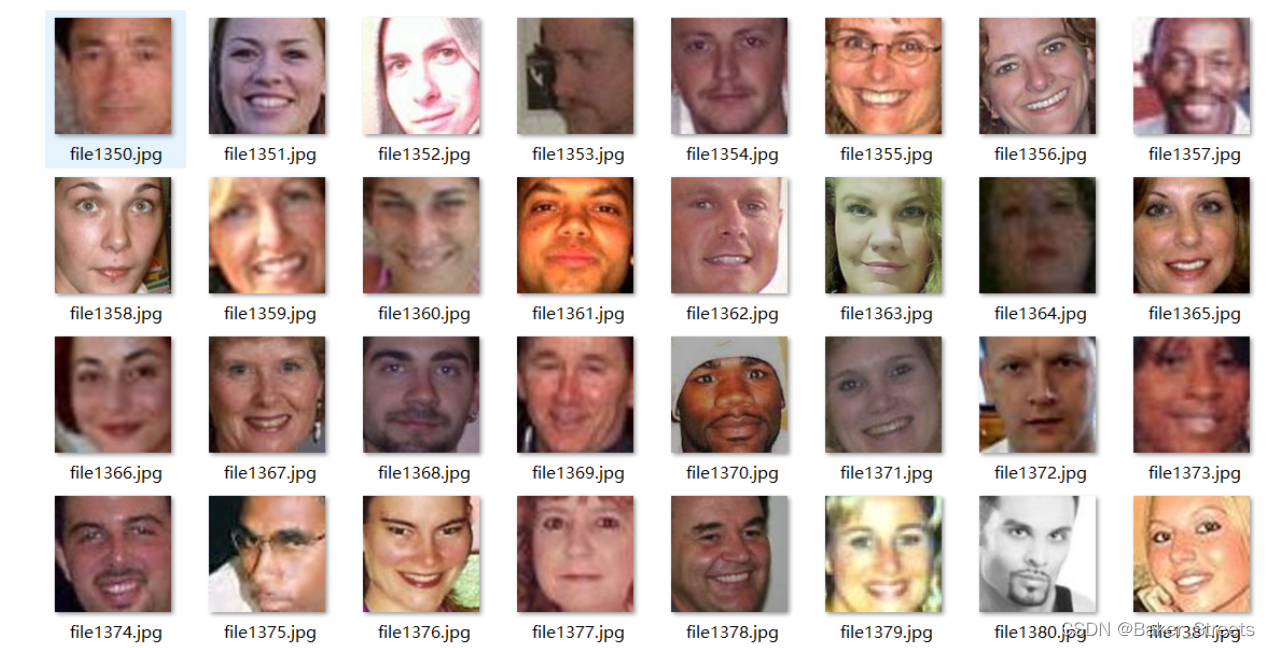
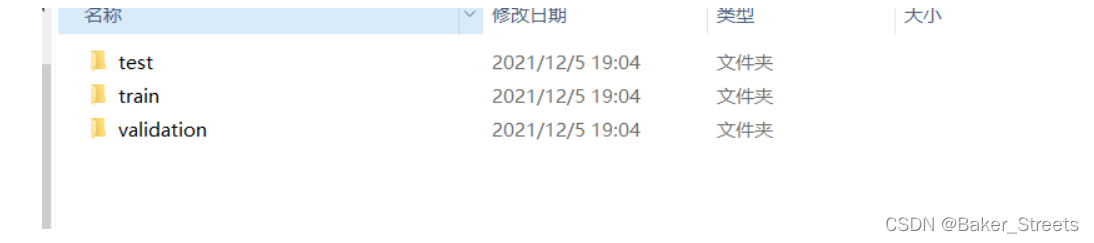
三、识别笑脸
#创建模型
from keras import layers
from keras import models
model = models.Sequential()
model.add(layers.Conv2D(32, (3, 3), activation='relu',input_shape=(150, 150, 3)))
model.add(layers.MaxPooling2D((2, 2)))
model.add(layers.Conv2D(64, (3, 3), activation='relu'))
model.add(layers.MaxPooling2D((2, 2)))
model.add(layers.Conv2D(128, (3, 3), activation='relu'))
model.add(layers.MaxPooling2D((2, 2)))
model.add(layers.Conv2D(128, (3, 3), activation='relu'))
model.add(layers.MaxPooling2D((2, 2)))
model.add(layers.Flatten())
model.add(layers.Dense(512, activation='relu'))
model.add(layers.Dense(1, activation='sigmoid'))
model.summary()#查看
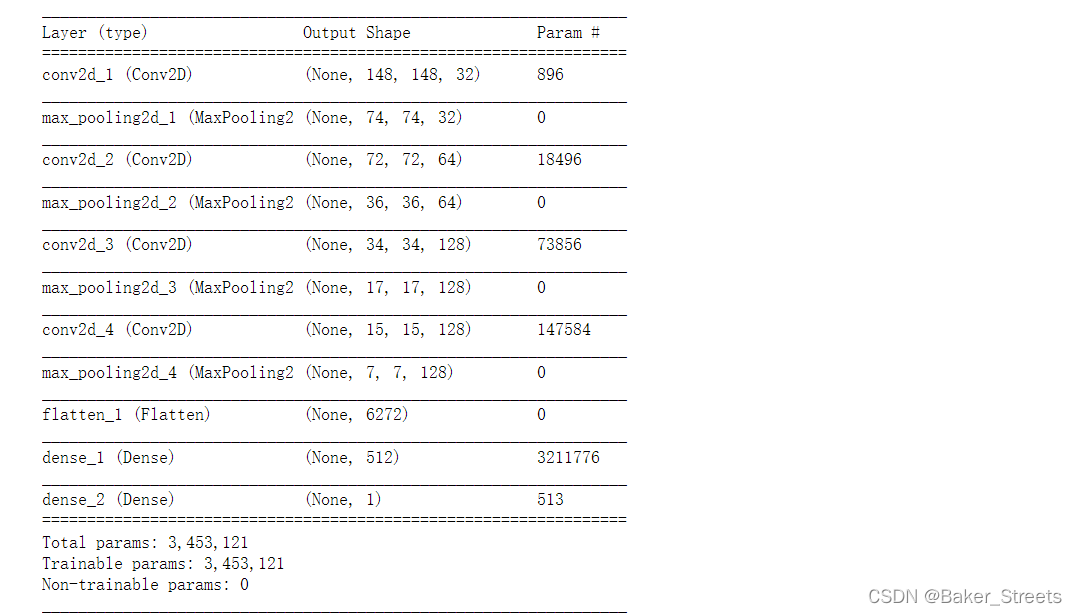
#归一化
from keras import optimizers
model.compile(loss='binary_crossentropy',
optimizer=optimizers.RMSprop(lr=1e-4),
metrics=['acc'])
from keras.preprocessing.image import ImageDataGenerator
train_datagen = ImageDataGenerator(rescale=1./255)
validation_datagen=ImageDataGenerator(rescale=1./255)
test_datagen = ImageDataGenerator(rescale=1./255)
train_generator = train_datagen.flow_from_directory(
# 目标文件目录
train_dir,
#所有图片的size必须是150x150
target_size=(150, 150),
batch_size=20,
# Since we use binary_crossentropy loss, we need binary labels
class_mode='binary')
validation_generator = test_datagen.flow_from_directory(
validation_dir,
target_size=(150, 150),
batch_size=20,
class_mode='binary')
test_generator = test_datagen.flow_from_directory(test_dir,
target_size=(150, 150),
batch_size=20,
class_mode='binary')
for data_batch, labels_batch in train_generator:
print('data batch shape:', data_batch.shape)
print('labels batch shape:', labels_batch)
break
#'smile': 0, 'unsmile': 1

#数据增强
datagen = ImageDataGenerator(
rotation_range=40,
width_shift_range=0.2,
