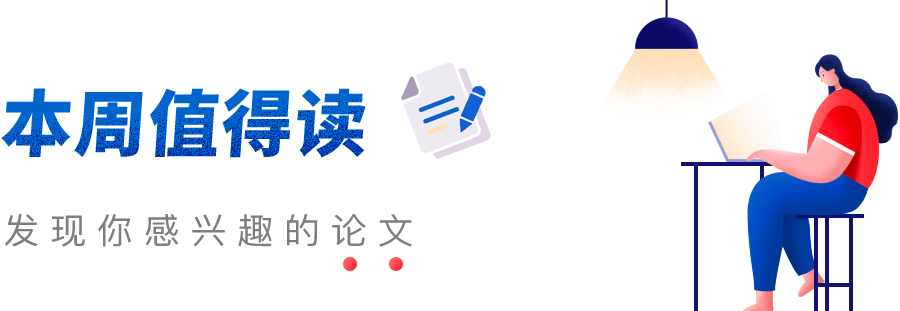
关于 #今日arXiv精选
这是「AI 学术前沿」旗下的一档栏目,编辑将每日从arXiv中精选高质量论文,推送给读者。
SUNet: Symmetric Undistortion Network for Rolling Shutter Correction
发表会议: ICCV 2021
论文地址: https://arxiv.org/abs/2108.04775
摘要
The vast majority of modern consumer-grade cameras employ a rolling shutter mechanism, leading to image distortions if the camera moves during image acquisition. In this paper, we present a novel deep network to solve the generic rolling shutter correction problem with two consecutive frames. Our pipeline is symmetrically designed to predict the global shutter image corresponding to the intermediate time of these two frames, which is difficult for existing methods because it corresponds to a camera pose that differs most from the two frames. First, two time-symmetric dense undistortion flows are estimated by using well-established principles: pyramidal construction, warping, and cost volume processing. Then, both rolling shutter images are warped into a common global shutter one in the feature space, respectively. Finally, a symmetric consistency constraint is constructed in the image decoder to effectively aggregate the contextual cues of two rolling shutter images, thereby recovering the high-quality global shutter image. Extensive experiments with both synthetic and real data from public benchmarks demonstrate the superiority of our proposed approach over the state-of-the-art methods.
Box-Aware Feature Enhancement for Single Object Tracking on Point Clouds
发表会议: ICCV 2021
论文地址: https://arxiv.org/abs/2108.04728
摘要
Current 3D single object tracking approaches track the target based on a feature comparison between the target template and the search area. However, due to the common occlusion in LiDAR scans, it is non-trivial to conduct accurate feature comparisons on severe sparse and incomplete shapes. In this work, we exploit the ground truth bounding box given in the first frame as a strong cue to enhance the feature description of the target object, enabling a more accurate feature comparison in a simple yet effective way. In particular, we first propose the BoxCloud, an informative and robust representation, to depict an object using the point-to-box relation. We further design an efficient box-aware feature fusion module, which leverages the aforementioned BoxCloud for reliable feature matching and embedding. Integrating the proposed general components into an existing model P2B, we construct a superior box-aware tracker (BAT). Experiments confirm that our proposed BAT outperforms the previous state-of-the-art by a large margin on both KITTI and NuScenes benchmarks, achieving a 12.8% improvement in terms of precision while running ~20% faster.
Multi-Camera Trajectory Forecasting with Trajectory Tensors
发表期刊: TPAMI
论文地址: https://arxiv.org/abs/2108.04694
摘要
We introduce the problem of multi-camera trajectory forecasting (MCTF), which involves predicting the trajectory of a moving object across a network of cameras. While multi-camera setups are widespread for applications such as surveillance and traffic monitoring, existing trajectory forecasting methods typically focus on single-camera trajectory forecasting (SCTF), limiting their use for such applications. Furthermore, using a single camera limits the field-of-view available, making long-term trajectory forecasting impossible. We address these shortcomings of SCTF by developing an MCTF framework that simultaneously uses all estimated relative object locations from several viewpoints and predicts the object's future location in all possible viewpoints. Our framework follows a Which-When-Where approach that predicts in which camera(s) the objects appear and when and where within the camera views they appear. To this end, we propose the concept of trajectory tensors: a new technique to encode trajectories across multiple camera views and the associated uncertainties. We develop several encoder-decoder MCTF models for trajectory tensors and present extensive experiments on our own database (comprising 600 hours of video data from 15 camera views) created particularly for the MCTF task. Results show that our trajectory tensor models outperform coordinate trajectory-based MCTF models and existing SCTF methods adapted for MCTF.
