文章目录
- NMS详解及pytorch实现:hard-nms(diou\overlap\merge\batched),soft-nms
- 1 简介
- 2 原理
- 3 实现
- 3.1 伪代码
- 3.2 pytorch源码
- 3.3 知识点
- 参考资料
NMS详解及pytorch实现:hard-nms(diou\overlap\merge\batched),soft-nms
1 简介
非极大值抑制算法(Non-maximum suppression, NMS)是有anchor系列目标检测的标配,如今大部分的One-Stage和Two-Stage算法在推断(Inference)阶段都使用了NMS作为网络的最后一层,例如YOLOv3、SSD、Faster-RCNN等。
当然NMS在目前最新的anchor-free(https://www.cnblogs.com/yumoye/p/11022800.html)目标检测算法中(CornerNet、CenterNet等)并不是必须的,对这种检测算法提升的精度也有限,但是这并不影响我们学习NMS。
NMS的本质是搜索局部极大值,抑制非极大值元素,在目标检测中,我们经常将其用于消除多余的检测框(从左到右消除了重复的检测框,只保留当前最大confidence的检测框):
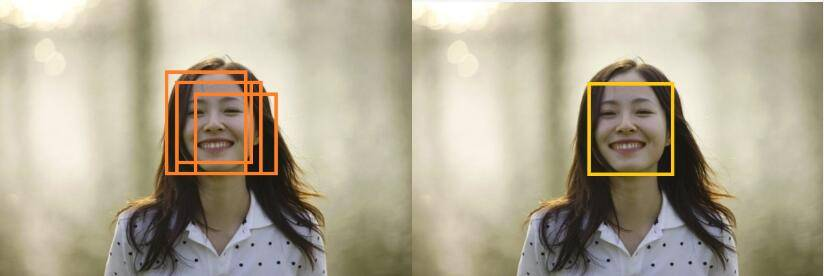
NMS有很多种变体,最为常见的Hard-NMS,我们通常所说的NMS就是指Hard-NMS,还有另外一种NMS叫做Soft-NMS,是Hard-NMS的变体,还有一些其他的一些变体(batched\diou\or\and\merge-nms)。
2 原理
最为常见的,也就是咱们提到的nms及为hard-nms,所以这里将以hard-nms入手,剖析内部操作原理。
- 选取当前类别box中scores最大的那一个,记为current_box,并保留它(为什么保留它,因为它预测出当前位置有物体的概率最大啊,对于我们来说当前confidence越大说明当前box中包含物体的可能行就越大)
- 计算current_box与其余的box的IOU
- 如果其IOU大于我们设定的阈值,那么就舍弃这些boxes(由于可能这两个box表示同一目标,因此这两个box的IOU就比较大,会超过我们设定的阈值,所以就保留分数高的那一个)
- 从最后剩余的boxes中,再找出最大scores的那一个(之前那个大的已经保存到输出的数组中,这个是从剩下的里面再挑一个最大的),如此循环往复
3 实现
3.1 伪代码
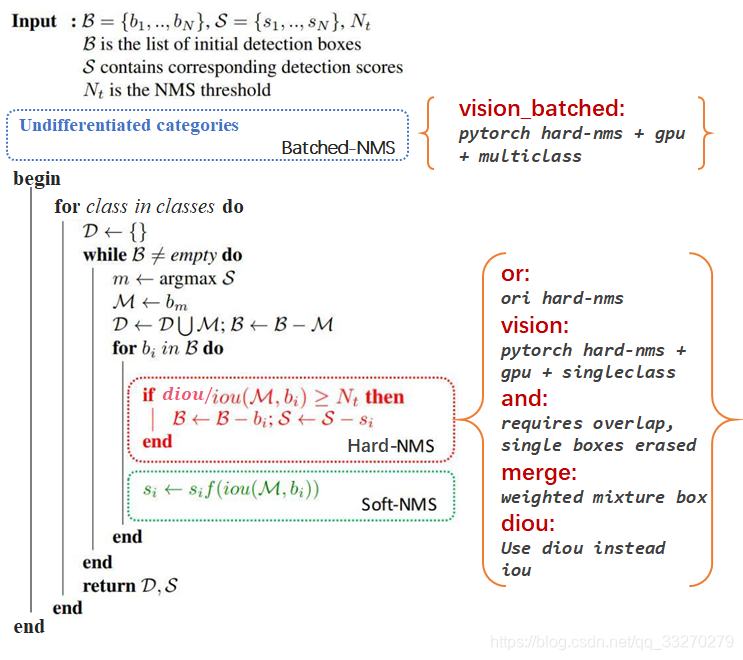
各种nms特点一句话总结:
Hard-nms–直接删除相邻的同类别目标,密集目标的输出不友好。
Soft-nms–改变其相邻同类别目标置信度(有关iou的函数),后期通过置信度阈值进行过滤,适用于目标密集的场景。
or-nms–hard-nms的非官方实现形式,只支持cpu。
vision-nms–hard-nms的官方实现形式(c函数库),可支持gpu(cuda),只支持单类别输入。
vision-batched-nms–hard-nms的官方实现形式(c函数库),可支持gpu(cuda),支持多类别输入。
and-nms–在hard-nms的逻辑基础上,增加是否为单独框的限制,删除没有重叠框的框(减少误检)。
merge-nms–在hard-nms的基础上,增加保留框位置平滑策略(重叠框位置信息求解平均值),使框的位置更加精确。
diou-nms–在hard-nms的基础上,用diou替换iou,里有参照diou的优势。
3.2 pytorch源码
nms实现函数:
def non_max_suppression(prediction, conf_thres=0.5, nms_thres=0.5, multi_cls=True, method='vision'):
"""
Removes detections with lower object confidence score than 'conf_thres'
Non-Maximum Suppression to further filter detections.