height_shift_range=0.2,
shear_range=0.2,
zoom_range=0.2,
horizontal_flip=True,
fill_mode='nearest')
#数据增强后图片变化
import matplotlib.pyplot as plt
# This is module with image preprocessing utilities
from keras.preprocessing import image
train_smile_dir = './ImageFiles//files2//train//smile/'
fnames = [os.path.join(train_smile_dir, fname) for fname in os.listdir(train_smile_dir)]
img_path = fnames[3]
img = image.load_img(img_path, target_size=(150, 150))
x = image.img_to_array(img)
x = x.reshape((1,) + x.shape)
i = 0
for batch in datagen.flow(x, batch_size=1):
plt.figure(i)
imgplot = plt.imshow(image.array_to_img(batch[0]))
i += 1
if i % 4 == 0:
break
plt.show()
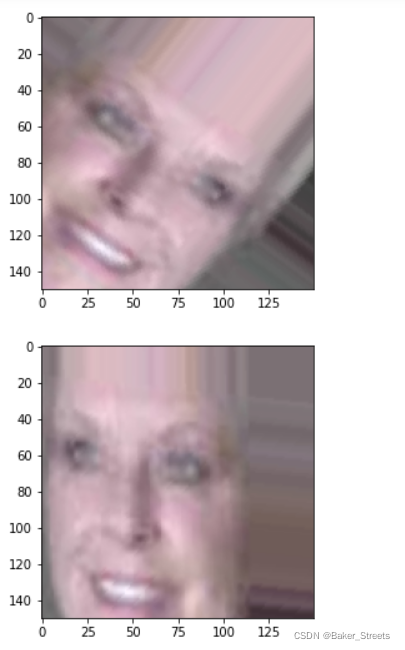
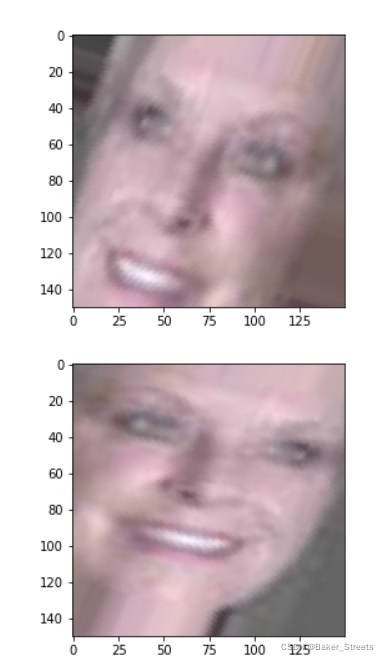
#创建网络
model = models.Sequential()
model.add(layers.Conv2D(32, (3, 3), activation='relu',input_shape=(150, 150, 3)))
model.add(layers.MaxPooling2D((2, 2)))
model.add(layers.Conv2D(64, (3, 3), activation='relu'))
model.add(layers.MaxPooling2D((2, 2)))
model.add(layers.Conv2D(128, (3, 3), activation='relu'))
model.add(layers.MaxPooling2D((2, 2)))
model.add(layers.Conv2D(128, (3, 3), activation='relu'))
model.add(layers.MaxPooling2D((2, 2)))
model.add(layers.Flatten())
model.add(layers.Dropout(0.5))
model.add(layers.Dense(512, activation='relu'))
model.add(layers.Dense(1, activation='sigmoid'))
model.compile(loss='binary_crossentropy',
optimizer=optimizers.RMSprop(lr=1e-4),
metrics=['acc'])
#归一化处理
train_datagen = ImageDataGenerator(
rescale=1./255,
rotation_range=40,
width_shift_range=0.2,
height_shift_range=0.2,
shear_range=0.2,
zoom_range=0.2,
horizontal_flip=True,)
test_datagen = ImageDataGenerator(rescale=1./255)
train_generator = train_datagen.flow_from_directory(
# This is the target directory
train_dir,
# All images will be resized to 150x150
target_size=(150, 150),