FoodLogoDet-1500: A Dataset for Large-Scale Food Logo Detection via Multi-Scale Feature Decoupling Network
发表会议: ACM MM 2021
论文地址: https://arxiv.org/abs/2108.04644
摘要
Food logo detection plays an important role in the multimedia for its wide real-world applications, such as food recommendation of the self-service shop and infringement detection on e-commerce platforms. A large-scale food logo dataset is urgently needed for developing advanced food logo detection algorithms. However, there are no available food logo datasets with food brand information. To support efforts towards food logo detection, we introduce the dataset FoodLogoDet-1500, a new large-scale publicly available food logo dataset, which has 1,500 categories, about 100,000 images and about 150,000 manually annotated food logo objects. We describe the collection and annotation process of FoodLogoDet-1500, analyze its scale and diversity, and compare it with other logo datasets. To the best of our knowledge, FoodLogoDet-1500 is the first largest publicly available high-quality dataset for food logo detection. The challenge of food logo detection lies in the large-scale categories and similarities between food logo categories. For that, we propose a novel food logo detection method Multi-scale Feature Decoupling Network (MFDNet), which decouples classification and regression into two branches and focuses on the classification branch to solve the problem of distinguishing multiple food logo categories. Specifically, we introduce the feature offset module, which utilizes the deformation-learning for optimal classification offset and can effectively obtain the most representative features of classification in detection. In addition, we adopt a balanced feature pyramid in MFDNet, which pays attention to global information, balances the multi-scale feature maps, and enhances feature extraction capability. Comprehensive experiments on FoodLogoDet-1500 and other two benchmark logo datasets demonstrate the effectiveness of the proposed method.
Learning Canonical 3D Object Representation for Fine-Grained Recognition
发表会议: ICCV 2021
论文地址: https://arxiv.org/abs/2108.04628
摘要
We propose a novel framework for fine-grained object recognition that learns to recover object variation in 3D space from a single image, trained on an image collection without using any ground-truth 3D annotation. We accomplish this by representing an object as a composition of 3D shape and its appearance, while eliminating the effect of camera viewpoint, in a canonical configuration. Unlike conventional methods modeling spatial variation in 2D images only, our method is capable of reconfiguring the appearance feature in a canonical 3D space, thus enabling the subsequent object classifier to be invariant under 3D geometric variation. Our representation also allows us to go beyond existing methods, by incorporating 3D shape variation as an additional cue for object recognition. To learn the model without ground-truth 3D annotation, we deploy a differentiable renderer in an analysis-by-synthesis framework. By incorporating 3D shape and appearance jointly in a deep representation, our method learns the discriminative representation of the object and achieves competitive performance on fine-grained image recognition and vehicle re-identification. We also demonstrate that the performance of 3D shape reconstruction is improved by learning fine-grained shape deformation in a boosting manner.
Relation-aware Compositional Zero-shot Learning for Attribute-Object Pair Recognition
发表期刊: IEEE Transactions on Multimedia
论文地址: https://arxiv.org/abs/2108.04603
摘要
This paper proposes a novel model for recognizing images with composite attribute-object concepts, notably for composite concepts that are unseen during model training. We aim to explore the three key properties required by the task --- relation-aware, consistent, and decoupled --- to learn rich and robust features for primitive concepts that compose attribute-object pairs. To this end, we propose the Blocked Message Passing Network (BMP-Net). The model consists of two modules. The concept module generates semantically meaningful features for primitive concepts, whereas the visual module extracts visual features for attributes and objects from input images. A message passing mechanism is used in the concept module to capture the relations between primitive concepts. Furthermore, to prevent the model from being biased towards seen composite concepts and reduce the entanglement between attributes and objects, we propose a blocking mechanism that equalizes the information available to the model for both seen and unseen concepts. Extensive experiments and ablation studies on two benchmarks show the efficacy of the proposed model.