Returns detections with shape:
(x1, y1, x2, y2, object_conf, conf, class)
"""
min_wh, max_wh = 2, 4096
output = [None] * len(prediction)
for image_i, pred in enumerate(prediction):
pred = pred[pred[:, 4] > conf_thres]
pred = pred[(pred[:, 2:4] > min_wh).all(1) & (pred[:, 2:4] < max_wh).all(1)]
if len(pred) == 0:
continue
torch.sigmoid_(pred[..., 5:])
pred[..., 5:] *= pred[..., 4:5]
box = xywh2xyxy(pred[:, :4])
if multi_cls or conf_thres < 0.01:
i, j = (pred[:, 5:] > conf_thres).nonzero().t()
pred = torch.cat((box[i], pred[i, j + 5].unsqueeze(1), j.float().unsqueeze(1)), 1)
else:
conf, j = pred[:, 5:].max(1)
pred = torch.cat((box, conf.unsqueeze(1), j.float().unsqueeze(1)), 1)
pred = pred[torch.isfinite(pred).all(1)]
pred = pred[pred[:, 4].argsort(descending=True)]
if method == 'vision_batch':
output[image_i] = pred[torchvision.ops.boxes.batched_nms(pred[:, :4], pred[:, 4], pred[:, 5], nms_thres)]
continue
det_max = []
cls = pred[:, -1]
for c in cls.unique():
dc = pred[cls == c]
n = len(dc)
if n == 1:
det_max.append(dc)
continue
elif n > 500:
dc = dc[:500]
if method == 'vision':
det_max.append(dc[torchvision.ops.boxes.nms(dc[:, :4], dc[:, 4], nms_thres)])
elif method == 'or':
while dc.shape[0]:
det_max.append(dc[:1])
if len(dc) == 1:
break
iou = bbox_iou(dc[0], dc[1:])
dc = dc[1:][iou < nms_thres]
elif method == 'and':
while len(dc) > 1:
iou = bbox_iou(dc[0], dc[1:])
if iou.max() > 0.5:
det_max.append(dc[:1])
dc = dc[1:][iou < nms_thres]
elif method == 'merge':
while len(dc):
if len(dc) == 1:
det_max.append(dc)
break
i = bbox_iou(dc[0], dc) > nms_thres
weights = dc[i, 4:5]
dc[0, :4] = (weights * dc[i, :4]).sum(0) / weights.sum()
det_max.append(dc[:1])
dc = dc[i == 0]
elif method == 'diounms':
while dc.shape[0]:
det_max.append(dc[:1])
if len(dc) == 1:
break
diou = bbox_iou(dc[0], dc[1:],DIoU=True)
dc = dc[1:][diou < nms_thres]
elif method == 'soft':
sigma = 0.5
while len(dc):
if len(dc) == 1:
det_max.append(dc)
break
det_max.append(dc[:1])
iou = bbox_iou(dc[0], dc[1:])
dc = dc[1:]
dc[:, 4] *= torch.exp(-iou ** 2 / sigma)
dc = dc[dc[:, 4] > conf_thres]
if len(det_max):
det_max = torch.cat(det_max)
output[image_i] = det_max[(-det_max[:, 4]).argsort()]
return output
iou计算函数:
def bbox_iou(box1, box2, x1y1x2y2=True, GIoU=False, DIoU=False, CIoU=False):
box2 = box2.t()
if x1y1x2y2:
b1_x1, b1_y1, b1_x2, b1_y2 = box1[0], box1[1], box1[2], box1[3]
b2_x1, b2_y1, b2_x2, b2_y2 = box2[0], box2[1], box2[2], box2[3]
else:
b1_x1, b1_x2 = box1[0] - box1[2] / 2, box1[0] + box1[2] / 2
b1_y1, b1_y2 = box1[1] - box1[3] / 2, box1[1] + box1[3] / 2
b2_x1, b2_x2 = box2[0] - box2[2] / 2, box2[0] + box2[2] / 2
b2_y1, b2_y2 = box2[1] - box2[3] / 2, box2[1] + box2[3] / 2
inter = (torch.min(b1_x2, b2_x2) - torch.max(b1_x1, b2_x1)).clamp(0) * \
(torch.min(b1_y2, b2_y2) - torch.max(b1_y1, b2_y1)).clamp(0)
w1, h1 = b1_x2 - b1_x1, b1_y2 - b1_y1
w2, h2 = b2_x2 - b2_x1, b2_y2 - b2_y1
union = (w1 * h1 + 1e-16) + w2 * h2 - inter
iou = inter / union