batch_size=32,
# Since we use binary_crossentropy loss, we need binary labels
class_mode='binary')
validation_generator = test_datagen.flow_from_directory(
validation_dir,
target_size=(150, 150),
batch_size=32,
class_mode='binary')
history = model.fit_generator(
train_generator,
steps_per_epoch=100,
epochs=60,
validation_data=validation_generator,
validation_steps=50)
model.save('smileAndUnsmile1.h5')
#数据增强过后的训练集与验证集的精确度与损失度的图形
acc = history.history['acc']
val_acc = history.history['val_acc']
loss = history.history['loss']
val_loss = history.history['val_loss']
epochs = range(len(acc))
plt.plot(epochs, acc, 'bo', label='Training acc')
plt.plot(epochs, val_acc, 'b', label='Validation acc')
plt.title('Training and validation accuracy')
plt.legend()
plt.figure()
plt.plot(epochs, loss, 'bo', label='Training loss')
plt.plot(epochs, val_loss, 'b', label='Validation loss')
plt.title('Training and validation loss')
plt.legend()
plt.show()
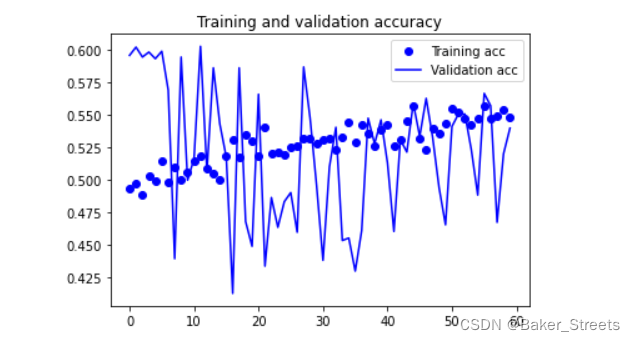
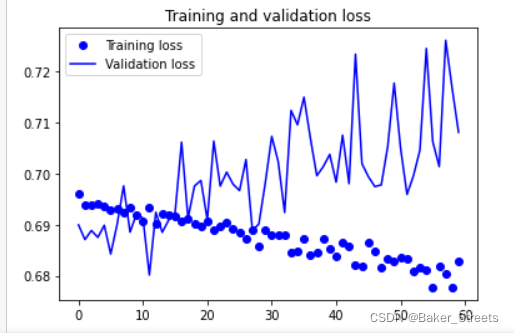
# 单张图片进行判断 是笑脸还是非笑脸
import cv2
from keras.preprocessing import image
from keras.models import load_model
import numpy as np
#加载模型
model = load_model('smileAndUnsmile1.h5')
#本地图片路径
img_path='test.jpg'
img = image.load_img(img_path, target_size=(150, 150))
img_tensor = image.img_to_array(img)/255.0
img_tensor = np.expand_dims(img_tensor, axis=0)
prediction =model.predict(img_tensor)
print(prediction)
if prediction[0][0]>0.5:
result='非笑脸'
else:
result='笑脸'
print(result)
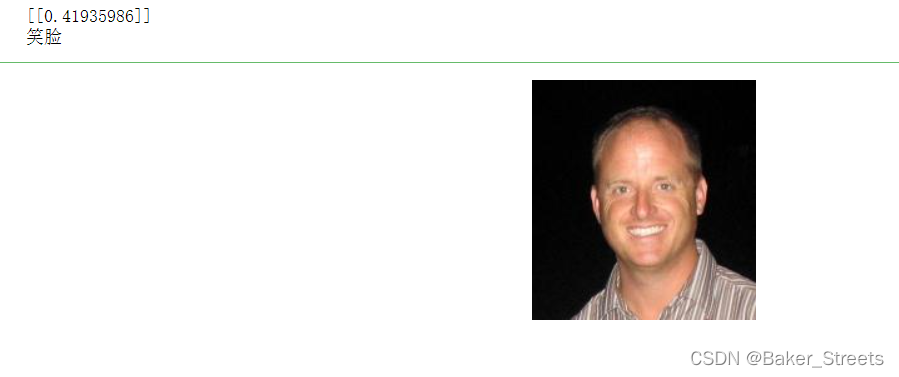
#检测视频或者摄像头中的人脸
import cv2
from keras.preprocessing import image
from keras.models import load_model
import numpy as np
import dlib
from PIL import Image
model = load_model('smileAndUnsmile1.h5')
detector = dlib.get_frontal_face_detector()
video=cv2.VideoCapture(0)