Deep Metric Learning for Open World Semantic Segmentation
发表会议: ICCV 2021
论文地址: https://arxiv.org/abs/2108.04562
摘要
Classical close-set semantic segmentation networks have limited ability to detect out-of-distribution (OOD) objects, which is important for safety-critical applications such as autonomous driving. Incrementally learning these OOD objects with few annotations is an ideal way to enlarge the knowledge base of the deep learning models. In this paper, we propose an open world semantic segmentation system that includes two modules: (1) an open-set semantic segmentation module to detect both in-distribution and OOD objects. (2) an incremental few-shot learning module to gradually incorporate those OOD objects into its existing knowledge base. This open world semantic segmentation system behaves like a human being, which is able to identify OOD objects and gradually learn them with corresponding supervision. We adopt the Deep Metric Learning Network (DMLNet) with contrastive clustering to implement open-set semantic segmentation. Compared to other open-set semantic segmentation methods, our DMLNet achieves state-of-the-art performance on three challenging open-set semantic segmentation datasets without using additional data or generative models. On this basis, two incremental few-shot learning methods are further proposed to progressively improve the DMLNet with the annotations of OOD objects.
Learning Multi-Granular Spatio-Temporal Graph Network for Skeleton-based Action Recognition
发表会议: ACM MM 2021
论文地址: https://arxiv.org/abs/2108.04536
摘要
The task of skeleton-based action recognition remains a core challenge in human-centred scene understanding due to the multiple granularities and large variation in human motion. Existing approaches typically employ a single neural representation for different motion patterns, which has difficulty in capturing fine-grained action classes given limited training data. To address the aforementioned problems, we propose a novel multi-granular spatio-temporal graph network for skeleton-based action classification that jointly models the coarse- and fine-grained skeleton motion patterns. To this end, we develop a dual-head graph network consisting of two interleaved branches, which enables us to extract features at two spatio-temporal resolutions in an effective and efficient manner. Moreover, our network utilises a cross-head communication strategy to mutually enhance the representations of both heads. We conducted extensive experiments on three large-scale datasets, namely NTU RGB+D 60, NTU RGB+D 120, and Kinetics-Skeleton, and achieves the state-of-the-art performance on all the benchmarks, which validates the effectiveness of our method.
ASMR: Learning Attribute-Based Person Search with Adaptive Semantic Margin Regularizer
发表会议: ICCV 2021
论文地址: https://arxiv.org/abs/2108.04533
摘要
Attribute-based person search is the task of finding person images that are best matched with a set of text attributes given as query. The main challenge of this task is the large modality gap between attributes and images. To reduce the gap, we present a new loss for learning cross-modal embeddings in the context of attribute-based person search. We regard a set of attributes as a category of people sharing the same traits. In a joint embedding space of the two modalities, our loss pulls images close to their person categories for modality alignment. More importantly, it pushes apart a pair of person categories by a margin determined adaptively by their semantic distance, where the distance metric is learned end-to-end so that the loss considers importance of each attribute when relating person categories. Our loss guided by the adaptive semantic margin leads to more discriminative and semantically well-arranged distributions of person images. As a consequence, it enables a simple embedding model to achieve state-of-the-art records on public benchmarks without bells and whistles.