if GIoU or DIoU or CIoU:
cw = torch.max(b1_x2, b2_x2) - torch.min(b1_x1, b2_x1)
ch = torch.max(b1_y2, b2_y2) - torch.min(b1_y1, b2_y1)
if GIoU:
c_area = cw * ch + 1e-16
return iou - (c_area - union) / c_area
if DIoU or CIoU:
c2 = cw ** 2 + ch ** 2 + 1e-16
rho2 = ((b2_x1 + b2_x2) - (b1_x1 + b1_x2)) ** 2 / 4 + ((b2_y1 + b2_y2) - (b1_y1 + b1_y2)) ** 2 / 4
if DIoU:
return iou - rho2 / c2
elif CIoU:
v = (4 / math.pi ** 2) * torch.pow(torch.atan(w2 / h2) - torch.atan(w1 / h1), 2)
with torch.no_grad():
alpha = v / (1 - iou + v)
return iou - (rho2 / c2 + v * alpha)
return iou
pytorch外接c函数库:
def nms(boxes, scores, iou_threshold):
"""
Performs non-maximum suppression (NMS) on the boxes according
to their intersection-over-union (IoU).
NMS iteratively removes lower scoring boxes which have an
IoU greater than iou_threshold with another (higher scoring)
box.
Parameters
----------
boxes : Tensor[N, 4])
boxes to perform NMS on. They
are expected to be in (x1, y1, x2, y2) format
scores : Tensor[N]
scores for each one of the boxes
iou_threshold : float
discards all overlapping
boxes with IoU < iou_threshold
Returns
-------
keep : Tensor
int64 tensor with the indices
of the elements that have been kept
by NMS, sorted in decreasing order of scores
"""
_C = _lazy_import()
return _C.nms(boxes, scores, iou_threshold)
def batched_nms(boxes, scores, idxs, iou_threshold):
"""
Performs non-maximum suppression in a batched fashion.
Each index value correspond to a category, and NMS
will not be applied between elements of different categories.
Parameters
----------
boxes : Tensor[N, 4]
boxes where NMS will be performed. They
are expected to be in (x1, y1, x2, y2) format
scores : Tensor[N]
scores for each one of the boxes
idxs : Tensor[N]
indices of the categories for each one of the boxes.
iou_threshold : float
discards all overlapping boxes
with IoU < iou_threshold
Returns
-------
keep : Tensor
int64 tensor with the indices of
the elements that have been kept by NMS, sorted
in decreasing order of scores
"""
if boxes.numel() == 0:
return torch.empty((0,), dtype=torch.int64, device=boxes.device)
max_coordinate = boxes.max()
offsets = idxs.to(boxes) * (max_coordinate + 1)
boxes_for_nms = boxes + offsets[:, None]
keep = nms(boxes_for_nms, scores, iou_threshold)
return keep
3.3 知识点
1.nms的gpu版本实现:
如有需求请参考:https://blog.csdn.net/qq_21368481/article/details/85722590
2.nms的应用范围:
只应用在前向推理的过程中,在训练中不进行此步。
3.以上几种nms的性能表现:
https://github.com/ultralytics/yolov3/issues/679
Speed mm:ss | COCO mAP @0.5…0.95 | COCO mAP @0.5 | |
---|
ultralytics 'OR' | 8:20 | 39.7 | 60.3 |
ultralytics 'AND' | 7:38 | 39.6 | 60.1 |
ultralytics 'SOFT' | 12:00 | 39.1 | 58.7 |
ultralytics 'MERGE' | 11:25 | 40.2 | 60.4 |
torchvision.ops.boxes.nms() | 5:08 | 39.7 | 60.3 |
torchvision.ops.boxes.batched_nms() | 6:00 | 39.7 | 60.3 |
参考资料
https://oldpan.me/archives/write-hard-nms-c
https://github.com/ultralytics/yolov3
本文内容由网友自发贡献,版权归原作者所有,本站不承担相应法律责任。如您发现有涉嫌抄袭侵权的内容,请联系:hwhale#tublm.com(使用前将#替换为@)