font = cv2.FONT_HERSHEY_SIMPLEX
def rec(img):
gray=cv2.cvtColor(img,cv2.COLOR_BGR2GRAY)
dets=detector(gray,1)
if dets is not None:
for face in dets:
left=face.left()
top=face.top()
right=face.right()
bottom=face.bottom()
cv2.rectangle(img,(left,top),(right,bottom),(0,255,0),2)
img1=cv2.resize(img[top:bottom,left:right],dsize=(150,150))
img1=cv2.cvtColor(img1,cv2.COLOR_BGR2RGB)
img1 = np.array(img1)/255.
img_tensor = img1.reshape(-1,150,150,3)
prediction =model.predict(img_tensor)
if prediction[0][0]>0.5:
result='unsmile'
else:
result='smile'
cv2.putText(img, result, (left,top), font, 2, (0, 255, 0), 2, cv2.LINE_AA)
cv2.imshow('Video', img)
while video.isOpened():
res, img_rd = video.read()
if not res:
break
rec(img_rd)
if cv2.waitKey(1) & 0xFF == ord('q'):
break
video.release()
cv2.destroyAllWindows()
运行结果:
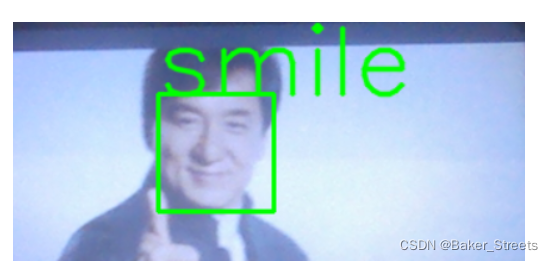
四、Dlib提取人脸特征识别笑脸和非笑脸
import cv2 # 图像处理的库 OpenCv
import dlib # 人脸识别的库 dlib
import numpy as np # 数据处理的库 numpy
class face_emotion():
def __init__(self):
self.detector = dlib.get_frontal_face_detector()
self.predictor = dlib.shape_predictor("shape_predictor_68_face_landmarks.dat")
self.cap = cv2.VideoCapture(0)
self.cap.set(3, 480)
self.cnt = 0
def learning_face(self):
line_brow_x = []
line_brow_y = []
while(self.cap.isOpened()):
flag, im_rd = self.cap.read()
k = cv2.waitKey(1)
# 取灰度
img_gray = cv2.cvtColor(im_rd, cv2.COLOR_RGB2GRAY)
faces = self.detector(img_gray, 0)
font = cv2.FONT_HERSHEY_SIMPLEX
# 如果检测到人脸
if(len(faces) != 0):
# 对每个人脸都标出68个特征点
for i in range(len(faces)):
for k, d in enumerate(faces):
cv2.rectangle(im_rd, (d.left(), d.top()), (d.right(), d.bottom()), (0,0,255))
self.face_width = d.right() - d.left()
shape = self.predictor(im_rd, d)
mouth_width = (shape.part(54).x - shape.part(48).x) / self.face_width
mouth_height = (shape.part(66).y - shape.part(62).y) / self.face_width