SP-GAN: Sphere-Guided 3D Shape Generation and Manipulation
发表会议: SIGGRAPH 2021
论文地址: https://arxiv.org/abs/2108.04476
摘要
We present SP-GAN, a new unsupervised sphere-guided generative model for direct synthesis of 3D shapes in the form of point clouds. Compared with existing models, SP-GAN is able to synthesize diverse and high-quality shapes with fine details and promote controllability for part-aware shape generation and manipulation, yet trainable without any parts annotations. In SP-GAN, we incorporate a global prior (uniform points on a sphere) to spatially guide the generative process and attach a local prior (a random latent code) to each sphere point to provide local details. The key insight in our design is to disentangle the complex 3D shape generation task into a global shape modeling and a local structure adjustment, to ease the learning process and enhance the shape generation quality. Also, our model forms an implicit dense correspondence between the sphere points and points in every generated shape, enabling various forms of structure-aware shape manipulations such as part editing, part-wise shape interpolation, and multi-shape part composition, etc., beyond the existing generative models. Experimental results, which include both visual and quantitative evaluations, demonstrate that our model is able to synthesize diverse point clouds with fine details and less noise, as compared with the state-of-the-art models.
Reference-based Defect Detection Network
发表期刊: IEEE Transactions on Image Processing
论文地址: https://arxiv.org/abs/2108.04456
摘要
The defect detection task can be regarded as a realistic scenario of object detection in the computer vision field and it is widely used in the industrial field. Directly applying vanilla object detector to defect detection task can achieve promising results, while there still exists challenging issues that have not been solved. The first issue is the texture shift which means a trained defect detector model will be easily affected by unseen texture, and the second issue is partial visual confusion which indicates that a partial defect box is visually similar with a complete box. To tackle these two problems, we propose a Reference-based Defect Detection Network (RDDN). Specifically, we introduce template reference and context reference to against those two problems, respectively. Template reference can reduce the texture shift from image, feature or region levels, and encourage the detectors to focus more on the defective area as a result. We can use either well-aligned template images or the outputs of a pseudo template generator as template references in this work, and they are jointly trained with detectors by the supervision of normal samples. To solve the partial visual confusion issue, we propose to leverage the carried context information of context reference, which is the concentric bigger box of each region proposal, to perform more accurate region classification and regression. Experiments on two defect detection datasets demonstrate the effectiveness of our proposed approach.
SnowflakeNet: Point Cloud Completion by Snowflake Point Deconvolution with Skip-Transformer
发表会议: ICCV 2021
论文地址: https://arxiv.org/abs/2108.04444
摘要
Point cloud completion aims to predict a complete shape in high accuracy from its partial observation. However, previous methods usually suffered from discrete nature of point cloud and unstructured prediction of points in local regions, which makes it hard to reveal fine local geometric details on the complete shape. To resolve this issue, we propose SnowflakeNet with Snowflake Point Deconvolution (SPD) to generate the complete point clouds. The SnowflakeNet models the generation of complete point clouds as the snowflake-like growth of points in 3D space, where the child points are progressively generated by splitting their parent points after each SPD. Our insight of revealing detailed geometry is to introduce skip-transformer in SPD to learn point splitting patterns which can fit local regions the best. Skip-transformer leverages attention mechanism to summarize the splitting patterns used in the previous SPD layer to produce the splitting in the current SPD layer. The locally compact and structured point cloud generated by SPD is able to precisely capture the structure characteristic of 3D shape in local patches, which enables the network to predict highly detailed geometries, such as smooth regions, sharp edges and corners. Our experimental results outperform the state-of-the-art point cloud completion methods under widely used benchmarks.
Domain-Aware Universal Style Transfer
发表会议: ICCV 2021
论文地址: https://arxiv.org/abs/2108.04441
摘要
Style transfer aims to reproduce content images with the styles from reference images. Existing universal style transfer methods successfully deliver arbitrary styles to original images either in an artistic or a photo-realistic way. However, the range of 'arbitrary style' defined by existing works is bounded in the particular domain due to their structural limitation. Specifically, the degrees of content preservation and stylization are established according to a predefined target domain. As a result, both photo-realistic and artistic models have difficulty in performing the desired style transfer for the other domain. To overcome this limitation, we propose a unified architecture, Domain-aware Style Transfer Networks (DSTN) that transfer not only the style but also the property of domain (i.e., domainness) from a given reference image. To this end, we design a novel domainness indicator that captures the domainness value from the texture and structural features of reference images. Moreover, we introduce a unified framework with domain-aware skip connection to adaptively transfer the stroke and palette to the input contents guided by the domainness indicator. Our extensive experiments validate that our model produces better qualitative results and outperforms previous methods in terms of proxy metrics on both artistic and photo-realistic stylizations.
VirtualConductor: Music-driven Conducting Video Generation System
发表会议: ICME 2021
论文地址: https://arxiv.org/abs/2108.04350
摘要
In this demo, we present VirtualConductor, a system that can generate conducting video from any given music and a single user's image. First, a large-scale conductor motion dataset is collected and constructed. Then, we propose Audio Motion Correspondence Network (AMCNet) and adversarial-perceptual learning to learn the cross-modal relationship and generate diverse, plausible, music-synchronized motion. Finally, we combine 3D animation rendering and a pose transfer model to synthesize conducting video from a single given user's image. Therefore, any user can become a virtual conductor through the system.
A Survey of Machine Learning Techniques for Detecting and Diagnosing COVID-19 from Imaging
论文地址: https://arxiv.org/abs/2108.04344
摘要
Due to the limited availability and high cost of the reverse transcription-polymerase chain reaction (RT-PCR) test, many studies have proposed machine learning techniques for detecting COVID-19 from medical imaging. The purpose of this study is to systematically review, assess, and synthesize research articles that have used different machine learning techniques to detect and diagnose COVID-19 from chest X-ray and CT scan images. A structured literature search was conducted in the relevant bibliographic databases to ensure that the survey solely centered on reproducible and high-quality research. We selected papers based on our inclusion criteria. In this survey, we reviewed 98 articles that fulfilled our inclusion criteria. We have surveyed a complete pipeline of chest imaging analysis techniques related to COVID-19, including data collection, pre-processing, feature extraction, classification, and visualization. We have considered CT scans and X-rays as both are widely used to describe the latest developments in medical imaging to detect COVID-19. This survey provides researchers with valuable insights into different machine learning techniques and their performance in the detection and diagnosis of COVID-19 from chest imaging. At the end, the challenges and limitations in detecting COVID-19 using machine learning techniques and the future direction of research are discussed.
Learning to Cut by Watching Movies
发表会议: ICCV 2021
论文地址: https://arxiv.org/abs/2108.04294
摘要
Video content creation keeps growing at an incredible pace; yet, creating engaging stories remains challenging and requires non-trivial video editing expertise. Many video editing components are astonishingly hard to automate primarily due to the lack of raw video materials. This paper focuses on a new task for computational video editing, namely the task of raking cut plausibility. Our key idea is to leverage content that has already been edited to learn fine-grained audiovisual patterns that trigger cuts. To do this, we first collected a data source of more than 10K videos, from which we extract more than 255K cuts. We devise a model that learns to discriminate between real and artificial cuts via contrastive learning. We set up a new task and a set of baselines to benchmark video cut generation. We observe that our proposed model outperforms the baselines by large margins. To demonstrate our model in real-world applications, we conduct human studies in a collection of unedited videos. The results show that our model does a better job at cutting than random and alternative baselines.