brow_sum = 0
frown_sum = 0
for j in range(17, 21):
brow_sum += (shape.part(j).y - d.top()) + (shape.part(j + 5).y - d.top())
frown_sum += shape.part(j + 5).x - shape.part(j).x
line_brow_x.append(shape.part(j).x)
line_brow_y.append(shape.part(j).y)
tempx = np.array(line_brow_x)
tempy = np.array(line_brow_y)
z1 = np.polyfit(tempx, tempy, 1)
self.brow_k = -round(z1[0], 3)
brow_height = (brow_sum / 10) / self.face_width # 眉毛高度占比
brow_width = (frown_sum / 5) / self.face_width # 眉毛距离占比
eye_sum = (shape.part(41).y - shape.part(37).y + shape.part(40).y - shape.part(38).y +
shape.part(47).y - shape.part(43).y + shape.part(46).y - shape.part(44).y)
eye_hight = (eye_sum / 4) / self.face_width
if round(mouth_height >= 0.03) and eye_hight<0.56:
cv2.putText(im_rd, "smile", (d.left(), d.bottom() + 20), cv2.FONT_HERSHEY_SIMPLEX, 2,
(0,255,0), 2, 4)
if round(mouth_height<0.03) and self.brow_k>-0.3:
cv2.putText(im_rd, "unsmile", (d.left(), d.bottom() + 20), cv2.FONT_HERSHEY_SIMPLEX, 2,
(0,255,0), 2, 4)
cv2.putText(im_rd, "Face-" + str(len(faces)), (20,50), font, 0.6, (0,0,255), 1, cv2.LINE_AA)
else:
cv2.putText(im_rd, "No Face", (20,50), font, 0.6, (0,0,255), 1, cv2.LINE_AA)
im_rd = cv2.putText(im_rd, "S: screenshot", (20,450), font, 0.6, (255,0,255), 1, cv2.LINE_AA)
im_rd = cv2.putText(im_rd, "Q: quit", (20,470), font, 0.6, (255,0,255), 1, cv2.LINE_AA)
if (cv2.waitKey(1) & 0xFF) == ord('s'):
self.cnt += 1
cv2.imwrite("screenshoot" + str(self.cnt) + ".jpg", im_rd)
# 按下 q 键退出
if (cv2.waitKey(1)) == ord('q'):
break
# 窗口显示
cv2.imshow("Face Recognition", im_rd)
self.cap.release()
cv2.destroyAllWindows()
if __name__ == "__main__":
my_face = face_emotion()
my_face.learning_face()
运行结果:
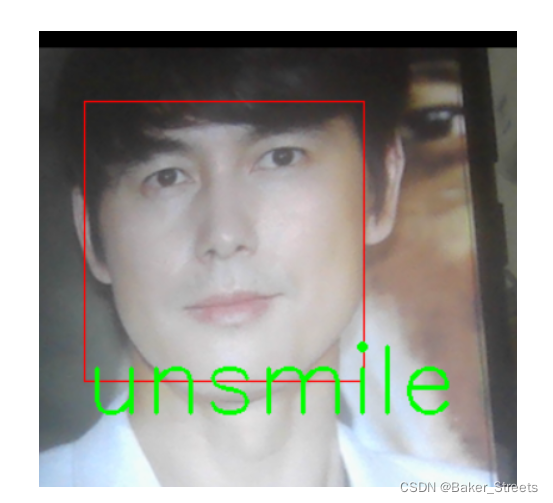
参考
Python人脸识别微笑检测
Python-人脸识别并判断表情 笑脸或非笑脸 使用笑脸数据集genki4k