TrUMAn: Trope Understanding in Movies and Animations
发表会议: CIKM 2021
论文地址: https://arxiv.org/abs/2108.04542
摘要
Understanding and comprehending video content is crucial for many real-world applications such as search and recommendation systems. While recent progress of deep learning has boosted performance on various tasks using visual cues, deep cognition to reason intentions, motivation, or causality remains challenging. Existing datasets that aim to examine video reasoning capability focus on visual signals such as actions, objects, relations, or could be answered utilizing text bias. Observing this, we propose a novel task, along with a new dataset: Trope Understanding in Movies and Animations (TrUMAn), intending to evaluate and develop learning systems beyond visual signals. Tropes are frequently used storytelling devices for creative works. By coping with the trope understanding task and enabling the deep cognition skills of machines, we are optimistic that data mining applications and algorithms could be taken to the next level. To tackle the challenging TrUMAn dataset, we present a Trope Understanding and Storytelling (TrUSt) with a new Conceptual Storyteller module, which guides the video encoder by performing video storytelling on a latent space. The generated story embedding is then fed into the trope understanding model to provide further signals. Experimental results demonstrate that state-of-the-art learning systems on existing tasks reach only 12.01% of accuracy with raw input signals. Also, even in the oracle case with human-annotated descriptions, BERT contextual embedding achieves at most 28% of accuracy. Our proposed TrUSt boosts the model performance and reaches 13.94% performance. We also provide detailed analysis topave the way for future research. TrUMAn is publicly available at:this https URL
Rethinking Architecture Selection in Differentiable NAS
发表会议: Outstanding Paper Award at ICLR 2021
论文地址: https://arxiv.org/abs/2108.04392
代码地址: https://github.com/ruocwang/darts-pt
摘要
Differentiable Neural Architecture Search is one of the most popular Neural Architecture Search (NAS) methods for its search efficiency and simplicity, accomplished by jointly optimizing the model weight and architecture parameters in a weight-sharing supernet via gradient-based algorithms. At the end of the search phase, the operations with the largest architecture parameters will be selected to form the final architecture, with the implicit assumption that the values of architecture parameters reflect the operation strength. While much has been discussed about the supernet's optimization, the architecture selection process has received little attention. We provide empirical and theoretical analysis to show that the magnitude of architecture parameters does not necessarily indicate how much the operation contributes to the supernet's performance. We propose an alternative perturbation-based architecture selection that directly measures each operation's influence on the supernet. We re-evaluate several differentiable NAS methods with the proposed architecture selection and find that it is able to extract significantly improved architectures from the underlying supernets consistently. Furthermore, we find that several failure modes of DARTS can be greatly alleviated with the proposed selection method, indicating that much of the poor generalization observed in DARTS can be attributed to the failure of magnitude-based architecture selection rather than entirely the optimization of its supernet.
Label-informed Graph Structure Learning for Node Classification
发表会议: CIKM 2021 short paper
论文地址: https://arxiv.org/abs/2108.04595
摘要
Graph Neural Networks (GNNs) have achieved great success among various domains. Nevertheless, most GNN methods are sensitive to the quality of graph structures. To tackle this problem, some studies exploit different graph structure learning strategies to refine the original graph structure. However, these methods only consider feature information while ignoring available label information. In this paper, we propose a novel label-informed graph structure learning framework which incorporates label information explicitly through a class transition matrix. We conduct extensive experiments on seven node classification benchmark datasets and the results show that our method outperforms or matches the state-of-the-art baselines.
A Survey on Deep Reinforcement Learning for Data Processing and Analytics
论文地址: https://arxiv.org/abs/2108.04526
摘要
Data processing and analytics are fundamental and pervasive. Algorithms play a vital role in data processing and analytics where many algorithm designs have incorporated heuristics and general rules from human knowledge and experience to improve their effectiveness. Recently, reinforcement learning, deep reinforcement learning (DRL) in particular, is increasingly explored and exploited in many areas because it can learn better strategies in complicated environments it is interacting with than statically designed algorithms. Motivated by this trend, we provide a comprehensive review of recent works focusing on utilizing deep reinforcement learning to improve data processing and analytics. First, we present an introduction to key concepts, theories, and methods in deep reinforcement learning. Next, we discuss deep reinforcement learning deployment on database systems, facilitating data processing and analytics in various aspects, including data organization, scheduling, tuning, and indexing. Then, we survey the application of deep reinforcement learning in data processing and analytics, ranging from data preparation, natural language interface to healthcare, fintech, etc. Finally, we discuss important open challenges and future research directions of using deep reinforcement learning in data processing and analytics.
AdaRNN: Adaptive Learning and Forecasting of Time Series
发表会议:CIKM 2021
论文地址:https://arxiv.org/abs/2108.04443
代码地址:
https://github.com/jindongwang/transferlearning/tree/master/code/deep/adarnn
摘要
Time series has wide applications in the real world and is known to be difficult to forecast. Since its statistical properties change over time, its distribution also changes temporally, which will cause severe distribution shift problem to existing methods. However, it remains unexplored to model the time series in the distribution perspective. In this paper, we term this as Temporal Covariate Shift (TCS). This paper proposes Adaptive RNNs (AdaRNN) to tackle the TCS problem by building an adaptive model that generalizes well on the unseen test data. AdaRNN is sequentially composed of two novel algorithms. First, we propose Temporal Distribution Characterization to better characterize the distribution information in the TS. Second, we propose Temporal Distribution Matching to reduce the distribution mismatch in TS to learn the adaptive TS model. AdaRNN is a general framework with flexible distribution distances integrated. Experiments on human activity recognition, air quality prediction, and financial analysis show that AdaRNN outperforms the latest methods by a classification accuracy of 2.6% and significantly reduces the RMSE by 9.0%. We also show that the temporal distribution matching algorithm can be extended in Transformer structure to boost its performance.
Continual Learning for Grounded Instruction Generation by Observing Human Following Behavior
发表期刊: TACL
论文地址: https://arxiv.org/abs/2108.04812
摘要
We study continual learning for natural language instruction generation, by observing human users' instruction execution. We focus on a collaborative scenario, where the system both acts and delegates tasks to human users using natural language. We compare user execution of generated instructions to the original system intent as an indication to the system's success communicating its intent. We show how to use this signal to improve the system's ability to generate instructions via contextual bandit learning. In interaction with real users, our system demonstrates dramatic improvements in its ability to generate language over time.
Multi-Factors Aware Dual-Attentional Knowledge Tracing
发表会议: CIKM 2021
论文地址: https://arxiv.org/abs/2108.04741
摘要
With the increasing demands of personalized learning, knowledge tracing has become important which traces students' knowledge states based on their historical practices. Factor analysis methods mainly use two kinds of factors which are separately related to students and questions to model students' knowledge states. These methods use the total number of attempts of students to model students' learning progress and hardly highlight the impact of the most recent relevant practices. Besides, current factor analysis methods ignore rich information contained in questions. In this paper, we propose Multi-Factors Aware Dual-Attentional model (MF-DAKT) which enriches question representations and utilizes multiple factors to model students' learning progress based on a dual-attentional mechanism. More specifically, we propose a novel student-related factor which records the most recent attempts on relevant concepts of students to highlight the impact of recent exercises. To enrich questions representations, we use a pre-training method to incorporate two kinds of question information including questions' relation and difficulty level. We also add a regularization term about questions' difficulty level to restrict pre-trained question representations to fine-tuning during the process of predicting students' performance. Moreover, we apply a dual-attentional mechanism to differentiate contributions of factors and factor interactions to final prediction in different practice records. At last, we conduct experiments on several real-world datasets and results show that MF-DAKT can outperform existing knowledge tracing methods. We also conduct several studies to validate the effects of each component of MF-DAKT.
Hierarchical Latent Relation Modeling for Collaborative Metric Learning
发表会议: ACM RecSys 2021
论文地址: https://arxiv.org/abs/2108.04655
摘要
Collaborative Metric Learning (CML) recently emerged as a powerful paradigm for recommendation based on implicit feedback collaborative filtering. However, standard CML methods learn fixed user and item representations, which fails to capture the complex interests of users. Existing extensions of CML also either ignore the heterogeneity of user-item relations, i.e. that a user can simultaneously like very different items, or the latent item-item relations, i.e. that a user's preference for an item depends, not only on its intrinsic characteristics, but also on items they previously interacted with. In this paper, we present a hierarchical CML model that jointly captures latent user-item and item-item relations from implicit data. Our approach is inspired by translation mechanisms from knowledge graph embedding and leverages memory-based attention networks. We empirically show the relevance of this joint relational modeling, by outperforming existing CML models on recommendation tasks on several real-world datasets. Our experiments also emphasize the limits of current CML relational models on very sparse datasets.
Learning Multi-Granular Spatio-Temporal Graph Network for Skeleton-based Action Recognition
发表会议: ACM MM 2021
论文地址: https://arxiv.org/abs/2108.04536
摘要
The task of skeleton-based action recognition remains a core challenge in human-centred scene understanding due to the multiple granularities and large variation in human motion. Existing approaches typically employ a single neural representation for different motion patterns, which has difficulty in capturing fine-grained action classes given limited training data. To address the aforementioned problems, we propose a novel multi-granular spatio-temporal graph network for skeleton-based action classification that jointly models the coarse- and fine-grained skeleton motion patterns. To this end, we develop a dual-head graph network consisting of two interleaved branches, which enables us to extract features at two spatio-temporal resolutions in an effective and efficient manner. Moreover, our network utilises a cross-head communication strategy to mutually enhance the representations of both heads. We conducted extensive experiments on three large-scale datasets, namely NTU RGB+D 60, NTU RGB+D 120, and Kinetics-Skeleton, and achieves the state-of-the-art performance on all the benchmarks, which validates the effectiveness of our method.
Enhancing Knowledge Tracing via Adversarial Training
发表会议: ACM MM 2021
论文地址: https://arxiv.org/abs/2108.04430
摘要
We study the problem of knowledge tracing (KT) where the goal is to trace the students' knowledge mastery over time so as to make predictions on their future performance. Owing to the good representation capacity of deep neural networks (DNNs), recent advances on KT have increasingly concentrated on exploring DNNs to improve the performance of KT. However, we empirically reveal that the DNNs based KT models may run the risk of overfitting, especially on small datasets, leading to limited generalization. In this paper, by leveraging the current advances in adversarial training (AT), we propose an efficient AT based KT method (ATKT) to enhance KT model's generalization and thus push the limit of KT. Specifically, we first construct adversarial perturbations and add them on the original interaction embeddings as adversarial examples. The original and adversarial examples are further used to jointly train the KT model, forcing it is not only to be robust to the adversarial examples, but also to enhance the generalization over the original ones. To better implement AT, we then present an efficient attentive-LSTM model as KT backbone, where the key is a proposed knowledge hidden state attention module that adaptively aggregates information from previous knowledge hidden states while simultaneously highlighting the importance of current knowledge hidden state to make a more accurate prediction. Extensive experiments on four public benchmark datasets demonstrate that our ATKT achieves new state-of-the-art performance. Code is available at: \color{blue} {\url{this https URL}}.
How Commonsense Knowledge Helps with Natural Language Tasks: A Survey of Recent Resources and Methodologies
论文地址: https://arxiv.org/abs/2108.04674
摘要
In this paper, we give an overview of commonsense reasoning in natural language processing, which requires a deeper understanding of the contexts and usually involves inference over implicit external knowledge. We first review some popular commonsense knowledge bases and commonsense reasoning benchmarks, but give more emphasis on the methodologies, including recent approaches that aim at solving some general natural language problems that take advantage of external knowledge bases. Finally, we discuss some future directions in pushing the boundary of commonsense reasoning in natural language processing.
FairyTailor: A Multimodal Generative Framework for Storytelling
论文地址: https://arxiv.org/abs/2108.04324
项目地址: https://github.com/EdenBD/MultiModalStory-demo
演示地址: https://fairytailor.org/
摘要
Storytelling is an open-ended task that entails creative thinking and requires a constant flow of ideas. Natural language generation (NLG) for storytelling is especially challenging because it requires the generated text to follow an overall theme while remaining creative and diverse to engage the reader. In this work, we introduce a system and a web-based demo, FairyTailor, for human-in-the-loop visual story co-creation. Users can create a cohesive children's fairytale by weaving generated texts and retrieved images with their input. FairyTailor adds another modality and modifies the text generation process to produce a coherent and creative sequence of text and images. To our knowledge, this is the first dynamic tool for multimodal story generation that allows interactive co-formation of both texts and images. It allows users to give feedback on co-created stories and share their results.
·